Building a generative AI solution to enhance your product's capabilities is a challenging task. There's the overwhelming task of identifying the right tools and technology. Then, you have actually to learn how to use those tools effectively. If you're considering Amazon SageMaker, that's a solid choice. The platform includes many valuable features to help you start your generative AI project. However, it can be expensive, especially if you need to scale your operations quickly. Furthermore, there may be better options to meet your specific needs. In this article, we'll explore the best SageMaker alternatives to help you find the right fit for your generative AI project.
One of the best resources for finding the right alternative to AWS SageMaker is Lamatic's generative AI tech stack. This solution provides many features to help you identify the right tools for your project so you can build your solution faster, cheaper, and with fewer headaches.
What is AWS SageMaker & Its Key Capabilities
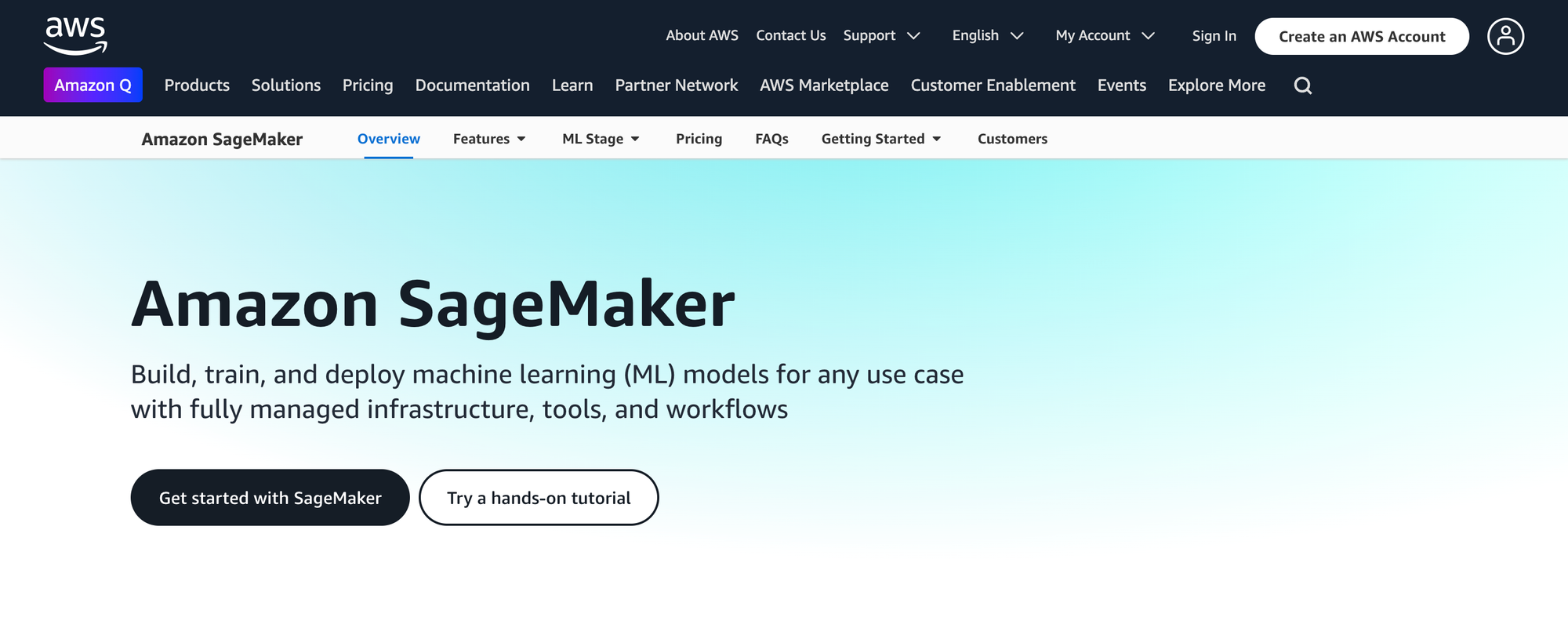
AWS SageMaker is a comprehensive, fully managed service offered by Amazon Web Services. It facilitates the entire machine learning (ML) workflow, making it simpler for developers and data scientists to build, train, and deploy machine learning models quickly and efficiently.
Sagemaker integrates various components of ML projects into a single, user-friendly environment, including:
- Data preparation
- Model building
- Training
- Deployment
Comprehensive Machine Learning Platform and AWS Integration
SageMaker's standout features include a broad selection of built-in algorithms, one-click deployment, automatic model tuning, and the ability to scale jobs of any size with fully managed infrastructure. Additionally, SageMaker offers robust integration with the AWS ecosystem, allowing seamless access to data storage, analytics, and other AWS services.
Pricing
Amazon Sagemaker uses the pay-as-you-go model. It allows users to pay for only what they use, with no upfront payments or long-term contracts required.
Is AWS SageMaker Right for Me?
Sagemaker could be the ideal choice for you if you are:
- An organization that leverages AWS services and benefits from native integration for a smoother workflow.
- An ML practitioner who values the ability to scale jobs easily and manage models comprehensively within a robust ecosystem.
- Mindful of the potential costs associated with a pay-as-you-go pricing model and prepared to manage these expenses as your project scales.
- Willing to navigate the complexity of SageMaker’s extensive features to take full advantage of its capabilities.
Reasons for Exploring Alternatives to Sagemaker
Compute Cost
SageMaker imposes compute costs on teams in two main ways. First, SageMaker instances are marked up, often significantly, compared to the equivalent underlying EC2 instance on which they run. This might be an acceptable cost for teams at the early stages of ML, who favor flexibility and total freedom from infrastructure management. But as teams develop and run more and heavier workloads, these start to add up.
SageMaker does not offer all available instance types in the choice of compute compared to the full EC2 or EKS catalog. This leads to the “wrong-sizing” of boxes to execute tasks.
Cloud Cost Optimization and Multi-Cloud Strategies
Teams might even want to go across clusters and clouds to access computing elsewhere in a more advanced setting, especially if GPU computing is more accessible or cheaper in other clouds. Combining and matching clouds within a single pipeline can significantly affect large cloud bills.
Complexity and Learning Curve
SageMaker is a set of tools that Amazon has built, and learning how to use each and then how to use them together is a significant lift. Data scientists who did not previously use the platform cannot be reasonably expected to know how to use it the way they might know how to use Python generically. And, given the complexity, once you enter the platform, it can be hard to depart again.
Workflows are not rendered in regular Python code or portable formats but in particular AWS-managed services. Lock-in is reasonable for vendors, not teams.
Flexibility
SageMaker makes things “easy,” but as is often the trade-off with software that has been made “easy,” it is also harder to debug, adjust, and customize. This means that you are bound to their opinionated views on what workflows should look like, what logging and tracking should be, etc.
Rather than providing the infinite flexibility of code or fine-grained control that managing your infrastructure brings, you are taking the benefits of all-in-one with the costs to flexibility as well.
Related Reading
- How to Build AI
- Gen AI vs AI
- GenAI Applications
- Generative AI Customer Experience
- Generative AI Automation
- Generative AI Risks
- AI Product Development
- GenAI Tools
- Enterprise Generative AI Tools
- Generative AI Development Services
20 Best AWS SageMaker Alternatives for Next-Gen GenAI Solutions
1. Lamatic: The All-in-One Solution for GenAI Applications
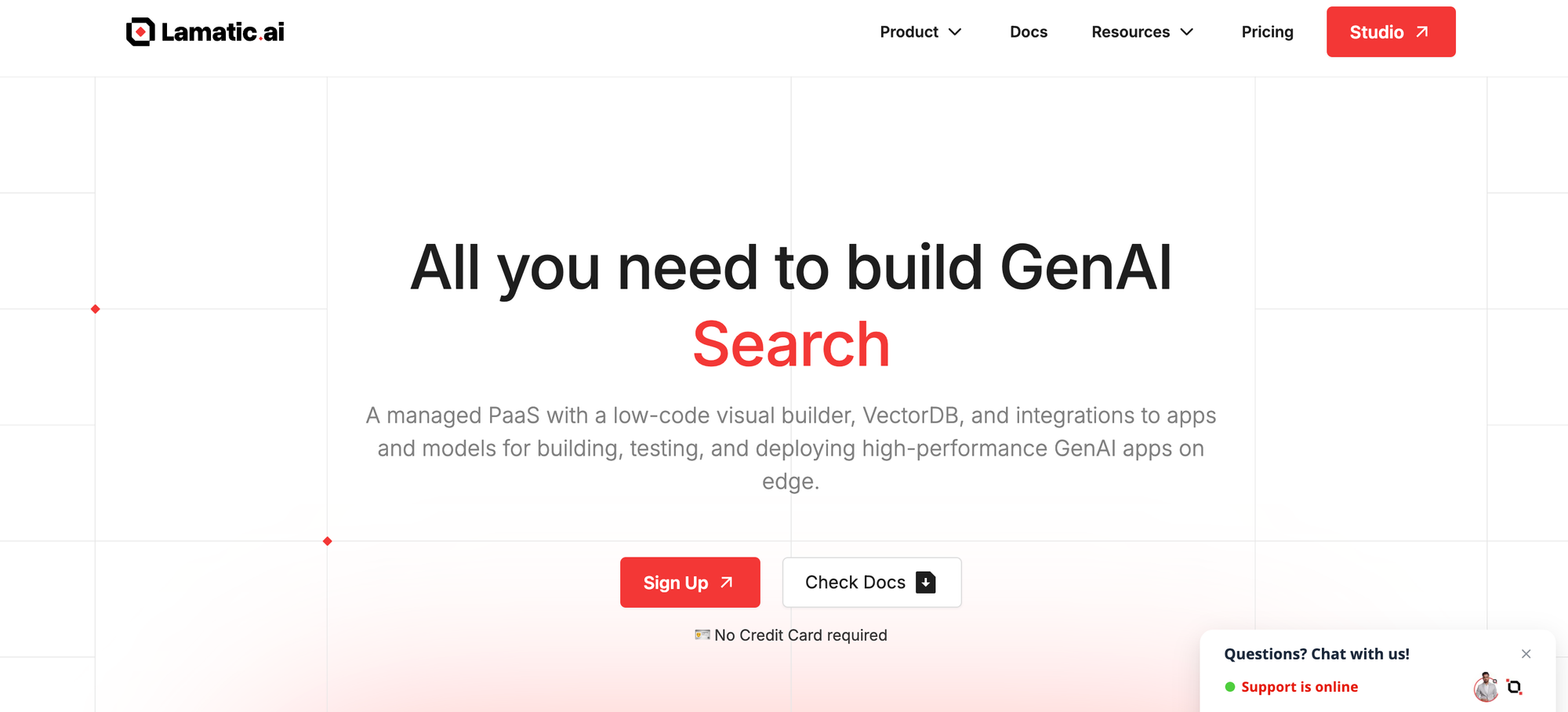
Lamatic offers a managed Generative AI tech stack that includes:
- Managed GenAI Middleware
- Custom GenAI API (GraphQL)
- Low-Code Agent Builder
- Automated GenAI Workflow (CI/CD)
- GenOps (DevOps for GenAI)
- Edge Deployment via Cloudflare Workers
- Integrated Vector Database (Weaviate)
Lamatic empowers teams to rapidly implement GenAI solutions without accruing tech debt. Our platform automates workflows and ensures production-grade deployment on the edge, enabling fast, efficient GenAI integration for products needing swift AI capabilities.
Key Offerings
- Intuitive Managed Backend: Facilitates drag-and-drop connectivity to your preferred models, data sources, tools, and applications.
- Collaborative Development Environment: This environment enables teams to build, test, and refine sophisticated GenAI workflows collaboratively in a unified space.
- Seamless Prototype to Production Pipeline: Features weaviate and self-documenting GraphQL API that automatically scales on Serverless Edge infrastructure
- Contextual App Development: Lamatic.ai offers a powerful, fully managed GenAI stack that includes Weaviate. This innovative platform allows users to harness advanced AI technologies without requiring deep technical expertise.
Let's explore how to build a simple contextual chatbot using Lamatic.ai, following three key phases:
- Build
- Connect
- Deploy
The low-code approach offered by Lamatic.ai transforms complex technical concepts such as:
- Vector databases
- Text chunking
- Model selection into intuitive, approachable processes
This simplification breaks down barriers that have traditionally deterred many from exploring AI applications. Lamatic's extensive library of templates and pre-built configurations significantly accelerates the development process. This allows businesses to rapidly prototype, iterate, and scale their AI solutions without getting bogged down in technical intricacies.
Lamatic.ai empowers organizations to focus on innovation and problem-solving rather than wrestling with infrastructure and code by lowering the entry barrier and speeding up development cycles.
Start building GenAI apps for free today with our managed generative AI tech stack.
2. TrueFoundry: A Secure and Efficient MLOps Platform
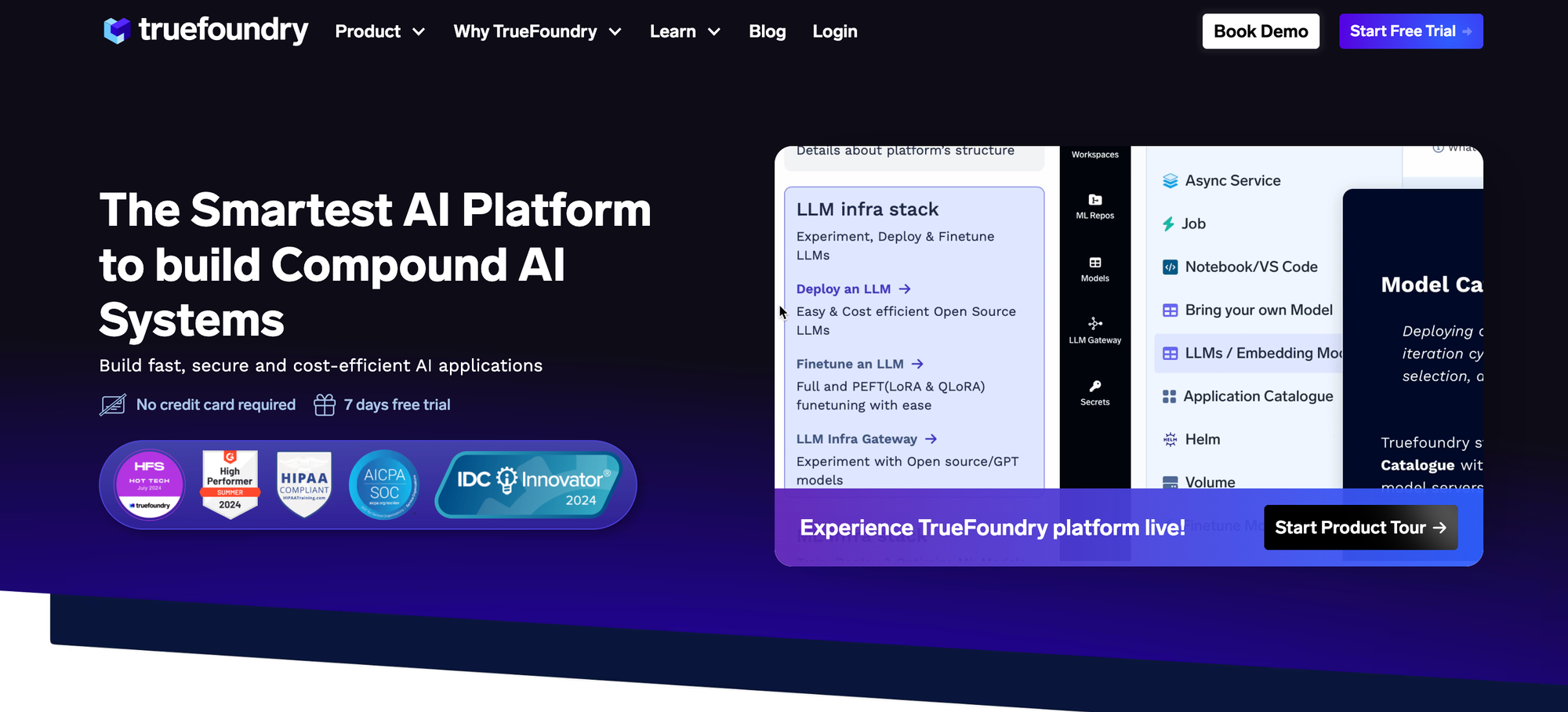
TrueFoundry is designed to significantly ease the deployment of applications on Kubernetes clusters within your own cloud provider account. It emphasizes data security through the following features:
- Data and Compute Operations: Ensures all operations remain within your environment.
- SRE Principles: Adheres to Site Reliability Engineering principles for enhanced reliability.
- Cloud-Native Architecture: Enables efficient use of hardware from various cloud providers.
Its architecture provides a split plane comprising a Control Plane for orchestration and a Compute Plane where user code runs. This architecture aims to secure, efficient, and cost-effective ML operations.
Streamlining the ML Workflow
TrueFoundry excels in offering an environment that streamlines the development to deployment pipeline, thanks to its integration with popular ML frameworks and tools. This allows for a more fluid workflow, easing the transition from model training to actual deployment. It provides engineers and data developers with an interface that prioritizes human-centric design, significantly reducing the overhead typically associated with ML operations.
With 24/7 support and guaranteed service level agreements (SLAs), TrueFoundry assures a solid foundation for data teams to innovate without reinventing infrastructure solutions.
Limitations: TrueFoundry's extensive feature set and integration capabilities may introduce complexity, leading to a steep learning curve for new users.
3. BentoML: A Smooth-Operating Open-Source Framework
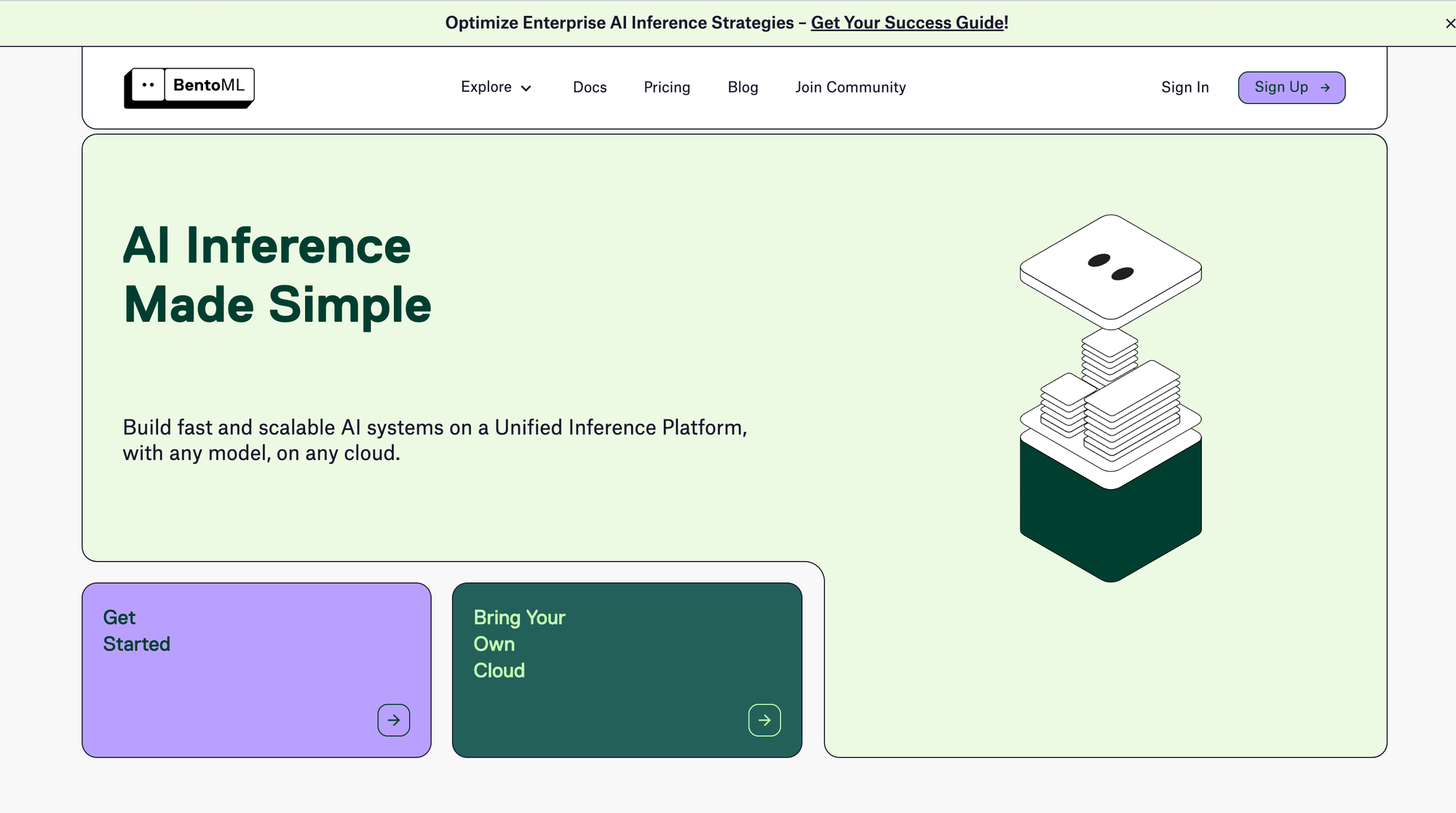
BentoML is an open-source platform designed for serving, managing, and deploying machine learning models easily and at scale.As part of AI application packaging, BentoML is a container for all the components in a model service, packaging applications and deployment in a streamlined way. The power of openness lies in its standard and SDK, through which developers can build applications using any model sourced from third-party hubs or developed in-house with popular frameworks like:
- PyTorch
- TensorFlow
BentoML also maximizes optimization and efficiency by integrating with high-performance runtimes, such as reduced response time and support for parallel processing, improved throughput, and adaptive batching for better resource efficiency. Not only that, but BentoML also ensures simple architecture because of the development that is Python-first and its tight integration with popular ML platforms like:
- MLFlow
- Kubeflow
BentoML makes the deployment process simple: single-click deployments to BentoCloud or large-scale deployments with Yatai on Kubernetes. BentoML supports deploying anywhere Docker is supported.
Limitations: We consider other options for BentoML mainly because it focuses on production workloads and offers limited support for the preceding steps in the machine learning development life cycle, such as experimentation and model refinement.
While BentoML excels at serving models in production through its own API and command-line tools for model registry and deployment, it requires manual integration for additional features beyond production, like model storage.
4. Vertex AI: Google Cloud's ML Powerhouse
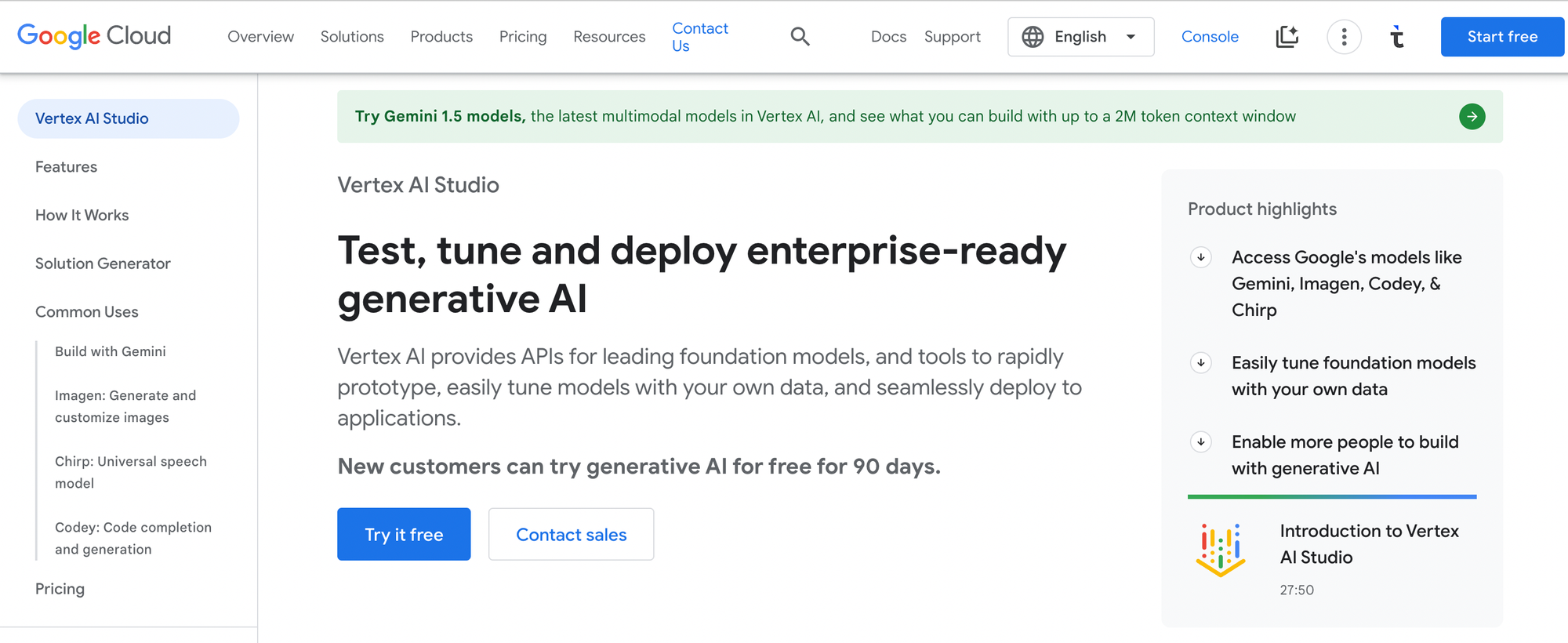
Vertex AI is Google Cloud's unified machine learning platform that streamlines the development of AI models and applications. It offers a cohesive environment for the entire machine-learning workflow, including:
- Training
- Fine-tuning
Deployment of Machine Learning Models
Vertex AI stands out for its ability to support over 100 foundation models and integration with services for conversational AI and other solutions. It accelerates the ML development process, allowing for rapid training and deployment of models on the same platform, which is beneficial for both efficiency and consistency in ML projects.
Limitations: Despite its extensive features and integration capabilities, Vertex AI can present challenges when transitioning existing code and workflows into its environment. Users may need to adapt to Vertex AI's operational methods, which could lead to a degree of vendor lock-in.
Cost Considerations for Large-Scale Deployments
Large-scale deployments could increase expenses, especially when utilizing high-resource services such as AutoML and large-language model training. These potential cost implications and operational adjustments are critical factors when choosing Vertex AI as a machine learning platform.
5. Seldon Core: An Open-Source Solution for ML Model Deployment
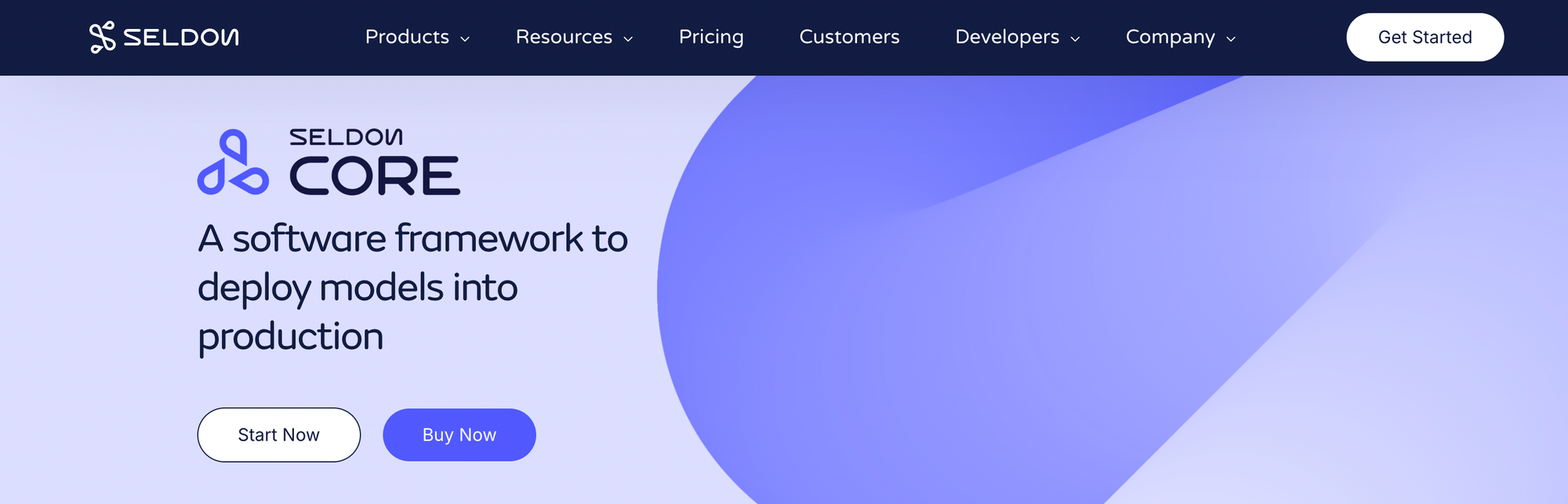
Seldon Core is an open-source platform designed to simplify the deployment, scaling, and management of machine learning models on Kubernetes. It provides a powerful framework for serving models built with any machine learning toolkit, enabling easy wrapping of models into Docker containers ready for deployment.
Seldon Core facilitates complex inference pipelines, A/B testing, canary rollouts, and comprehensive monitoring with Prometheus, ensuring high efficiency and scalability for machine learning operations.
Limitations: The initial setup requires a good understanding of Kubernetes, which may present a steep learning curve for those unfamiliar with container orchestration. Also, while it supports a wide range of ML tools and languages, customization or use of non-standard frameworks can complicate the workflow.
Certain servers, like MLServer or Triton Server, do not support some advanced features, like data preprocessing and postprocessing. Although extensive, the documentation may be lacking for advanced use cases and occasionally leads to deprecated or unavailable content.
6. MLflow: An Open-Source Tool for Managing the ML Lifecycle
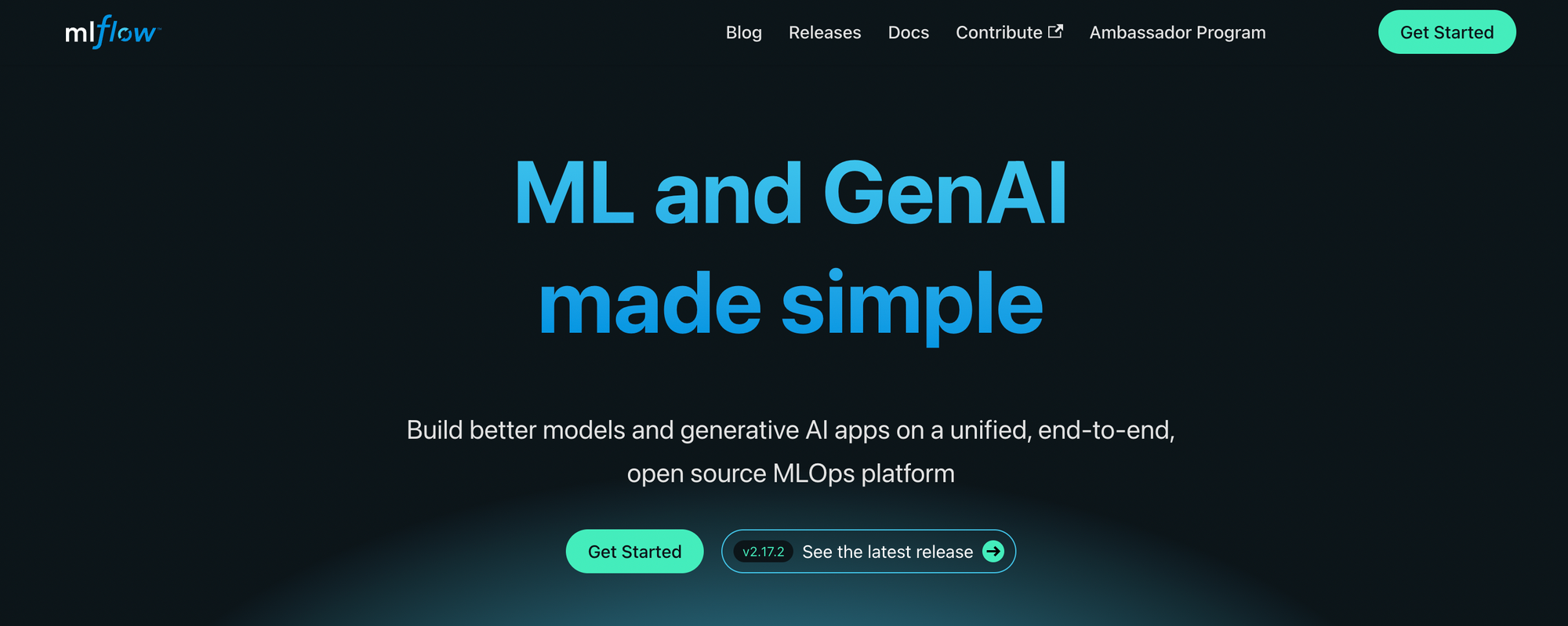
MLflow is an open-source platform designed to manage the ML lifecycle, including:
- Experimentation
- Reproducibility
- Deployment
A Comprehensive ML Toolkit
It offers four primary components: MLflow Tracking to log experiments, MLflow Projects for packaging ML code, MLflow Models for managing and deploying models across frameworks, and MLflow Registry to centralize model management. This comprehensive toolkit simplifies processes across the machine learning lifecycle, making it easier for teams to collaborate, track, and deploy their ML models efficiently.
Limitations: MLflow is versatile and powerful for experiment tracking and model management, but it faces challenges in areas like security and compliance, user access management, and the need for self-managed infrastructure. It also has issues with scalability and is limited in terms of features.
7. Valohai: MLOps for Machine Learning Pioneers
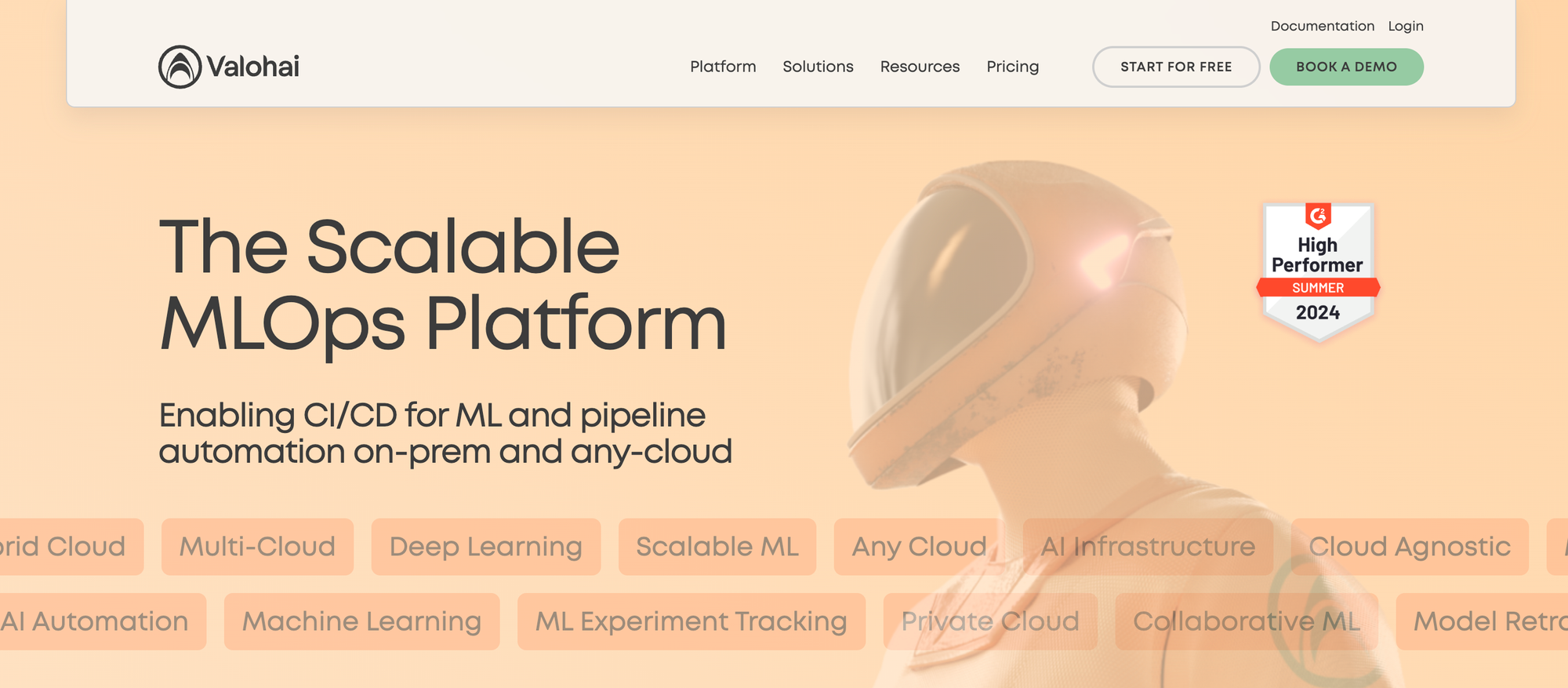
Valohai is an MLOps platform engineered for machine learning pioneers. It aims to streamline the ML workflow by providing tools that automate machine learning infrastructure. This empowers data scientists to orchestrate machine learning workloads across various environments, whether cloud-based or on-premise.
With features designed to manage complex deep learning processes, Valohai facilitates the efficient tracking of every step in the machine learning model's life cycle.
Limitations: Valohai promises to automate and optimize the deployment of machine learning models, offering a comprehensive system that supports batch and real-time inferences. Users looking to utilize this platform must manage the complexity of integrating it within their existing systems. They might face challenges if they need to familiarize themselves with handling extensive ML workflows and infrastructure management.
8. Google Cloud AI Platform: Google Cloud's Unified Platform for Machine Learning
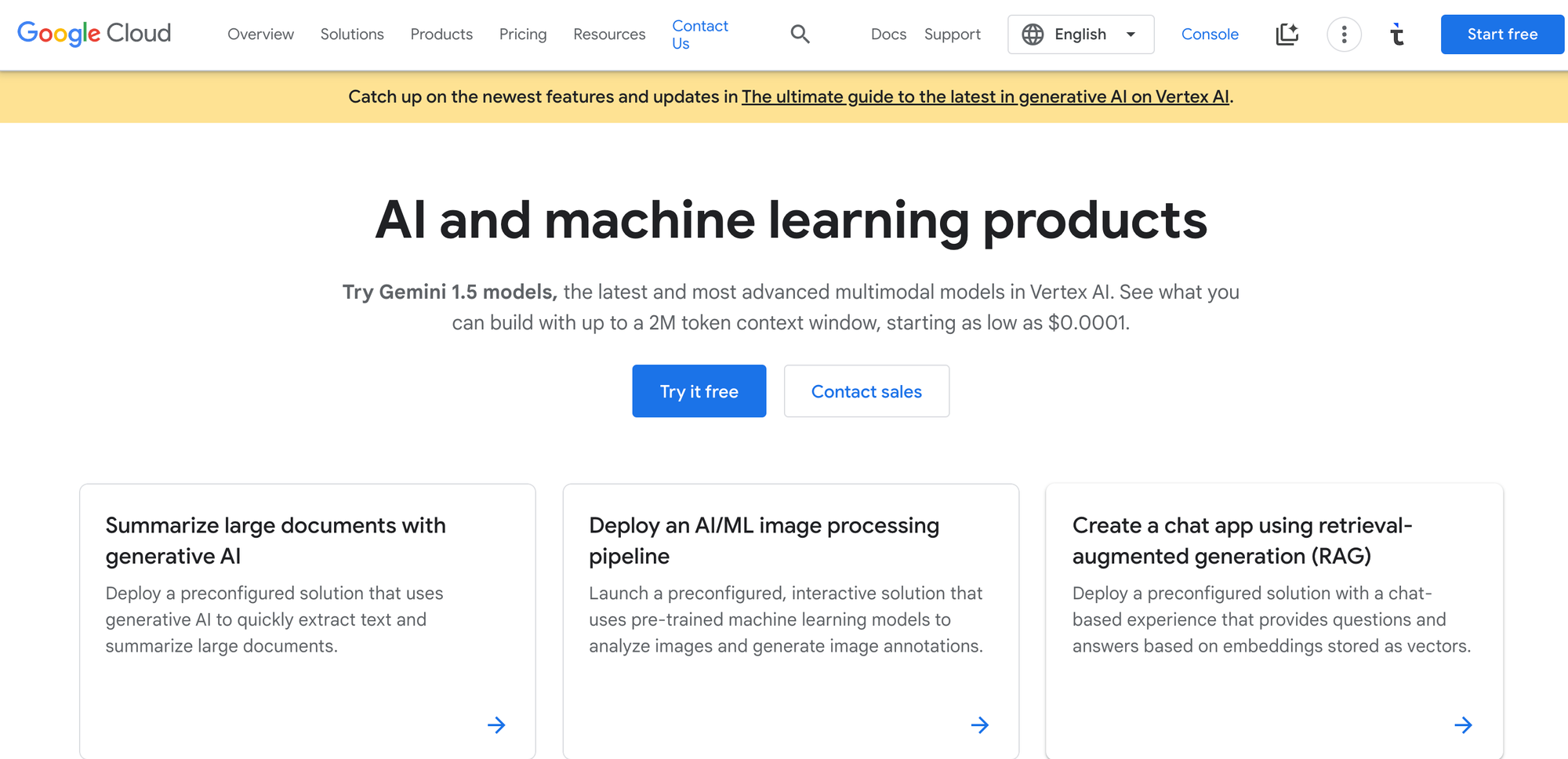
Google Cloud AI Platform is a machine learning platform offering various tools for building and deploying machine learning models. The platform includes features like autoML, which can automatically build and train models, and Kubeflow, which provides a scalable and portable machine-learning workflow.
Features and Benefits
Google Cloud AI Platform offers a range of features, including support for TensorFlow, PyTorch, and scikit-learn, as well as pre-built models for common use cases like image and text classification. The platform also offers integration with other Google Cloud services, like:
- BigQuery
- Cloud Storage
Limitations: One potential limitation of Google Cloud AI Platform is its pricing, which can be more expensive than other options on the market. Some users may find the platform’s interface less intuitive than other options.
9. Microsoft Azure Machine Learning Studio: A User-Friendly Machine Learning Tool
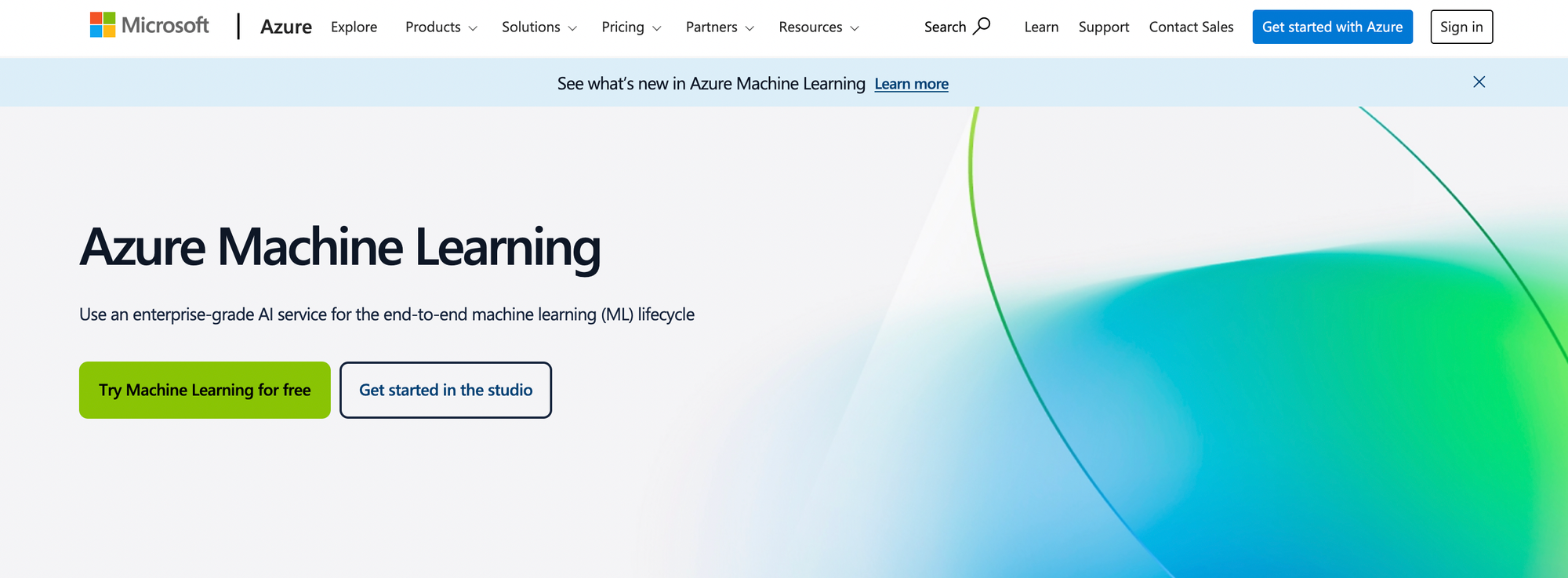
Microsoft Azure Machine Learning Studio is a cloud-based platform that provides tools for building, training, and deploying machine learning models. The platform includes drag-and-drop model building and integration with other Azure services.
Features and Benefits
Azure Machine Learning Studio offers a range of features, including support for R and Python and pre-built models for common use cases like fraud detection and sentiment analysis. The platform also offers integration with other Azure services, like:
- Azure Data Factory
- Azure DevOps
Limitations: One potential limitation of Azure Machine Learning Studio is its limited support for deep learning models. Additionally, some users may find the platform’s interface less intuitive than other options.
10. IBM Watson Studio: A Comprehensive Machine Learning Solution
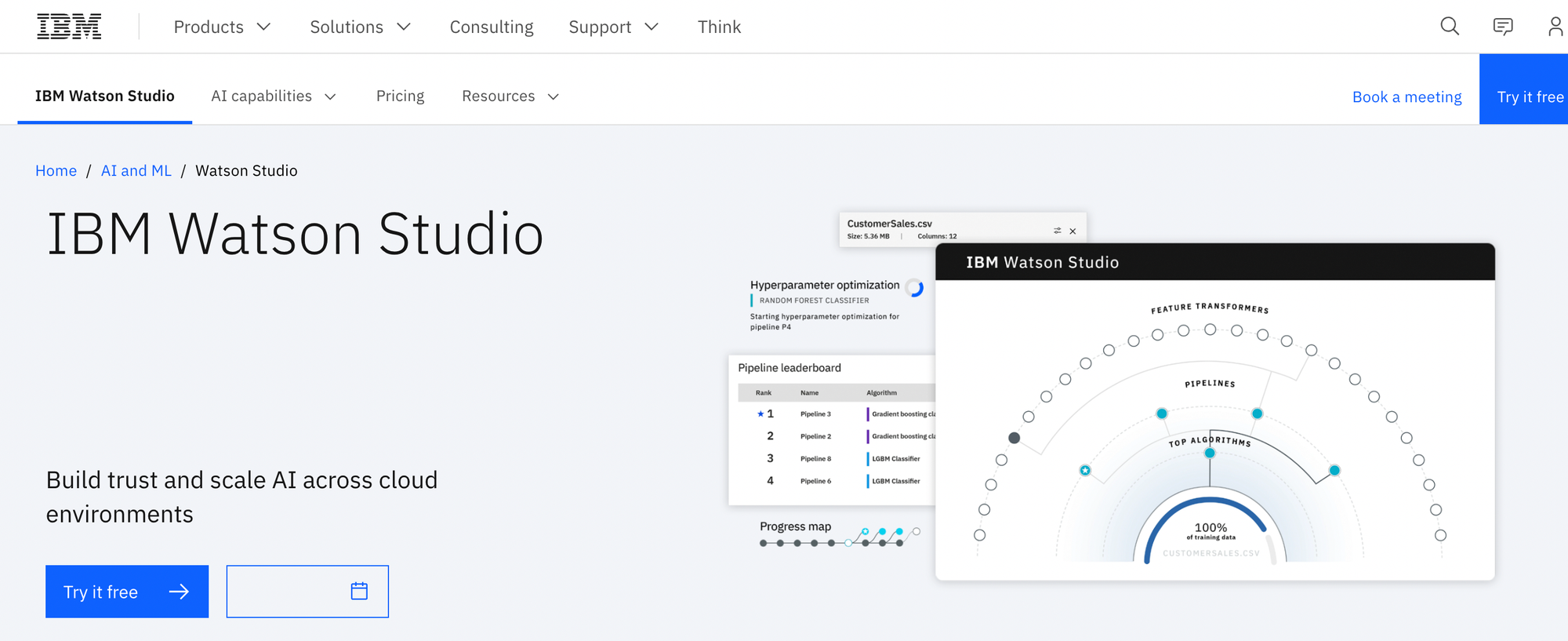
IBM Watson Studio is a cloud-based platform that provides tools for building, training, and deploying machine learning models. The platform includes features like autoAI, which can automatically build and train models, and integration with other IBM Watson services.
Features and Benefits
IBM Watson Studio offers a range of features, including support for R and Python and pre-built models for common use cases like image and text classification. The platform also offers integration with other IBM Watson services, like:
- Watson Assistant
- Watson Discovery
Limitations: One potential limitation of IBM Watson Studio is its pricing, which can be more expensive than other options on the market. Additionally, some users may find the platform’s interface less intuitive than other options.
11. DataRobot: An Easy-to-Use Machine Learning Platform
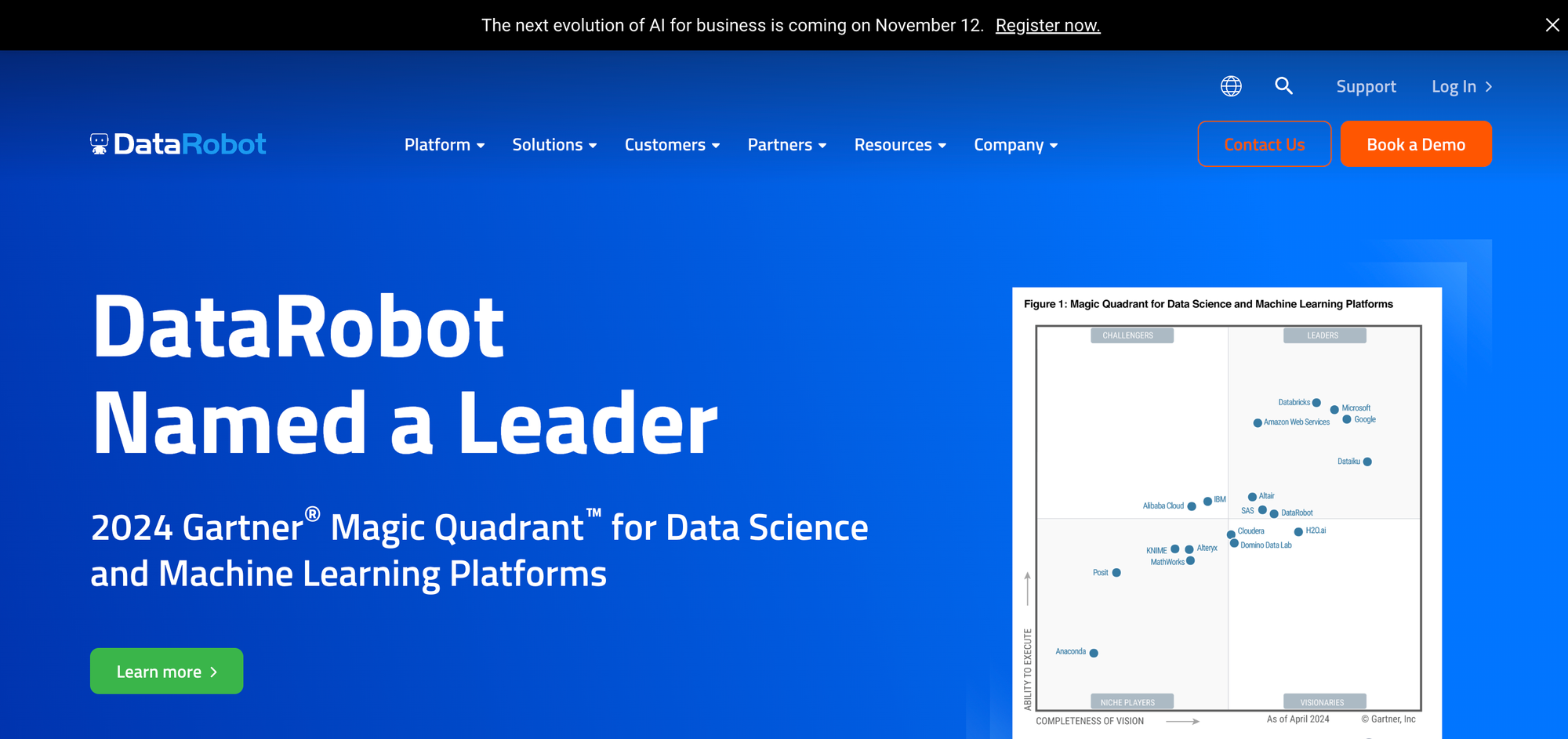
DataRobot is a machine learning platform that provides data scientists with various tools for building, training, and deploying machine learning models. The platform is designed to be easy to use, with a user-friendly interface and intuitive tools for data visualization and model building.
Features and Benefits
DataRobot offers a range of features for data scientists, including:
- Support for different machine learning models
- Data visualization tools
- Integration with other tools
The platform also offers automated machine learning, making it easy for data scientists to build and deploy models quickly.
Limitations: One limitation of DataRobot is that it may be more expensive than other platforms, with pricing plans based on usage and enterprise needs. The platform also has a steeper learning curve compared to some other alternatives.
12. H2O.ai: A Scalable Machine Learning Platform
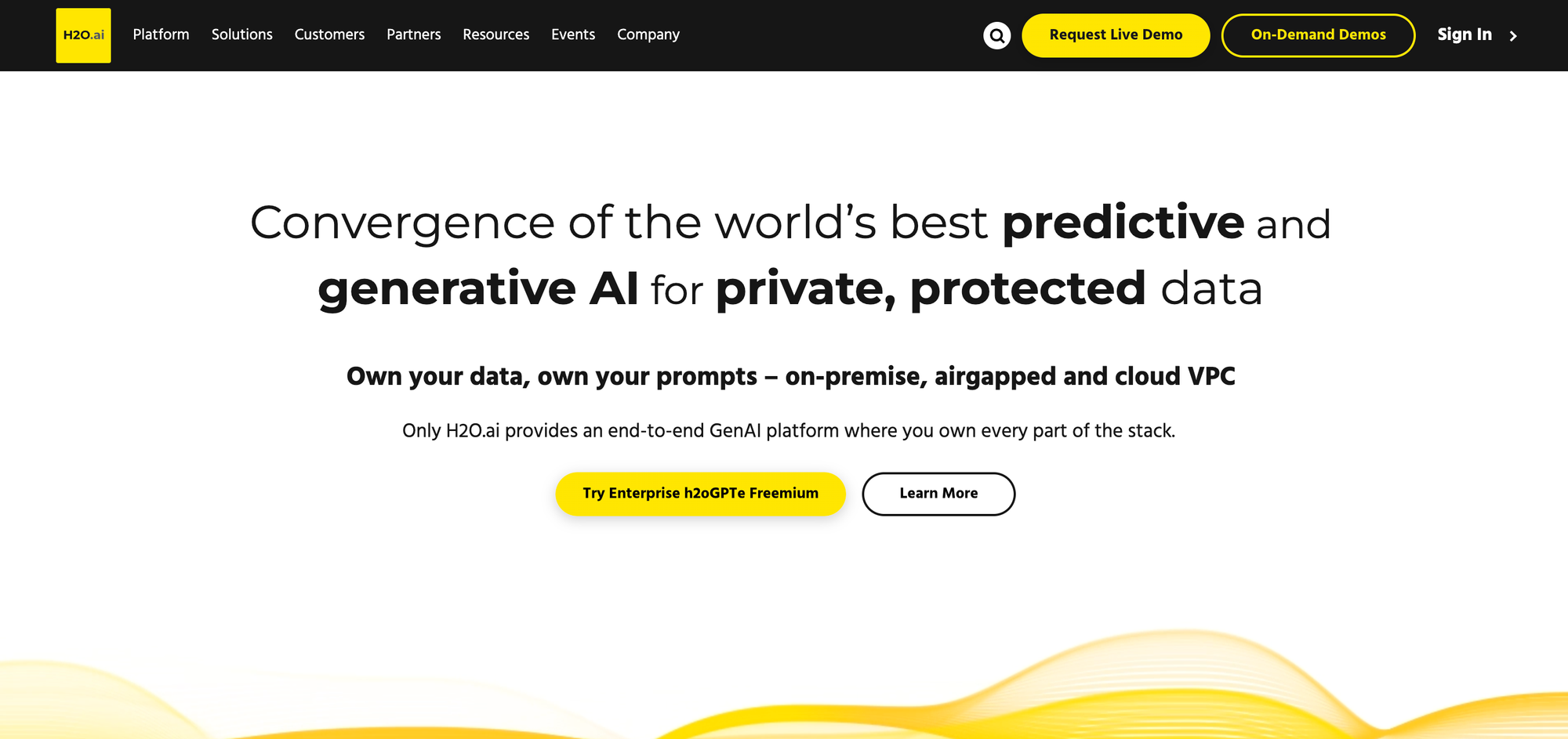
H2O.ai is a machine learning platform that provides tools for:
- Building
- Training
- Deploying machine learning models
The platform includes automatic machine learning and integration with other data science tools.
Features and Benefits
H2O.ai offers a range of features, including support for R and Python and pre-built models for common use cases like fraud detection and customer churn. The platform also integrates with other data science tools, like Tableau and Excel.
Limitations: One potential limitation of H2O.ai is its limited support for deep learning models. Additionally, some users may find the platform’s interface less intuitive than other options.
13. Deepnote: A Real-Time Collaborative Notebook
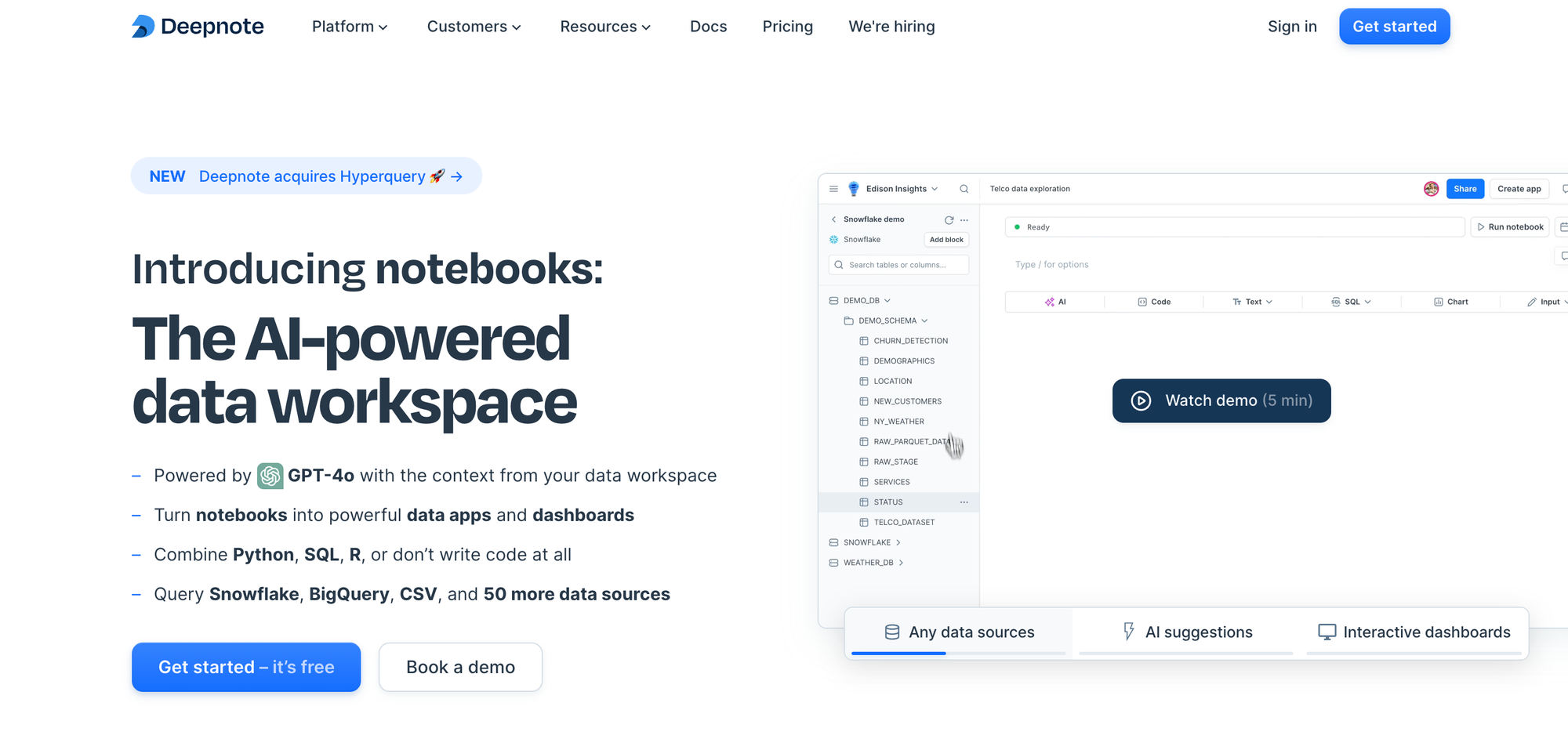
Deepnote is a real-time collaborative notebook. It directly integrates with popular data sources like GitHub, Google Drive, and BigQuery. Its modern, intuitive interface makes it a compelling choice for beginners and experienced data scientists.
Main Benefits
- Real-Time Collaboration: Multiple users can work on the same notebook simultaneously, making it ideal for team projects.
- Integrations: Integrations with popular data sources like GitHub, Google Drive, and BigQuery enhance its usability and efficiency.
- Smart Autocomplete: This feature accelerates code writing and reduces the chance of errors.
14. JupyterLab: The Next Generation of Jupyter Notebooks
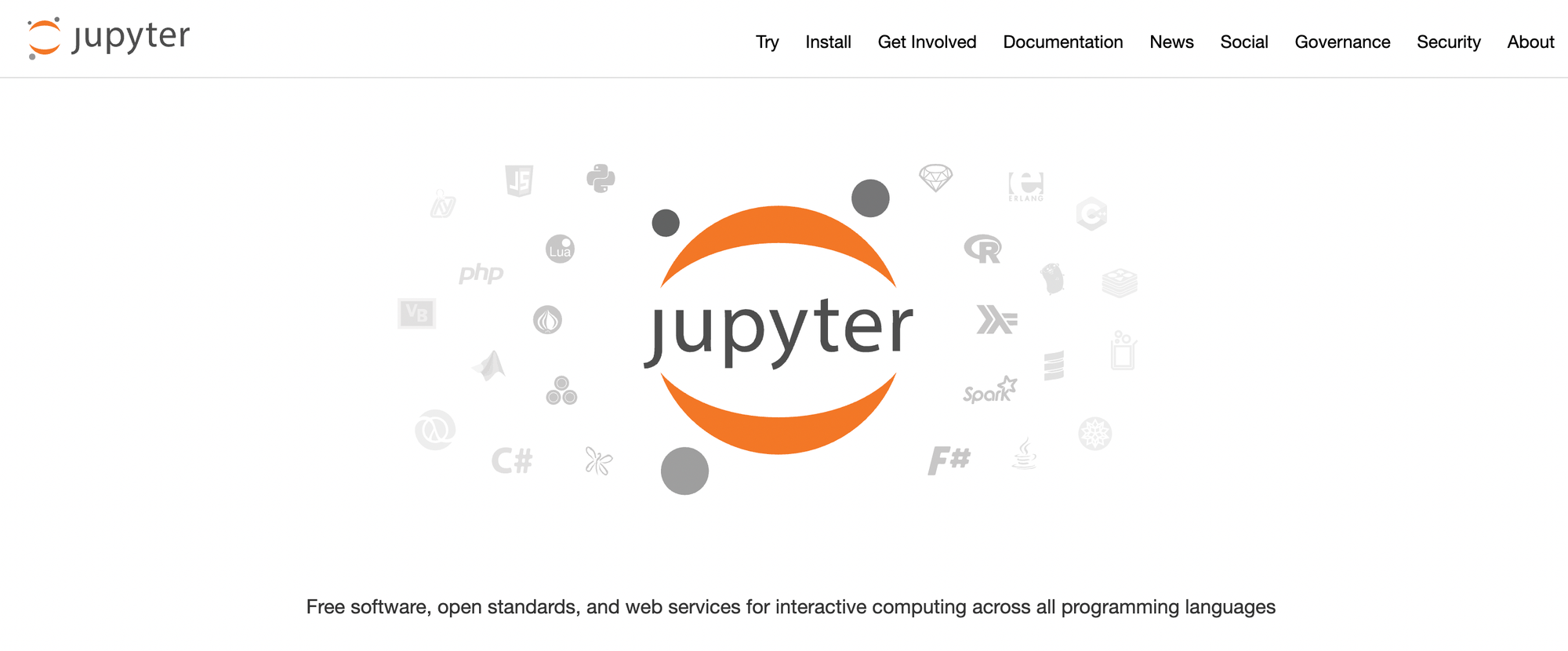
JupyterLab is the next-generation user interface for Project Jupyter. Like Amazon SageMaker Studio, it's an interactive development environment for working with:
- Notebooks
- Code
- Data
JupyterLab offers more flexibility as it can be self-hosted, enabling users to use their hardware resources. It also supports extensions for integrating other services, making it a highly customizable and versatile tool for data scientists.
Main Benefits
- Self-Hosting: This feature provides the flexibility to use your hardware resources.
- Extension Support: Its extension support allows for integrating other services, enhancing customization and versatility.
15. Kaggle Kernels: A Free ML Playground with Community Integration
Kaggle is best known for its data science competitions, but it also offers a cloud-based computation environment known as Kaggle Kernels. Unlike Microsoft Azure Notebooks, it provides free access to GPUs and supports Python, R, and Julia languages. It is also deeply integrated with other Kaggle features, providing direct access to many datasets and community notebooks, making it an excellent learning platform.
Main Benefits
- Community Integration: It is deeply integrated with other Kaggle features, providing direct access to many datasets and community notebooks.
- Language Support: It supports multiple languages, including Python, R, and Julia.
- Free GPU Access: Unlike Microsoft Azure Notebooks, it provides free access to GPU resources.
16. Databricks Community Edition: Cloud-Based ML with Apache Spark

Databricks is a platform built around Apache Spark, an open-source, distributed computing system. The Databricks Community Edition offers a collaborative workspace where users can create Jupyter notebooks. Although it doesn't offer free GPU resources, it's an excellent tool for distributed data processing and big data analytics.
Main Benefits of Databricks Community Edition
- Scalability: It's an excellent tool for distributed data processing and big data analytics.
- Spark Integration: The platform is built around Apache Spark, allowing for high-performance analytics.
17. Google Colab: A Powerful SageMaker Alternative for ML Projects
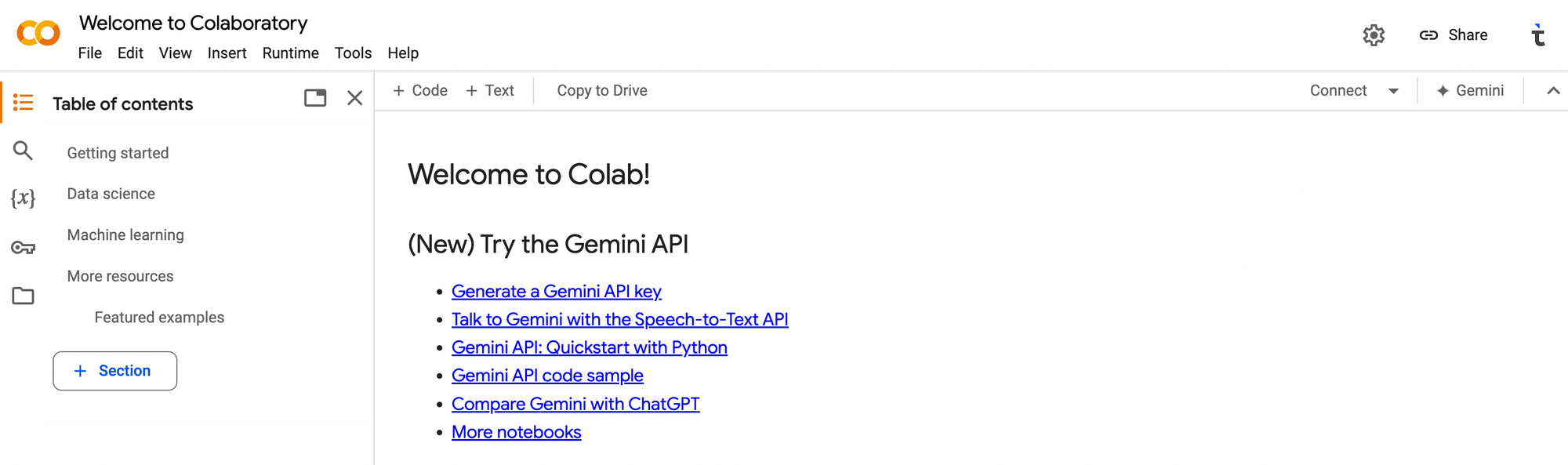
Google Colab is Google's direct answer to Amazon SageMaker Studio. Colab weights Google behind it and thus plays very well with many Google products, such as:
- BigQuery
- Google Drive
- AlloyDB
- Dataproc
- Cloud SQL
Main Benefits
- Google Ecosystem Integration: It is well-integrated into the wider Google ecosystem, providing easy access to robust data pipelines, storage solutions, and machine learning models.
- Enterprise-Friendly: It's a powerful choice for enterprises already using Google Cloud Platform for their cloud computing needs.
- Collaboration Tools: Google Colab offers various collaboration and sharing features, making it easy to work in teams.
18. Microsoft Azure Notebooks: Another SageMaker Alternative
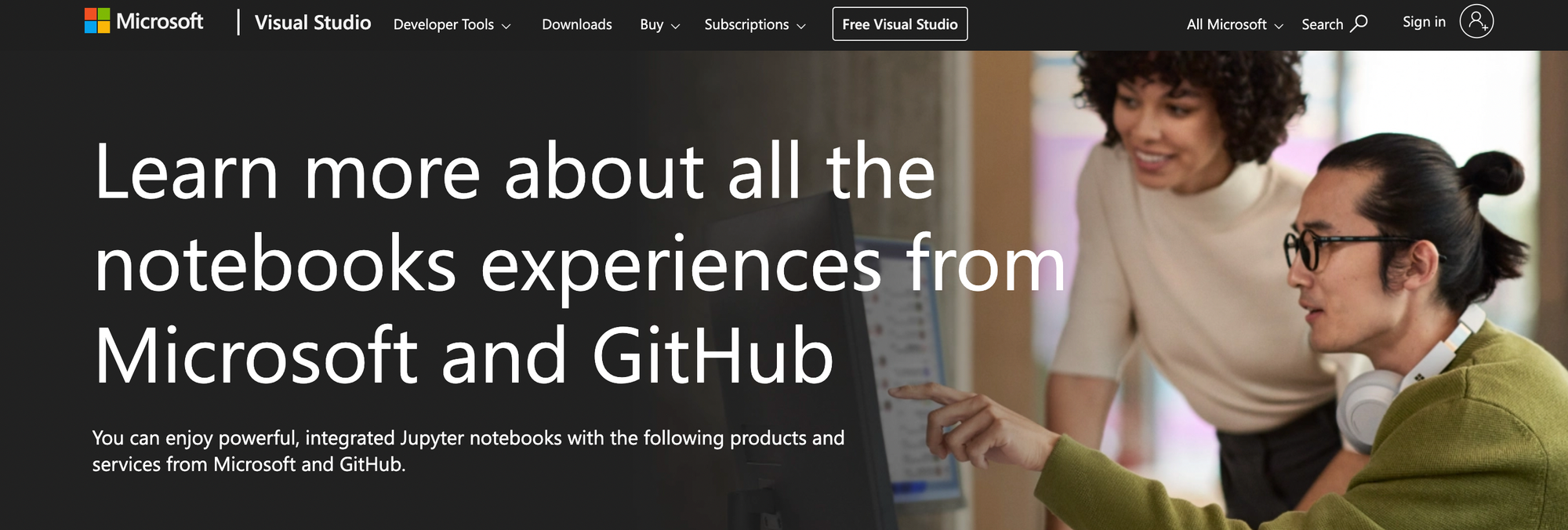
Azure Notebooks is Microsoft's direct answer to Amazon SageMaker Studio. It offers similar Jupyter notebook functionality with additional integration into the wider Azure ecosystem. This allows easy access to:
- Robust data pipelines
- Storage solutions
- Machine learning models available in Azure
It's a powerful choice for enterprises already using Azure for cloud computing.
Main Benefits
- Azure Ecosystem Integration: It is well-integrated into the wider Azure ecosystem, providing easy access to robust data pipelines, storage solutions, and machine learning models.
- Enterprise-Friendly: It's a powerful choice for enterprises already using Azure for their cloud computing needs.
- Collaboration Tools: Azure Notebooks offer various collaboration and sharing features, making it easy to work in teams.
19. Gradient by Paperspace: An Easy-To-Use ML Platform
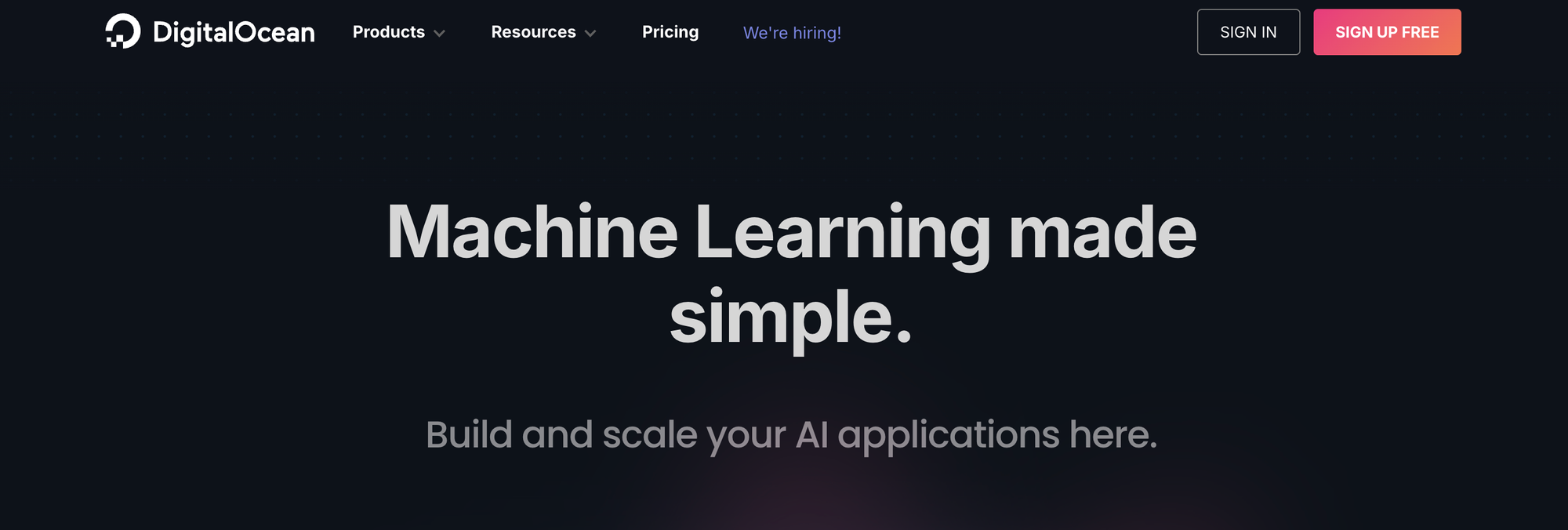
Gradient by Paperspace is a robust alternative for developing, training, and deploying machine learning models quickly. With a free GPU tier and one-click Jupyter notebooks, it's an easy-to-use platform that doesn't compromise on functionality. Gradient is also known for its powerful experiment tracking and version control capabilities.
Main Benefits
- Ease of Use: Gradient offers one-click Jupyter notebooks, making it incredibly easy to start training models. It's an excellent platform for beginners and experienced data scientists alike.
- Experiment Tracking: Gradient provides robust experiment tracking capabilities, which help manage and compare different model training runs. This is crucial for fine-tuning models and selecting the best-performing one.
- Free GPU Access: Unlike Amazon SageMaker Studio, Gradient offers a free tier that includes access to a GPU, which can be a great advantage for those who are just getting started with deep learning or working on smaller projects.
20. Runhouse: An Open Source Alternative to SageMaker
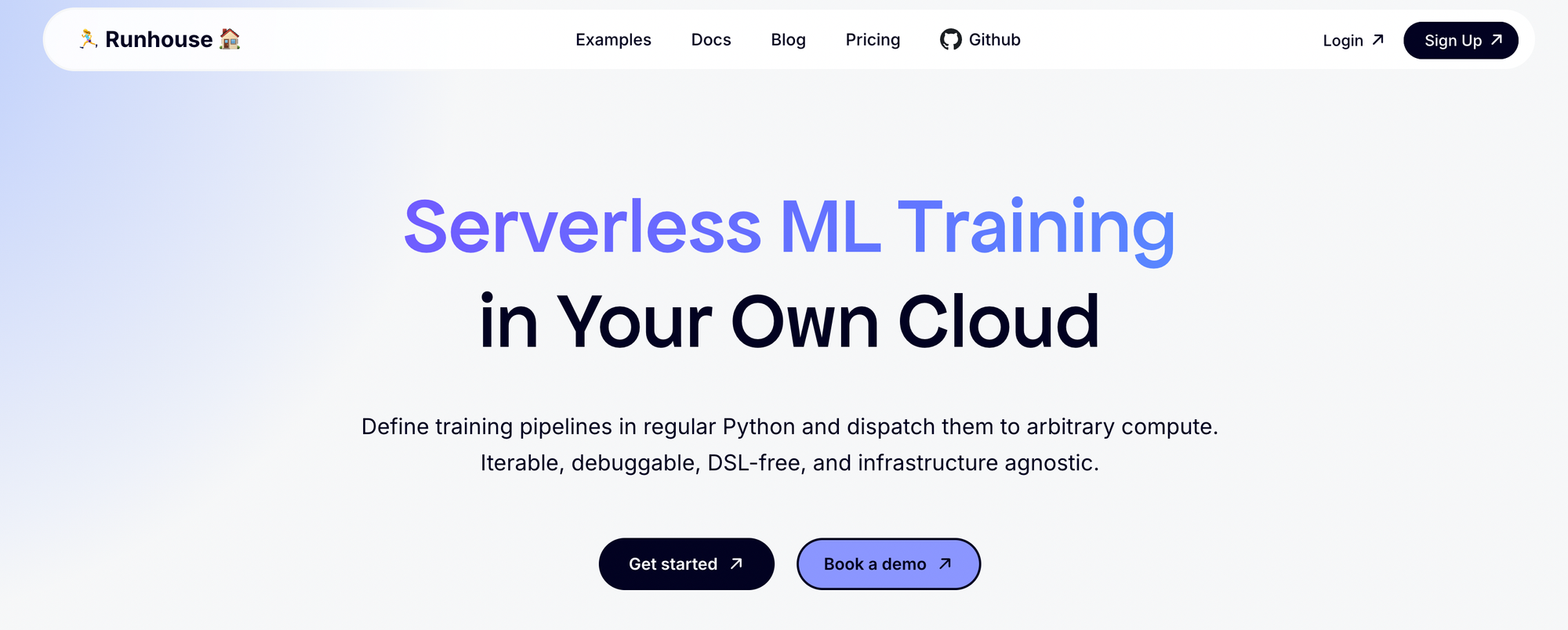
Runhouse is built to deliver benefits without the costs of SageMaker. Using Runhouse as a foundational framework for your ML platform delivers all the benefits of an easy-to-use platform while building on the same best practice principles we’ve observed at top-tier ML practices. Runhouse is:
- Open source
- Can write normal Python code, no domain-specific language and no vendor lock-in
- Is easy to stand up, working as a universal runtime on any infrastructure and compute
- Can flexibly use computers across multiple regions, clusters, and even cloud providers.
- Share and collaborate with functions and modules that are reusable and trackable from central management
Related Reading
- Gen AI Architecture
- Generative AI Implementation
- Gen AI Platforms
- Generative AI Challenges
- Generative AI Providers
- How to Train a Generative AI Model
- Generative AI Infrastructure
- AI Middleware
- Top AI Cloud Business Management Platform Tools
- AI Frameworks
- AI Tech Stack
Start Building GenAI Apps for Free Today With Our Managed Generative AI Tech Stack
Building a generative AI application involves various steps. Lamatic’s managed generative AI tech stack simplifies this process by providing the building blocks you need to start quickly. For example, the platform includes automated workflows that help you seamlessly move from development to deployment to get to business faster.
Lamatic’s tools help you identify and resolve issues before they become problematic, ensuring your production deployment is stable and reliable.
GenAI Middleware: The Heart of Lamatic's Tech Stack
Lamatic's generative AI middleware sits at the platform's core and provides the crucial connectivity needed between your application and underlying AI models. It helps to:
- Automate workflows
- Streamline processes
- Eliminate technical debt by providing out-of-the-box solutions so you don’t have to build everything from scratch.
This component of Lamatic's platform will help you get to market quickly and at a lower cost.
Custom GenAI API (GraphQL)
Lamatic's custom GenAI API based on GraphQL helps developers build applications that communicate with generative AI models. The API comes preconfigured to help you connect to Weaviate, an integrated vector database that stores and organizes data for AI applications. The custom API will help you get to market faster with reliable, production-grade applications.
Low-Code Agent Builder
Build your GenAI application with Lamatic's low-code agent builder, allowing developers to
create intelligent agents with little to no coding. The interface uses a visual approach that helps users organize tasks and build workflows through simple drag-and-drop functionality.
This makes it easy to create custom applications for specific business needs and reduce technical debt by providing out-of-the-box solutions that eliminate the need for excessive coding.
Automated GenAI Workflows (CI/CD)
Lamatic's managed generative AI stack helps you implement automated workflows that streamline the development and deployment of generative AI applications. Continuous integration and continuous deployment (CI/CD) workflows allow you to quickly identify and resolve issues in your code before they become problematic.
This makes it easier to ensure stable, reliable applications that can perform under stress in production environments.
GenOps: DevOps for GenAI
As organizations deploy more generative AI applications, they need a way to manage these complex systems. GenOps, or DevOps for generative AI, helps teams do just that. Lamatic’s platform helps automate the deployment and management of GenAI applications to eliminate technical debt and improve application performance over time.
This makes ensuring your applications are reliable and meet business goals easier.
Edge Deployment via Cloudflare Workers
The Lamatic platform helps you deploy generative AI applications at the edge using Cloudflare Workers. This means your applications can run on servers closest to end users instead of a centralized data center. This drastically reduces latency and improves application performance, creating better user experiences.
Deploying applications at the edge also helps improve security and reduces the risk of a costly data breach.
Integrated Vector Database (Weaviate)
Lamatic's managed generative AI tech stack has an integrated vector database to help you get to market faster. Weaviate is a leading open-source AI database that stores and organizes unstructured data for generative AI applications.
By including it as part of the Lamatic platform, you can eliminate the guesswork involved in connecting to a database and ensure your application uses reliable, production-grade resources from the start.
Related Reading
- AI Application Development
- Best AI App Builder
- AI Development Platforms
- AI Development Cost
- Gemini Alternatives
- LangChain Alternatives
- Flowise AI