Imagine a world where technology adapts to our needs autonomously. Sounds like science fiction, right? But, it’s closer than you think. Advances in artificial intelligence are creating systems that can learn, reason, and make decisions with little human intervention. Yet, these systems must possess’ agentic’ qualities to become truly autonomous and less reliant on human oversight. Understanding the multi agent AI can help you leverage the best LLMs to create brighter, more autonomous systems that drive innovation and efficiency in your AI applications. This article will provide valuable insights to help you achieve your objectives. Lamatic's generative AI tech stack can help you achieve your goals. Our tools and resources can help you understand how the best agentic definition in AI leads to more intelligent, more autonomous systems that drive innovation and efficiency in their AI applications.
What Is the Best Agentic Definition in AI, and How Does It Work?
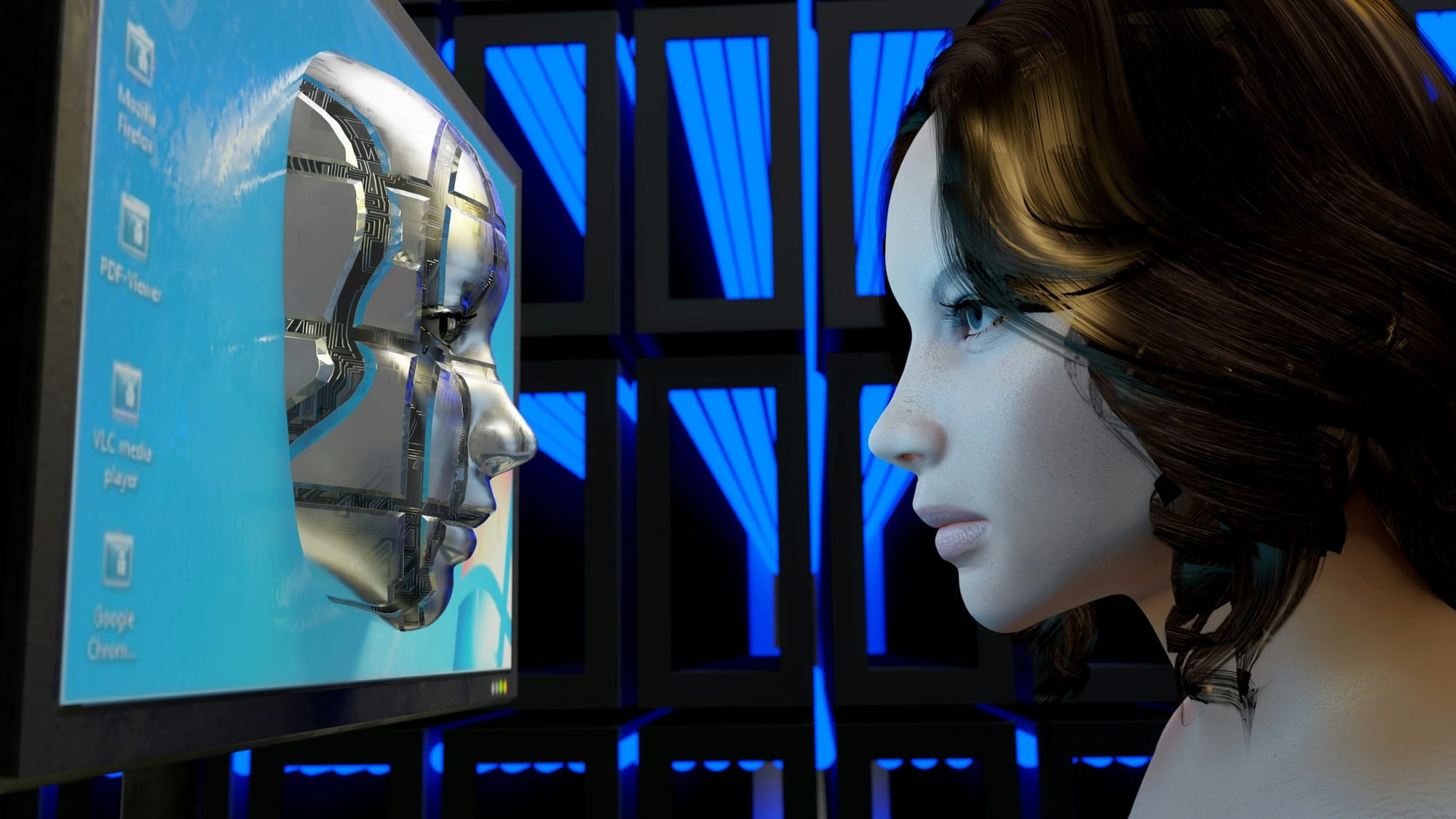
Agentic AI is a term that describes a new breed of artificial intelligence systems and models that can act autonomously to achieve goals without the need for constant human guidance. The agentic AI system understands the user’s goal or vision and the context of the problem they are trying to solve.
“You can define agentic AI with one word: proactiveness,” said Enver Cetin, an AI expert at global Experience Engineering firm Ciklum. “These systems don’t wait for humans to tell them what to do. They are empowered to make decisions independently and take action to optimize outcomes.”
How Agentic AI Works
Agentic AI uses a four-step process for problem-solving.
Here’s how it works:
1. Perceive
AI agents gather and process data from various sources, such as:
- Sensors
- Databases
- Digital interfaces
This involves extracting meaningful features, recognizing objects, or identifying relevant environmental entities.
Reason
A large language model acts as the orchestrator, or reasoning engine, that understands tasks, generates solutions, and coordinates specialized models for specific functions like:
- Content creation
- Visual processing
- Recommendation systems
This step uses retrieval-augmented generation to access proprietary data sources and deliver accurate, relevant outputs.
Act
By integrating with external tools and software via application programming interfaces, agentic AI can quickly execute tasks based on the plans it has formulated. Guardrails can be built into AI agents to help ensure they execute tasks correctly.
For example, a customer service AI agent may process claims up to a certain amount, while claims above the amount would have to be approved by a human.
Learn
Agentic AI continuously improves through a feedback loop, or “data flywheel,” where the data generated from interactions is fed into the system to enhance models.
This ability to adapt and become more effective over time offers businesses a powerful tool for driving better decision-making and operational efficiency.
The Benefits of Working with Agentic AI
With their supercharged reasoning and execution capabilities, agentic AI systems promise to transform many aspects of human-machine collaboration, especially in areas of work that were previously insulated from AI-led automation, such as:
- Proactively managing complex IT systems to pre-empt outages
- Dynamically re-configuring supply chains in response to geopolitical or weather disruptions
- Engaging in realistic interactions with patients or customers to resolve issues
Three of the main benefits will be:
Greater Specialization
The value of workforce specialization, famously illustrated by Adam Smith’s pin factory example in The Wealth of Nations, lies in its ability to:
- Drive efficiency
- Foster skill development
- Spur innovation
Yet, realizing this in modern businesses is often hindered by talent shortages and skill mismatches.
Agentic AI models offer a transformative solution: designed for particular tasks, they enable deeper role specialization than traditional automation. These agents can be deployed rapidly across functions, working both independently and in coordination, such as:
- Data retrieval
- Analysis
- Workflow creation
- Employee support
Innovation
With advanced judgment and execution capabilities, agentic AI systems are well-suited for driving experimentation and innovation. Tools like ChemCrow, a chemistry-focused AI agent, have been used to plan and synthesize new insect repellents and novel organic compounds. Multi-agent models further accelerate discovery by rapidly scanning and analyzing extensive research domains.
For instance, MIT’s SciAgents system includes AI agents that design research plans and a Critic Agent that reviews and refines them. This collaborative agentic approach led to the discovery of a new biomaterial combining silk and dandelion pigments, offering improved mechanical and optical properties with lower energy requirements.
Greater Trustworthiness
With their advanced cognitive reasoning, Agentic AI systems are less prone to hallucinations, or fabricated outputs that commonly affect generative AI. Their ability to assess and differentiate information sources based on quality and reliability enhances trust in their decisions.
For instance, when processing fragmented customer data across emails, spreadsheets, and databases, an agentic system can identify the firm’s CRM platform as the most reliable source. These systems are designed to rapidly learn and align with an organization’s human and brand values, ensuring that actions and decisions reflect the company’s principles.
Potential Use Cases
While many applications of agentic AI are still experimental or at a pilot stage, the broad contours of potential use cases are already emerging across different industries and functions.
Some examples include:
Customer Service
Unlike traditional automated bots with fixed responses, agentic customer service agents can understand customer intent and emotion and take independent action to resolve issues. For instance, an agent might anticipate a delayed delivery, notify the customer, and proactively offer compensation.
Companies like Ema, a California-based AI startup, are pioneering such solutions with chatbots capable of navigating thousands of databases and applications to address customer needs. These agents learn from each interaction, assist human agents with recommended actions, and ensure accuracy, compliance, and continuous improvement of the customer knowledge base.
Manufacturing
From controlling the flow of production lines to customizing products to making suggestions for improved product design, agentic AI will likely have multiple applications in smart manufacturing. An agentic AI system can analyze data from sensors attached to machines, components, and other physical assets in factories and transportation to predict wear-and-tear and production outages, avoiding unscheduled downtime and associated costs to manufacturers.
German AI start-up Juna.ai deploys AI agents to run virtual factories. It maximizes productivity and quality while reducing energy consumption and carbon emissions. It even offers agents tailored to specific goals, such as production and quality agents.
Sales Support
Sales professionals often struggle to focus on lead generation amid the burden of emails, paperwork, and routine admin. Agentic AI systems offer a transformative solution by automating these time-consuming tasks.
Salesforce, a leader in CRM technology, has introduced its Agent Force Service Development Rep, an AI-powered assistant that interprets:
- Customer messages
- Suggests next steps
- Schedules meetings
- Answers queries
- Crafts brand-aligned responses
The Agent Force Sales Coach supports this by delivering personalized feedback and virtual role-play training to enhance the performance and development of human sales agents.
Health and Social Care
With the ability to adapt, interpret human emotions, and demonstrate empathy, agentic AI systems are well-suited for non-routine, soft-skill roles in healthcare and caregiving. California-based Hippocratic AI has developed a suite of specialized agents to support various healthcare and social services functions.
Among them is Sarah, an empathetic AI agent designed for assisted living, who engages patients in conversation, coordinates meals and transportation, and provides medication reminders. Another agent, Judy, supports pre-operative care by offering timely reminders and guidance on arrival times, fasting, and medication protocols.
Related Reading
- What is Agentic AI
- How to Integrate AI Into an App
- Generative AI Tech Stack
- Application Integration Framework
- Mobile App Development Frameworks
- How to Build an AI app
- How to Build an AI Agent
- Crewai vs Autogen
- Types of AI Agents
What’s the Difference Between AI Agents and Agentic AI?
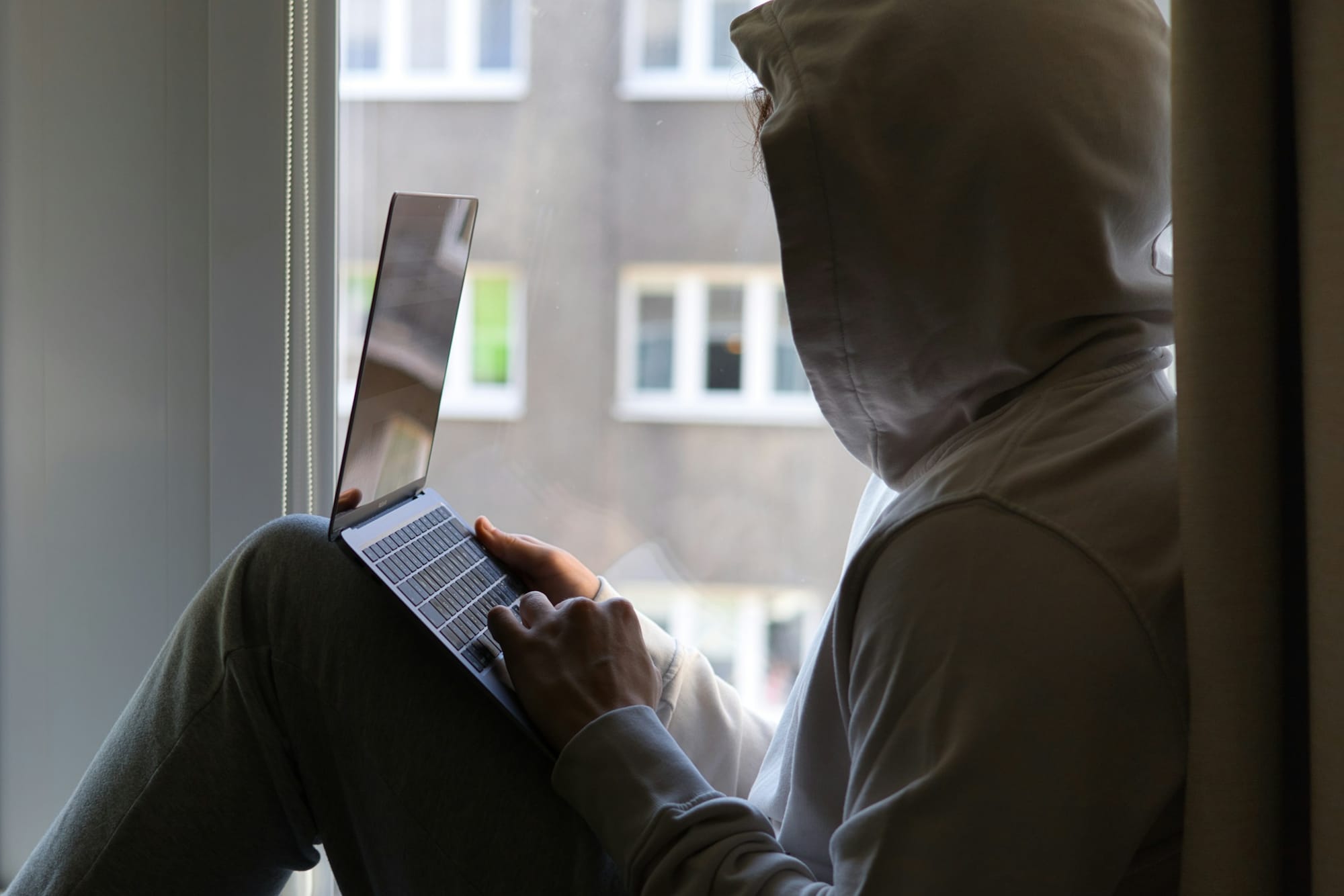
Agentic AI focuses on autonomous systems capable of independent decision-making and actions. At its core, Agentic AI is an AI that’s all about autonomy.
This means that it can:
- Make decisions
- Take actions
- Learn on its own to achieve specific goals
It’s like having a virtual assistant who can think, reason, and adapt to changing circumstances without constant direction.
What is an AI Agent?
AI agents are programs designed to perform specific tasks or address defined problems, often automating simple processes. They lack the autonomy and decision-making capabilities of agentic AI. For example, a chatbot that answers customer queries functions as an AI agent: it responds based on data patterns but doesn’t truly understand the context.
While helpful, AI agents cannot think or adapt independently. When faced with unexpected questions, they require human intervention to either provide a response or reprogram the system to handle similar queries in the future.
What’s the Difference?
Although AI agents and agentic AI are powered by artificial intelligence, they operate in distinct ways with different focuses. Generative AI (GenAI) excels in creating content across various formats such as text, images, and music, driven by human input to define context and goals.
It’s ideal for:
- Brainstorming
- Idea generation
- Solution development
Agentic AI is action-oriented, designed to autonomously make decisions, formulate strategies, and execute tasks to achieve specific goals with minimal human involvement. While GenAI generates new content, agentic AI produces actions and decisions. These two technologies can work together, with GenAI creating content (e.g., marketing copy) and agentic AI autonomously deploying it across the proper channels based on real-time data and objectives.
Where Do We See These in the Real World?
Both agentic AI and AI agents have started popping up in various industries, and their applications are growing fast.
Agentic AI in Action
Some of the most exciting real-world applications of agentic AI include:
Self-Driving Cars
One of the most exciting uses of agentic AI is in autonomous vehicles. These AI systems perceive:
- Their surroundings
- Make driving decisions
- Learn from every trip
Over time, they get better at navigating and handling new challenges on the road.
For example, Tesla’s Full Self-Driving system is an example of agentic AI that continuously learns from the driving environment and adjusts its behavior to improve safety and efficiency.
Supply Chain Management
Agentic AI is also helping companies optimize their supply chains. AI can ensure smoother, more efficient operations by autonomously managing inventory, predicting demand, and adjusting delivery routes in real time.
Amazon’s warehouse robots, powered by AI, are an example. These robots navigate complex environments, adapt to conditions, and move goods around warehouses autonomously.
Cybersecurity
In cybersecurity, agentic AI can detect threats and vulnerabilities by analyzing network activity and automatically responding to potential breaches. Darktrace, an AI cybersecurity company, uses agentic AI to autonomously detect, respond to, and learn from potential cyber threats in real time.
Healthcare
AI is playing a significant role in healthcare, too. Agentic AI can assist with:
- Diagnostics
- Treatment recommendations
- Patient care management
It analyzes medical data, identifies patterns, and helps doctors make more informed decisions. For instance, IBM’s Watson Health uses AI to analyze massive amounts of healthcare data, learning from new information to offer insights that help doctors and healthcare professionals.
AI Agents in Action
In contrast, some typical applications of AI agents include:
Customer Support
One of the most common uses of AI agents is in customer service. Chatbots can answer questions, resolve issues, and guide customers through processes without human intervention.
Zendesk’s AI-powered chatbot helps businesses respond to customer queries quickly and efficiently. It acts as an AI agent that handles common issues and frees up human agents for more complex tasks.
Personal Assistants
You already interact with an AI agent daily if you use voice assistants like Siri or Google Assistant. They can help you:
- Set reminders
- Check the weather
- Play your favorite music
These functional tasks don’t require much decision-making. These AI agents rely on predefined commands and are great at handling simple, repetitive tasks.
Email Management
AI agents are also great for managing your inbox. They can sort emails, flag important ones, and even provide smart replies to save time. Google’s Gmail Smart Compose feature is an excellent example of an AI agent at work, helping users respond to emails faster by suggesting phrases based on context.
Productivity Tools
Tools like GitHub Copilot are AI agents that help software developers by suggesting code and helping with debugging. They’re like having a second set of eyes always there to help.
This AI agent enhances developer productivity by offering real-time code suggestions, allowing them to focus on more creative aspects of their work.
Related Reading
- Llamaindex vs Langchain
- LLM Agents
- LangChain vs LangSmith
- Langsmith Alternatives
- LangChain vs RAG
- Crewai vs Langchain
- AutoGPT vs AutoGen
- GPT vs LLM
- AI Development Tools
- Rapid Application Development Tools
Start Building GenAI Apps for Free Today with Our Managed Generative AI Tech Stack
What is Lamatic’s Generative AI Solution?
Lamatic offers a comprehensive Generative AI tech stack that helps businesses integrate AI into their operations. Their managed Generative AI solution includes a:
- Custom GenAI API
- Low-code agent builder
- Automated workflow
- GenOps, edge deployment
- Integrated vector database
This solution allows teams to implement AI solutions quickly, reducing tech debt and automating workflows to ensure production-grade deployment.
What is Managed GenAI Middleware?
Managed GenAI middleware automates the integration of Generative AI technology into existing operations. It provides a layer between the technology and a business’s applications to ease communication and reduce the complexity of implementing AI solutions.
This approach allows organizations to focus on business operations and workflows instead of getting bogged down with the technical details of implementing AI. Lamatic’s managed middleware solution accelerates the integration of AI and helps teams avoid costly tech debt associated with building custom solutions from scratch.
What is Lamatic’s Custom GenAI API?
Lamatic’s custom Generative AI API is built on GraphQL, an open-source data query and manipulation language. This flexible GenAI API allows businesses to access the data they need for GenAI applications quickly and efficiently.
The API provides a more efficient way to query and retrieve data than traditional REST APIs, reducing the time it takes to build production-grade Generative AI applications. With Lamatic’s custom GenAI API, teams can easily pull the critical data they need to ensure GenAI applications perform accurately and efficiently.
What is a Low-Code Agent Builder?
Lamatic’s low-code agent builder provides a visual interface for creating intelligent agents powered by Generative AI. Businesses can use the tool to build custom AI applications without extensive technical knowledge or programming skills.
The low-code interface allows users to build GenAI applications with prebuilt templates and modules that speed up development time. This approach makes it easier for organizations to develop custom solutions that meet their business needs.
Related Reading
- Best No Code App Builders
- LLM vs Generative AI
- Langchain Alternatives
- Autogen vs Langchain
- Langflow vs Flowise
- SLM vs LLM
- Langgraph vs Langchain
- Haystack vs Langchain
- Semantic Kernel vs Langchain
- UiPath Competitors
- AI Developers
- Best AI Models
- Best AI Coding Assistant
- LangChain Agent
- Best AI Code Generator
- AI Developer Tools