To integrate generative AI into your product, you need the right tools. But with so many to choose from, how do you find the best fit? If you've been researching Langsmith for your LLM app development, you may have discovered it's not the only option. Exploring Langsmith alternatives can provide the insights you need to enhance app performance, accelerate growth, and minimize complexity. This article will help you seamlessly scale generative AI in your product by finding the best Langsmith alternative for your objectives and multi agent AI. Lamatic's generative AI tech stack can help you achieve your goals, like enhancing performance, accelerating development, and minimizing complexity.
11 Best Langsmith Alternatives for Smarter LLM Apps & Faster AI Development
1. Lamatic: A Managed Generative AI Tech Stack to Rapidly Build GenAI Apps
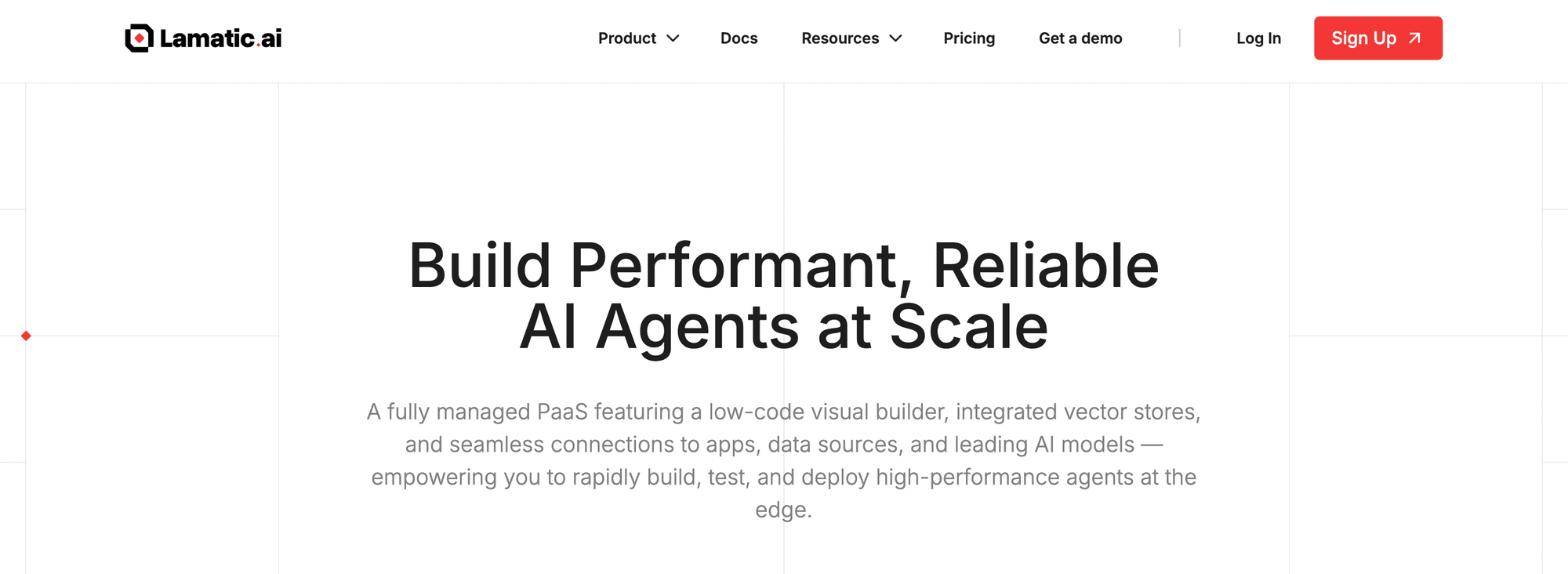
Lamatic offers a managed Generative AI Tech Stack. Our solution provides:
- Managed GenAI Middleware
- Custom GenAI API (GraphQL)
- Low Code Agent Builder
- Automated GenAI Workflow (CI/CD)
- GenOps (DevOps for GenAI)
- Edge deployment via Cloudflare workers
- Integrated Vector Database (Weaviate)
Lamatic empowers teams to rapidly implement GenAI solutions without accruing tech debt. Our platform automates workflows and ensures production-grade deployment on edge, enabling fast, efficient GenAI integration for products needing swift AI capabilities. Start building GenAI apps for free today with our managed generative AI tech stack.
2. Orq.ai: A Collaborative Hub for Generative AI Teams
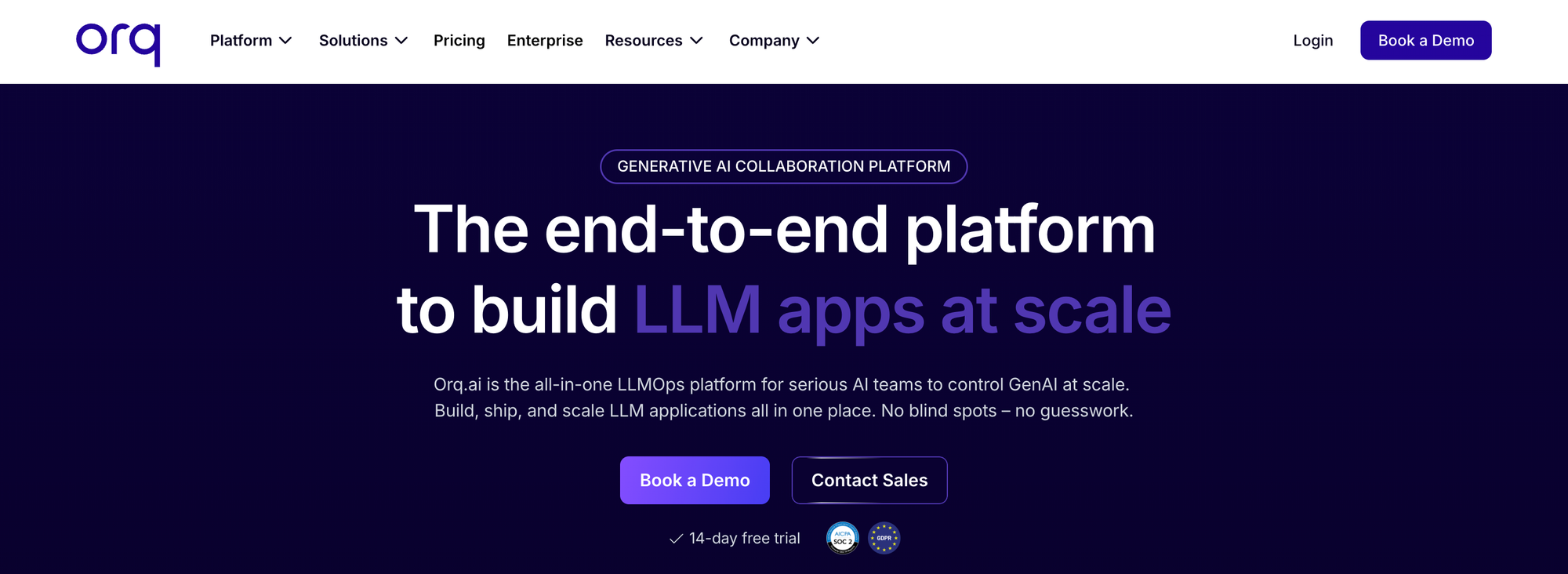
Orq.ai is an advanced Generative AI Collaboration Platform designed to help AI teams develop, deploy, and optimize large language models (LLMs) at scale. Launched in February 2024, Orq.ai provides a powerful tool suite that streamlines the entire AI application lifecycle. With its integration capabilities and user-friendly interface, Orq.ai transforms how teams interact with AI models and deploy them to production.
Orq.ai: A Scalable Solution for LLM Observability and Collaboration
Orq.ai offers a comprehensive, end-to-end solution for managing LLM observability, from initial development to deployment and ongoing optimization. Whether looking for LangChain alternatives or a platform that supports model drift detection and annotation for detailed analysis, Orq.ai delivers the tools necessary for scaling and optimizing AI applications efficiently.
It bridges the gap between technical and non-technical teams, making it easy for everyone to collaborate on AI projects and deploy at scale. As a relatively new player in the market, Orq.ai may have fewer community-driven resources and third-party integrations than more established platforms. Teams accustomed to longer-term, highly supported tools might need to invest time in fully exploring and adopting the platform.
3. Helicone: Open Source LLM Observability and Monitoring
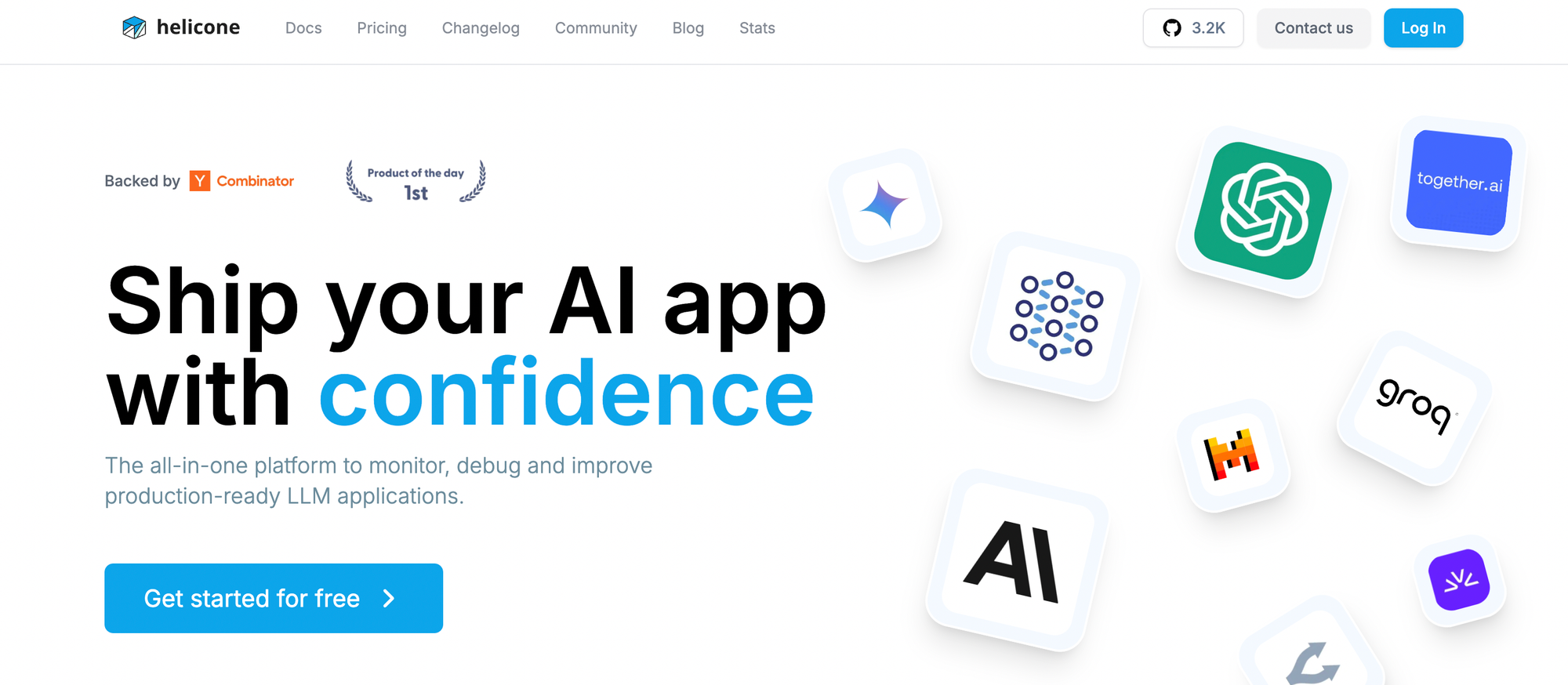
Helicone is an open-source framework for LLM observability and monitoring, specifically designed for developers who need to track, debug, and optimize large language models efficiently. Offering both self-hosted and gateway deployment options, Helicone provides the flexibility to scale observability efforts without sacrificing control or performance. It is a strong competitor among LangChain competitors and is well-suited for teams that prefer open-source solutions that can customize the platform according to their needs.
Helicone is a strong LLM observability alternative, especially for developers seeking an open-source platform that offers flexibility and scalability. While it excels in providing cost-effective and customizable solutions for developers, it may not be the best fit for larger enterprises or non-technical teams due to its limited enterprise features and steeper learning curve.
4. Phoenix Arize AI: Model Monitoring and Explainability
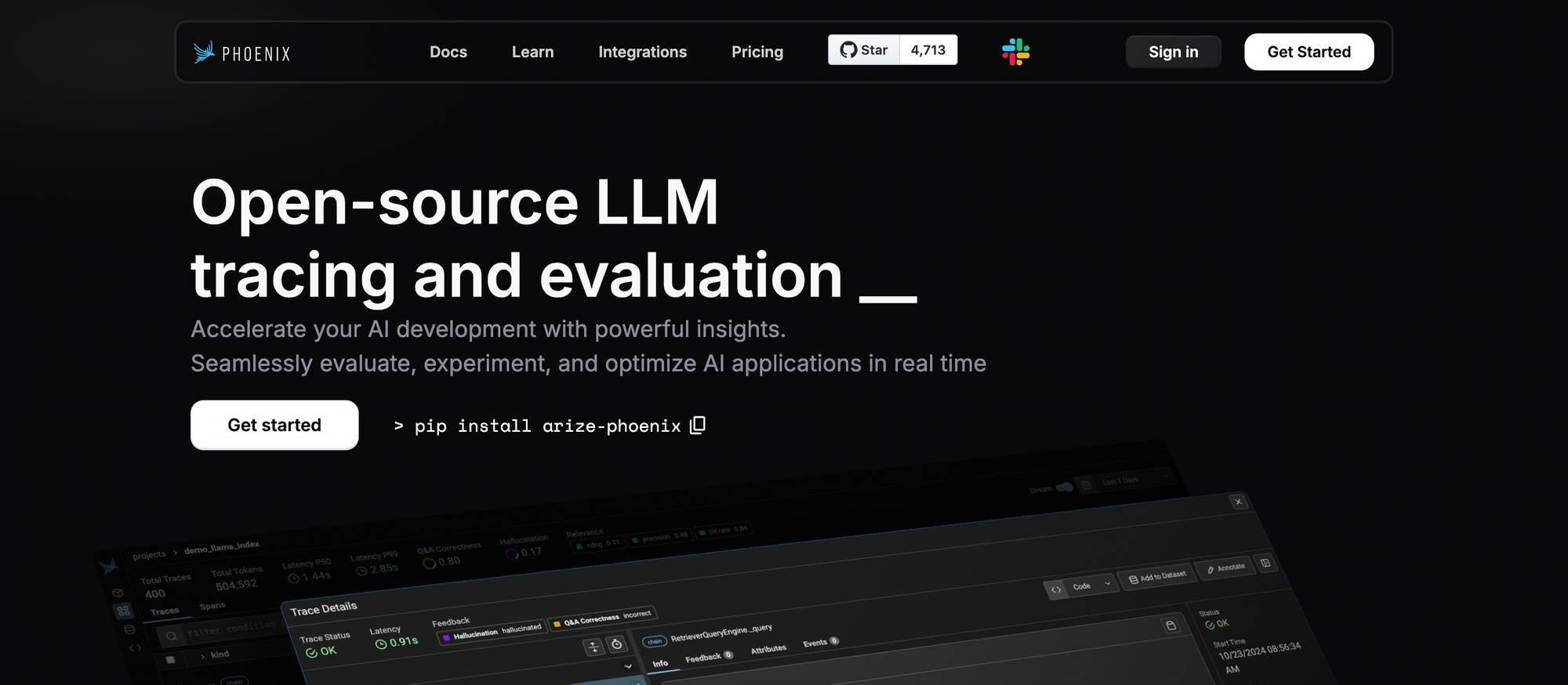
Phoenix by Arize AI is a specialized platform designed to help teams monitor, evaluate, and optimize their AI models at scale. Focusing on model explainability, Phoenix provides advanced tools for tracking and improving the performance of large-scale AI systems.
Phoenix offers a robust solution for organizations seeking to dive deeper into evaluation metrics and detect model drift.
Phoenix: Focused LLM Insights and When to Pair It with Other Tools
Phoenix stands out for its emphasis on deep insights into the performance and behavior of LLM applications. Its comprehensive tools for tracking model drift and providing actionable explanations make it an excellent choice for teams working with high-stakes AI models.
While Phoenix shines in explainability and evaluation, its narrower focus may not cater to teams looking for an all-in-one solution. For organizations seeking a broader set of observability features—such as prompt templating or full deployment capabilities—it might be necessary to pair Phoenix with other tools.
5. Langfuse: An Open-Source Alternative for LLM Observability
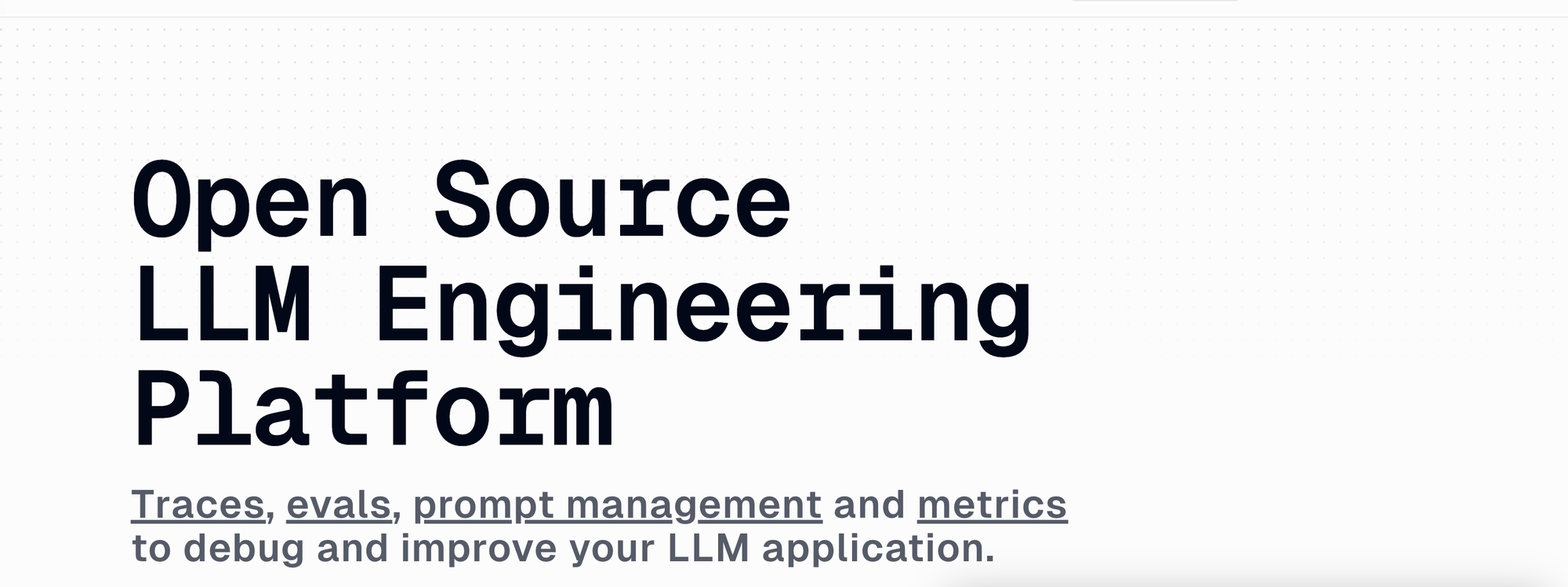
For teams seeking an open-source alternative to LangSmith, Langfuse delivers a robust and transparent platform for LLM observability. Its self-hosted architecture ensures that teams maintain complete control over their data and deployment environments, making it an attractive option for organizations prioritizing customization and data security.
Langfuse: The Pros and Cons of an Open-Source, Self-Hosted LLM Observability Platform
Langfuse is an ideal solution for:
- Teams prioritizing transparency
- Customization
- The ability to self-host their observability platform
Its open-source nature means organizations can adapt the tool as their needs evolve, while community-driven development ensures continuous updates and improvements.
Teams considering Langfuse should know that relying on an open-source framework might require more internal setup, maintenance, and scaling resources. The self-hosted deployment option could introduce additional complexity for organizations without dedicated technical expertise.
6. HoneyHive: An Observability Tool with a Focus on Customer Engagement
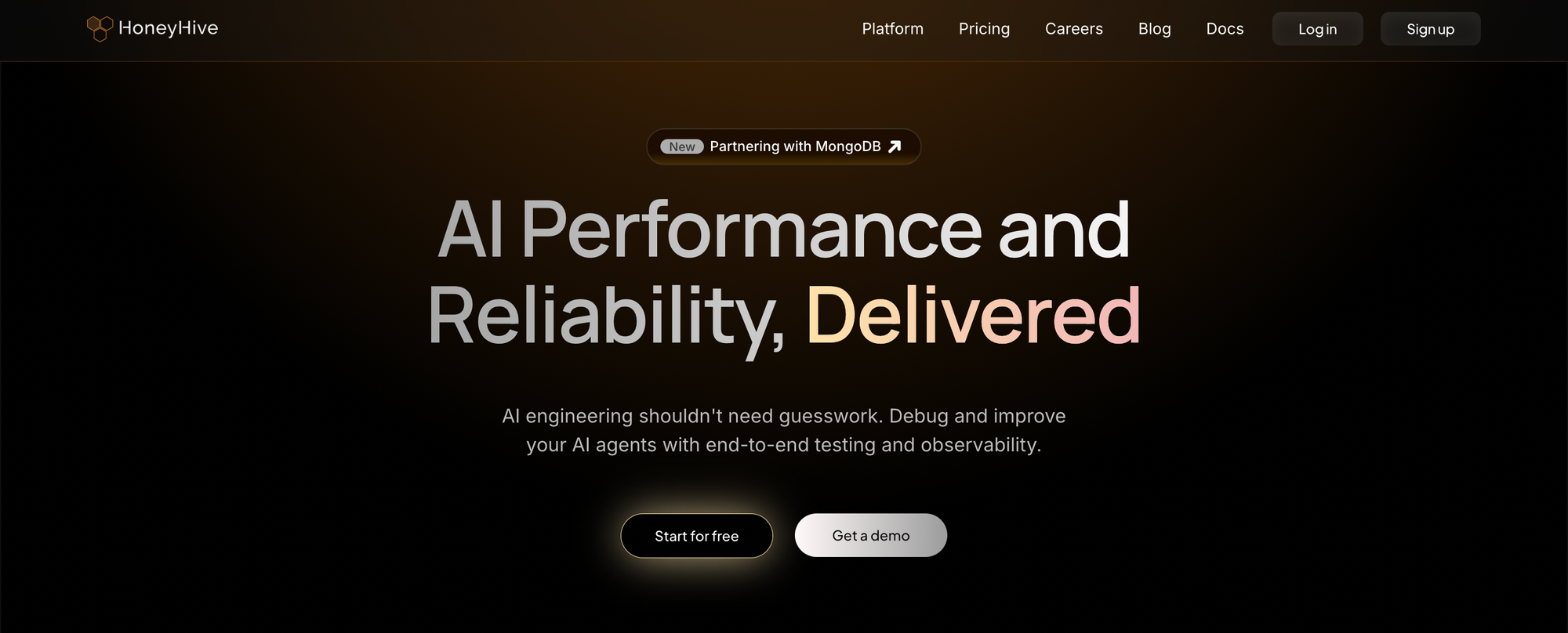
HoneyHive distinguishes itself as a LangSmith alternative by emphasizing user tracking and engagement analytics, making it a strong choice for teams prioritizing customer experience and application optimization.
Designed for startups and smaller companies, HoneyHive’s affordability and intuitive interface enable teams to gather actionable insights without overextending their budgets.
HoneyHive: Balancing User Feedback and LLM Observability for Better Customer Experience
HoneyHive is a standout solution for teams aiming to merge LLM observability with a focus on user feedback. Its affordable pricing, intuitive analytics, and robust engagement tools make it a compelling option for those prioritizing customer experience and performance optimization.
While HoneyHive excels in user engagement and feedback tracking, its feature set may not be as comprehensive as other platforms regarding advanced evaluation or model drift detection. Larger enterprises or teams needing deeper AI optimization features might find it more suitable as a supplementary tool than a standalone solution.
7. OpenLLMetry by Traceloop: Customizable LLM Observability
OpenLLMetry, developed by Traceloop, is an open-source observability tool for LLM applications. It provides developers with powerful tools for tracking and optimizing LLM performance while offering the flexibility to customize and adapt the platform to fit specific workflows.
OpenLLMetry: A Community-Driven Approach to Customizable LLM Observability
OpenLLMetry is best suited for developers looking for a community-driven, customizable solution for LLM observability. Its open-source nature and focus on transparency make it a reliable choice for teams wanting to tailor their observability tools to their unique needs.
It’s worth noting that as a community-supported platform, OpenLLMetry may lack the dedicated customer support or polished user experience of proprietary tools. Teams without sufficient technical expertise or resources might find setup and maintenance more challenging than commercial options.
8. Weights & Biases (WandB): Experiment Tracking and LLM Observability
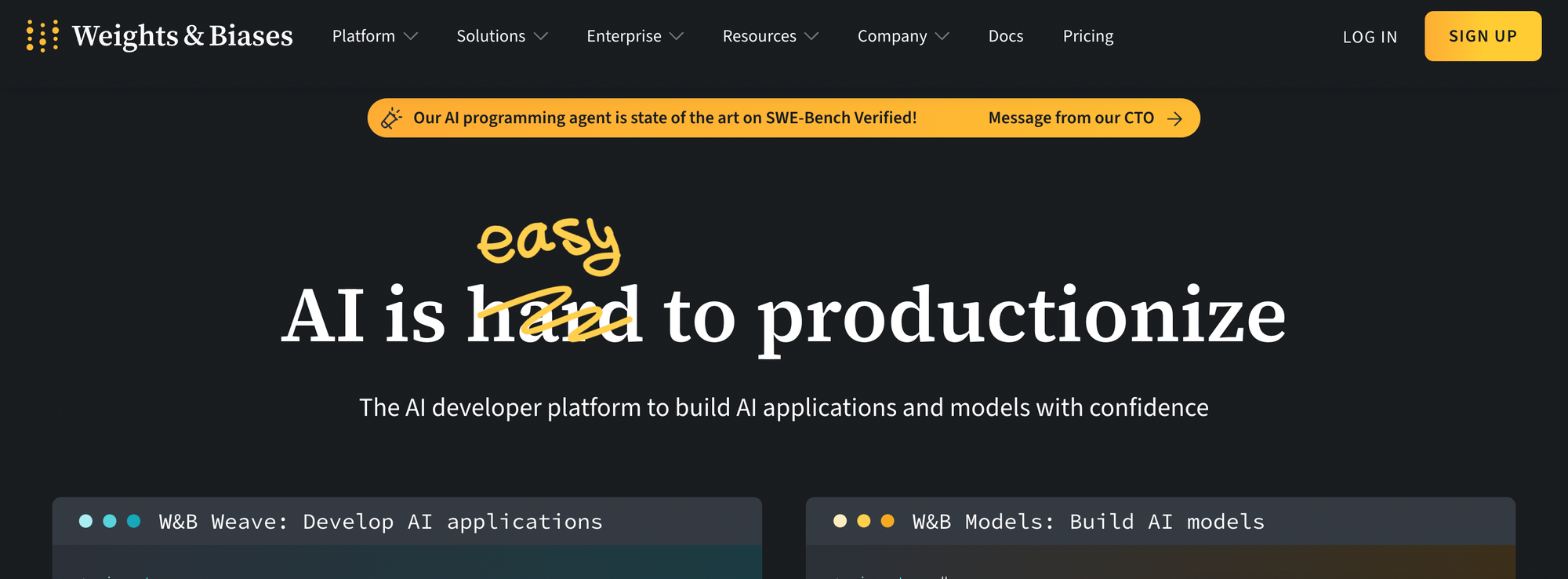
If you’ve ever been involved in machine learning or deep learning projects, Weights & Biases (WandB) is likely on your radar. While it focuses more on experiment tracking and hyperparameter tuning, it’s a fantastic alternative to Langsmith for anyone involved in research or developing highly experimental models.
WandB vs. LangSmith: Advanced Visualization and Deep Learning Integration
You’ll appreciate its seamless ability to track experiments over time and its strong emphasis on visualization. You might wonder: What sets WandB apart from Langsmith? The answer lies in its visualization capabilities.
WandB excels at giving detailed, interactive plots of your experiments, model performance metrics, and training progress. Unlike Langsmith, WandB integrates effortlessly with many deep learning frameworks (like TensorFlow and PyTorch), so it’s the better choice if you’re researching these frameworks.
9. MLflow: An Open-Source Tool for Managing Machine Learning Projects
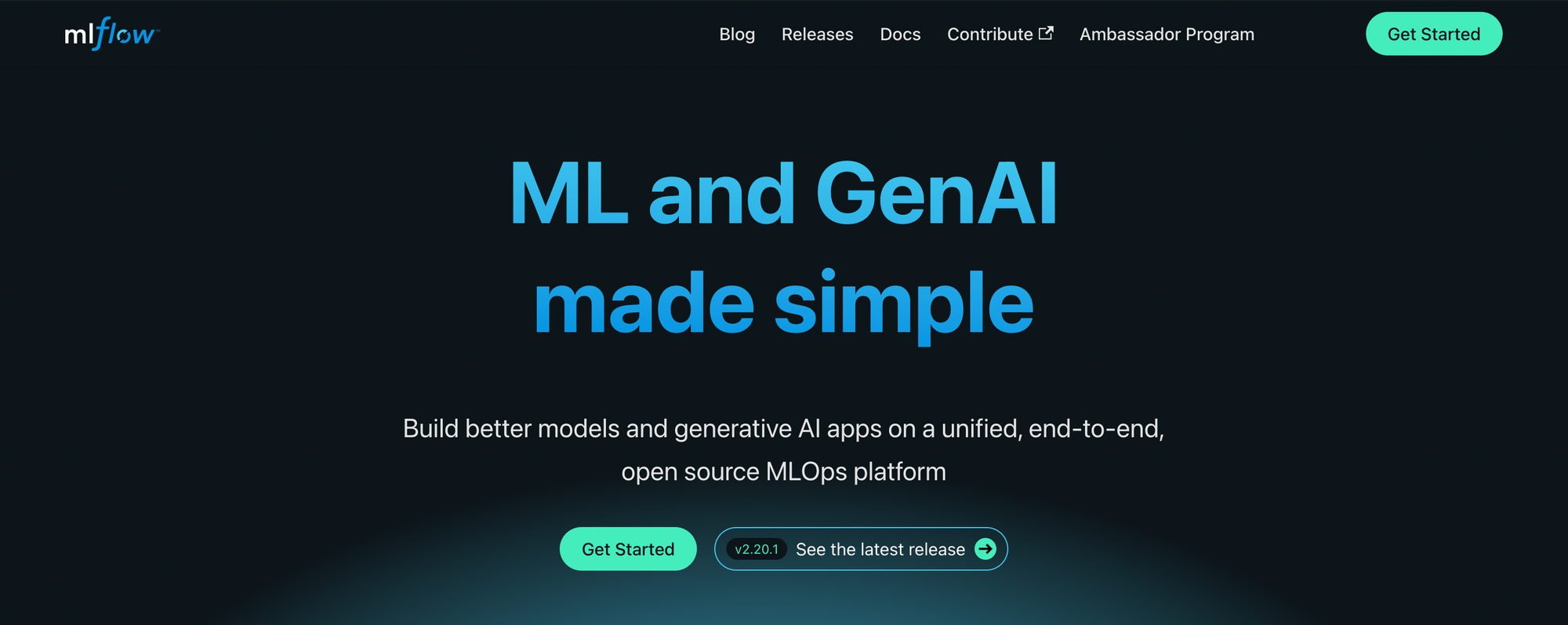
Regarding flexibility, MLflow is often hailed as one of the most versatile open-source tools for managing machine learning experiments. MLflow breaks away from Langsmith by offering a more decentralized approach to handling your ML pipeline.
MLflow: The Modular Alternative to Langsmith for Flexible AI Pipelines
With Langsmith, you have an all-in-one solution, but MLflow takes the opposite approach, allowing you to mix and match its services according to your needs. What makes MLflow stand out is that it’s entirely open-source and highly flexible.
You can use its tracking or model registry features while pairing it with other tools for different parts of your pipeline. This modularity is where MLflow shines, especially when dealing with unique setups that don’t follow a cookie-cutter workflow.
10. Kubeflow: The Gold Standard for Production-Grade Machine Learning
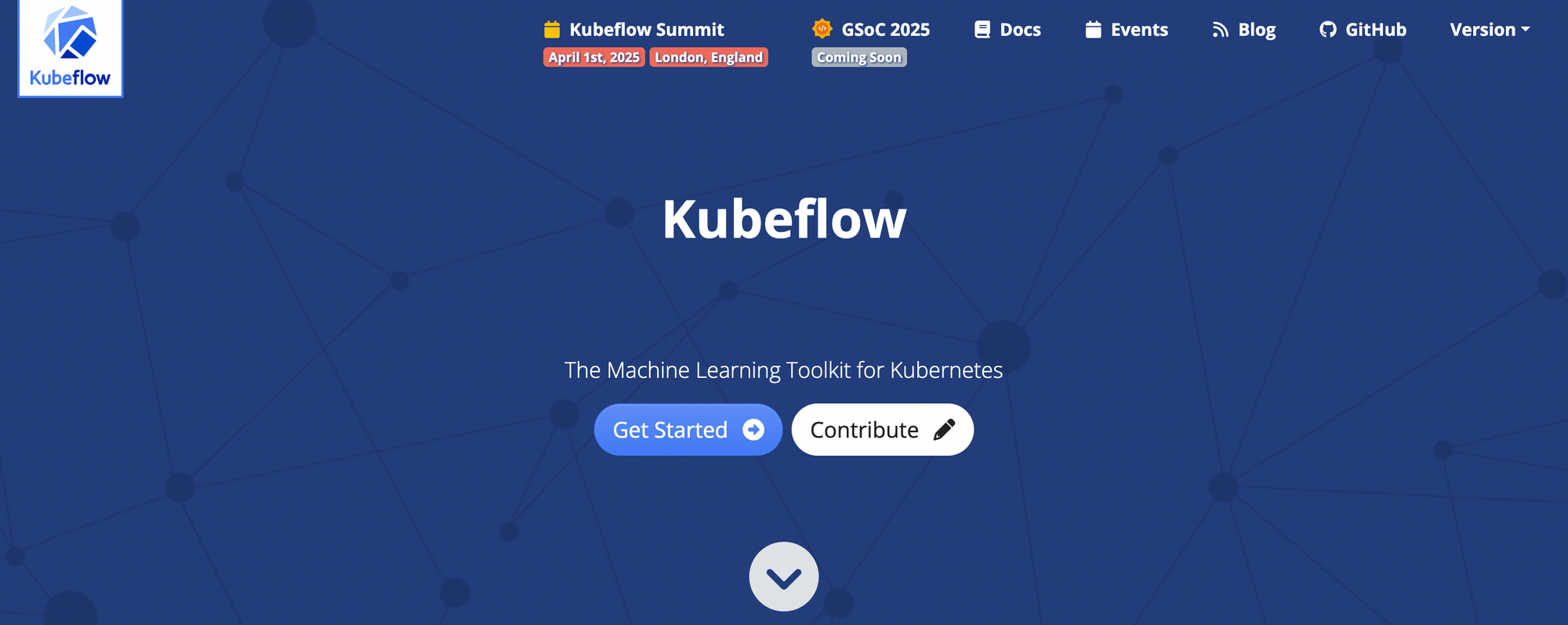
For those working with production-grade machine learning workflows, Kubeflow is the gold standard. Where Langsmith is more of a plug-and-play solution, Kubeflow is the entire machine learning lifecycle management platform. If you’re deploying at scale or dealing with hybrid cloud environments,
Kubeflow has the tools to orchestrate even the most complex ML systems. Here’s the deal: Kubeflow’s main strength is managing end-to-end ML workflows in production environments. It’s especially suited for enterprises that require a lot of customization and need the platform to handle everything—from data preparation to model serving—at scale.
11. Neptune.ai: A Collaboration-Focused LLM Observability Platform
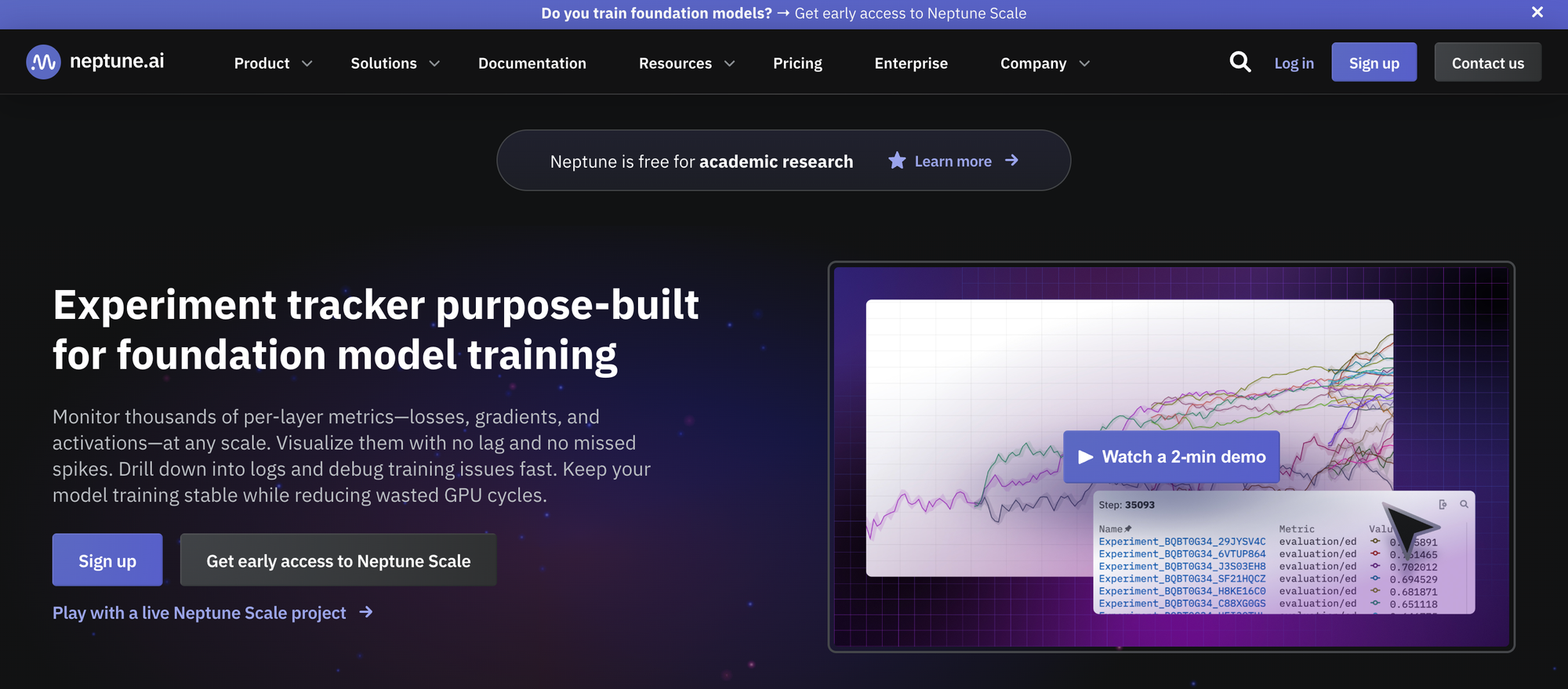
Neptune.ai is a strong alternative when collaboration is key. While Langsmith focuses more on workflows for data scientists and engineers, Neptune.ai strongly emphasizes model tracking and collaboration. It’s perfect for teams across a distributed team that need to:
- Share model metrics
- Training runs
- Visualizations
Why should I consider Neptune.ai? The reason is simple: If you’re managing a team or working remotely with multiple collaborators, Neptune.ai simplifies communication and collaboration. It allows team members to:
- Easily track experiments
- Visualize results
- Comment on specific runs or model versions
Related Reading
- What is Agentic AI
- How to Integrate AI Into an App
- Generative AI Tech Stack
- Application Integration Framework
- Mobile App Development Frameworks
- How to Build an AI app
- How to Build an AI Agent
- Crewai vs Autogen
- Types of AI Agents
What is Langsmith and What are its Strengths & Weaknesses?
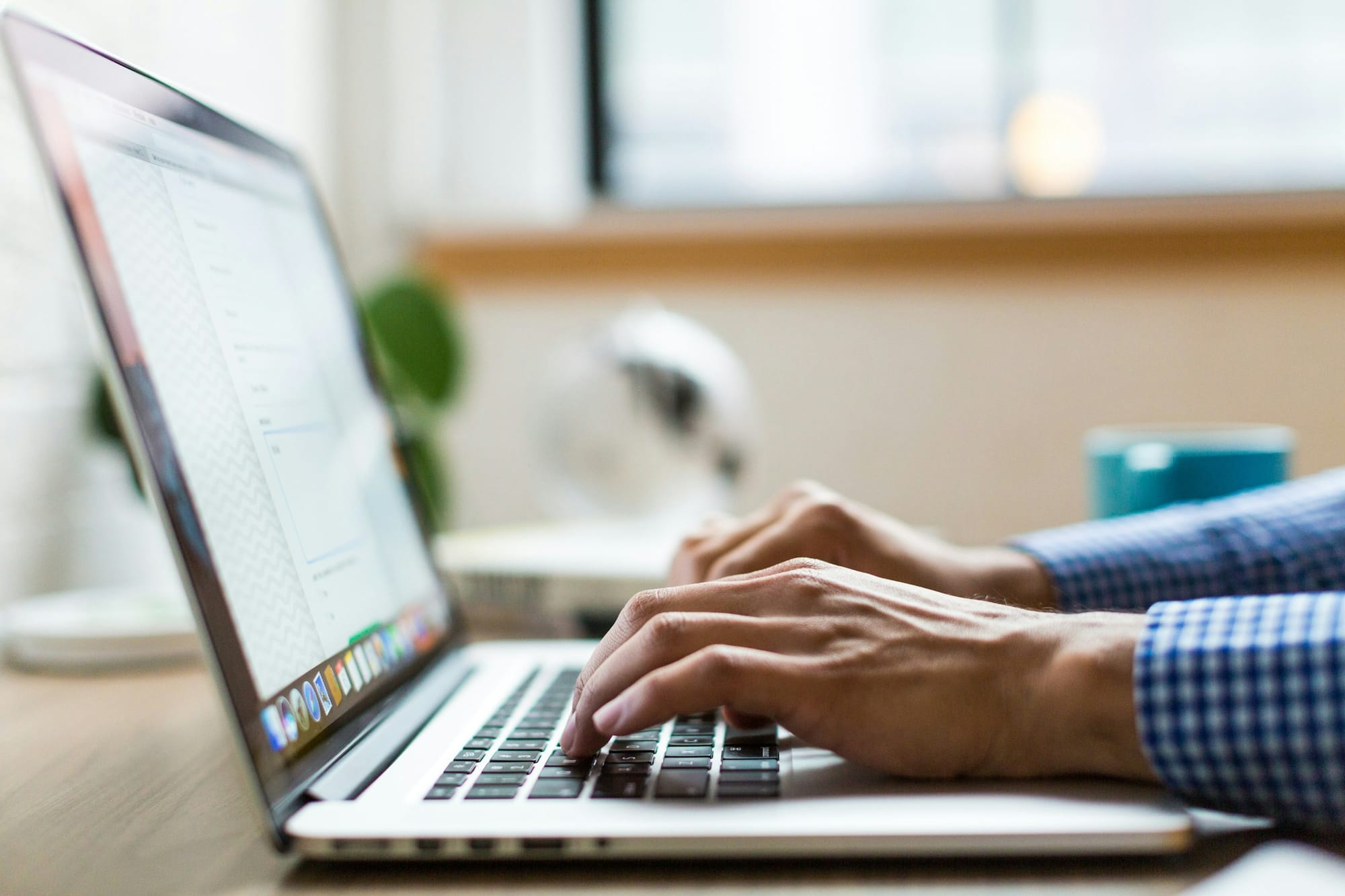
LangSmith is an advanced tool designed for LLM observability, helping developers monitor and improve the performance of language models (LLMs). This platform offers features like tracing, prompt versioning, and experimentation, enabling teams to fine-tune their AI models for optimal performance. As the demand for LLM applications continues to grow, LangSmith’s robust suite has made it a go-to for LLM monitoring.
LangSmith Strengths
LangSmith has many features that set it apart from other LLM observability tools. Its key strengths include:
Comprehensive Observability Features
LangSmith is packed with observability features to help teams efficiently monitor and improve the performance of their LLMs. These capabilities include:
- Prompt versioning
- Different types of traces
- Interactions
- Agent behavior tracking
- Visualizations
User-Friendly Interface
LangSmith has an intuitive interface that is easy for technical and non-technical users. The platform provides a clear overview of LLM performance and breaks down different traces for better understanding into digestible components that can be:
- Visualized
- Filtered
- Categorized
Integrations
LangSmith has native integrations with popular tools and frameworks, including:
- LangChain
- Hugging Face
- OpenAI
This allows teams to build LLMs using their preferred tools and seamlessly incorporate LangSmith to monitor and optimize performance.
LangSmith Weaknesses
LangSmith has some limitations that may drive potential users to look for alternatives.
LangSmith’s weaknesses include:
Pricing
LangSmith’s pricing is not publicly listed on its website and likely varies based on usage. Some users have reported that it can get expensive as usage increases. This can be a concern for teams operating on a tight budget.
Learning Curve for Advanced Features
While LangSmith is user-friendly, some of its advanced features may take time to learn, especially for users unfamiliar with LLMs.
Limited Customization
LangSmith is a proprietary tool, meaning customization is limited. This may be a drawback for teams that prefer open-source solutions.
How to Choose the Best Langsmith Alternatives
LangSmith is a good tool for LLM observability, but it’s not for everyone. Some users might seek LangSmith alternatives for various reasons, such as cost analysis, specific self-hosted deployment needs, or a more flexible pricing model.
With the rapid evolution of LLM observability tools, multiple solutions are now on the market that provide similar capabilities, often with unique features or better pricing structures.
Evaluating LangSmith Alternatives for LLM Development
When selecting the best LangSmith alternatives, it is essential to evaluate the tools based on several key criteria that align with your organization's needs and technical requirements. The right observability tool should offer comprehensive features supporting LLM performance monitoring and optimization.
Open-Source Availability and Alternatives
Choosing an open-source alternative is often a priority for teams that prefer to modify and customize their observability tools. Open-source platforms offer:
- Transparency
- Flexibility
- The ability to tailor the tool to specific needs
These platforms also benefit from community contributions, ensuring they evolve with industry trends.
It’s worth noting that excellent non-open-source alternatives provide robust features and flexibility without needing code customization. These tools often offer strong support and maintenance and additional enterprise-grade features that can be valuable for teams looking for comprehensive solutions with minimal setup and maintenance requirements.
Self-Hosting Capabilities
A key consideration for many organizations is the ability to self-host the observability tool. This option allows for greater control over data, security, and the customization of monitoring systems. Whether managing sensitive information or preferring on-premise solutions, self-hosting can offer peace of mind and enhanced flexibility.
Support for Prompt Templating and Agent Tracing
Effective prompt templating is crucial for maintaining consistency and streamlining workflows. Many LLM observability tools allow users to define reusable prompts that can be customized for different use cases.
Agent tracing also plays a vital role in understanding how an agent's behavior evolves during interactions with language models, helping developers track performance issues and refine agent design.
Experimentation and Evaluation Feature
Experimentation is critical in optimizing language models. Tools that support experiments and allow for the creation of prompt experiments provide a structured way to test different configurations and compare model outputs.
Features such as trace visualization make analyzing and debugging these experiments easier by giving developers clear insights into the flow of interactions and model outputs.
Cost Analysis Tools
Given that LangSmith pricing can be a concern for many users, tools that provide built-in cost analysis features are essential. These tools help track:
- Resource consumption
- Assess usage patterns
- Predict future costs based on model utilization
Understanding the financial impact of observability tools allows organizations to make data-driven decisions about scalability and budget allocation.
User and Feedback Tracking
Incorporating user tracking is another critical factor in evaluating observability tools. By collecting feedback and tracking user interactions with the language model, these tools enable teams to understand how models are performing in real-world scenarios.
Feedback can refine models and improve performance over time. Tools that support user tracking often include customizable custom properties for segmenting users based on behavior or other attributes.
Integration with Frameworks Like LangChain
Seamless LangChain integration and compatibility with other machine learning frameworks are essential for maximizing the utility of your observability tool. A tool that can easily integrate with existing infrastructure and other tools used in the machine learning lifecycle will save time and enhance collaboration.
Consider whether the tool can be incorporated into your existing machine learning observability stack without extensive customization.
Pricing Flexibility
Pricing flexibility is another vital consideration when choosing an observability tool. Whether you're looking for an open-source alternative or a commercial solution with various pricing tiers, assessing how pricing structures align with your team’s budget is essential.
Flexible pricing can make a significant difference for startups, small teams, and enterprises alike, as it provides scalability as usage increases.
Support for Various Data Types (e.g., Text, Images)
LLM applications are not limited to text-based models. Observability tools that support a variety of datasets, such as:
- Images
- Audio
- Text
It offers the flexibility to monitor and analyze multimodal AI models. This makes them highly valuable for teams working on diverse AI projects beyond traditional text generation and requiring handling different data types.
Dashboard and Data Export Functionalities
A comprehensive dashboard is essential for visualizing model performance, tracking metrics, and monitoring experiment progress. Effective dashboards provide both high-level overviews and granular insights into performance.
Exporting data in different formats, such as CSV or JSON, is crucial for analyzing performance offline or integrating with other systems for advanced analytics.
Related Reading
- Llamaindex vs Langchain
- LLM Agents
- LangChain vs LangSmith
- Crewai vs Langchain
- AutoGPT vs AutoGen
- AI Development Tools
- GPT vs LLM
- Rapid Application Development Tools
- LangChain vs RAG
Start Building GenAI Apps for Free Today with Our Managed Generative AI Tech Stack
Lamatic offers a managed Generative AI Tech Stack. Our solution provides:
- Managed GenAI Middleware
- Custom GenAI API (GraphQL)
- Low Code Agent Builder
- Automated GenAI Workflow (CI/CD)
- GenOps (DevOps for GenAI)
- Edge deployment via Cloudflare workers
- Integrated Vector Database (Weaviate)
Lamatic empowers teams to rapidly implement GenAI solutions without accruing tech debt. Our platform automates workflows and ensures production-grade deployment on edge, enabling fast, efficient GenAI integration for products needing swift AI capabilities. Start building GenAI apps for free today with our managed generative AI tech stack.
Related Reading
- Best No Code App Builders
- Langchain Alternatives
- Langflow vs Flowise
- Langgraph vs Langchain
- LLM vs Generative AI
- SLM vs LLM
- Autogen vs Langchain
- UiPath Competitors
- Haystack vs Langchain
- Semantic Kernel vs Langchain