The generative AI tech stack has many moving parts, and understanding how they differ can help you clearly understand each technology's key differences, applications, and advantages. This will enable you to decide which AI solution best suits your needs or goals. Lamatic’s managed generative AI tech stack can help you achieve your objectives by clearly understanding the differences between foundation models and specialized generative AI tools.
Ultimate Gen AI vs AI Comparison Guide
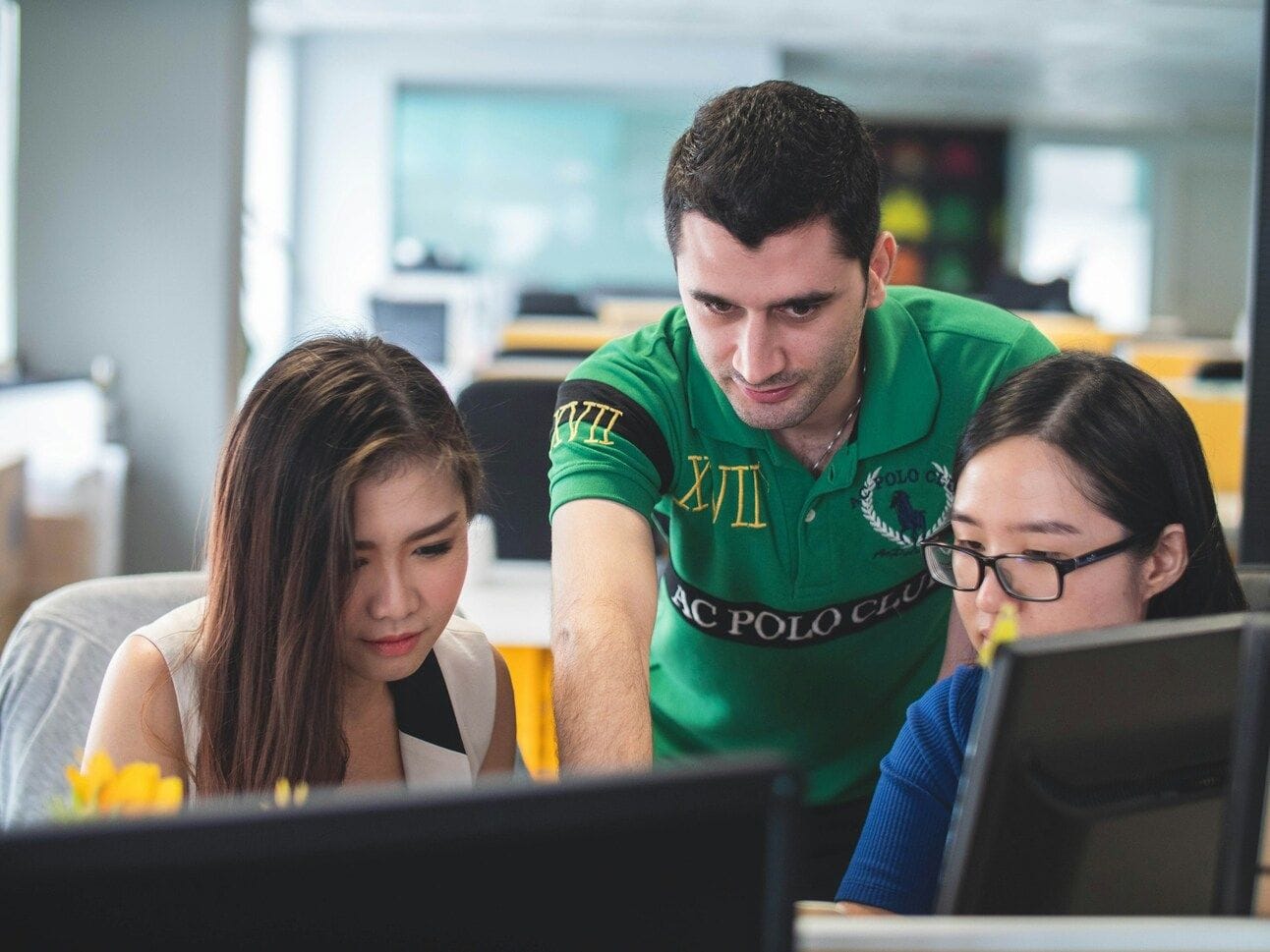
Artificial Intelligence (AI) is a branch of computer science dedicated to creating machines capable of intelligent behavior. It’s the science of designing smart algorithms and systems that can perform tasks typically requiring human intelligence. This includes:
- Problem-solving
- Recognizing speech
- Translating languages
- Decision-making
AI ranges from your smartphone’s voice assistant to complex algorithms that drive decision-making in finance and healthcare.
What is Generative AI (Gen AI)?
Generative AI (Gen AI) represents the next step in the evolution of AI. It’s a subset of AI focused on creating new and original content. Unlike traditional AI systems, which operate based on pre-existing data and rules, Generative AI generates new data and ideas, from digital art to novel text compositions. It uses advanced machine learning techniques, like deep learning, to analyze and produce creative and contextually relevant content.
AI’s Journey to Gen AI
The transition from AI to Generative AI is a key milestone in the history of technology. AI’s roots trace back to the 1950s, with the development of simple neural networks and the Turing Test, designed to evaluate a machine’s ability to exhibit intelligent behavior. It wasn’t until the rise of big data and advanced computational power in the 21st century that AI began to flourish. Significant developments include:
Deep Blue and Chess
In 1997, IBM’s Deep Blue became the first computer system to defeat the reigning world chess champion, Garry Kasparov. This feat showcased the potential of AI in complex problem-solving.
Deep Learning Revolution
The 2010s saw a surge in deep learning, a subset of machine learning based on artificial neural networks. This transformed AI’s capabilities, especially in image and speech recognition.
Rise of Generative Models
The mid-2010s witnessed the emergence of generative AI models like GANs (Generative Adversarial Networks), which could create realistic images and artworks.
Language Processing Breakthroughs
Tools like OpenAI’s GPT-3, introduced in 2020, marked a significant leap in natural language processing and generation, offering capabilities from writing essays to coding.
These milestones illustrate AI’s transformation from a rule-based system to an adaptive, creative force. Today, Generative AI is not just a tool for automation but a catalyst for innovation, reshaping industries from entertainment to healthcare.
Generative AI vs Traditional AI: Technology and Algorithms
Now that we’ve covered the foundations, it’s time to discuss the difference between AI and Gen AI regarding their technological frameworks and algorithms. To fully grasp Gen AI, one must first understand how AI works. This foundational knowledge not only illuminates the intricacies of AI but also helps in understanding the complex technology of Gen AI.
Traditional AI Focuses
AI’s functionality is built on a foundation of diverse algorithms, each crafted to execute distinct tasks effectively. Traditional AI systems often employ decision trees that mirror human decision-making, showcasing how AI can replicate our logical processes. These systems excel in identifying underlying patterns in data, a cornerstone in AI’s ability to simulate human-like decision-making.
Machine Learning: A Core Component
A substantial portion of AI’s prowess lies in machine learning algorithms. Using training data, these algorithms are trained to recognize patterns and make data-driven decisions. Techniques like logistic regression analysis and support vector machines are integral in this sphere, allowing AI to learn from and adapt to new data. Labeled data in supervised learning scenarios is a classic example where AI is trained to identify and categorize data based on predefined labels.
Neural Networks: Advanced Pattern Recognition
Neural networks represent an advanced tier of AI that is crucial in fields like. These structures mimic the human brain’s ability to recognize and interpret complex patterns. They play a vital role in processing extensive sensory data, such as image and speech recognition. Neural networks’ ability to process and analyze large datasets makes them invaluable in extracting meaningful insights from intricate data structures.
Generative AI Algorithms
Generative AI stands apart from traditional AI, including forms of weak AI designed for narrow, specific tasks. Gen AI focuses on a broader and more dynamic range of capabilities. It transcends simple data processing, venturing into the creation of novel and original content. This shift from mere data interpretation to content creation marks a significant evolution in the AI landscape.
Deep Learning: The Backbone of Gen AI
Deep learning plays a critical role in Gen AI. As an advanced subset of machine learning, it employs multi-layered neural networks that can process and learn from vast amounts of unstructured data, such as texts and images. This capability is central to Gen AI’s ability to analyze and generate complex data patterns, making it a powerful tool in data science and content creation.
Generative Models: GANs and VAEs
At the forefront of Gen AI are generative models like Generative Adversarial Networks (GANs) and Variational Autoencoders (VAEs). GANs, for example, use two ANNs in a generative-discriminative interplay, significantly expanding the possibilities of what AI can achieve. This unique mechanism allows GANs to generate highly refined and sophisticated outputs, a process that is central to the innovative nature of Gen AI.
Contrasting the Technological Frameworks of AI and Gen AI
The key distinction between AI and Gen AI lies in the complexity and objectives of their algorithms.
AI’s Structured Approach
Traditional AI excels in analysis, decision-making, and predictive modeling, thriving in environments where objectives and parameters are clearly defined. This structured approach is evident in applications ranging from data analysis to complex automated systems.
Gen AI’s Creative Frontier
In contrast, Generative AI focuses on more dynamic and creative domains. It is designed to analyze existing data and use it as a foundation for generating new, innovative outputs. This requires more adaptability and advanced learning capabilities, setting Gen AI apart as a tool for innovation and creation.
AI and Generative AI Use Cases
Understanding the practical applications of AI and discovering Generative AI use cases helps illustrate their transformative impacts, demonstrating how they solve real-world problems across various domains.
Traditional AI Use Cases
- Predictive Analytics: AI is extensively used in industries like manufacturing and transportation for predictive analytics. It analyzes data from machinery to predict potential failures, facilitating proactive maintenance and minimizing downtime.
- Fraud Detection: In the finance sector, AI algorithms are pivotal in detecting fraud. By scrutinizing transaction patterns, these systems identify patterns indicative of fraud, thus enhancing security and protecting assets.
- Personalized Recommendations: AI is a game-changer in e-commerce and streaming services, offering customized recommendations. It can analyze user behavior data and craft tailored suggestions, enriching customer experience and engagement.
- Business Process Automation: AI is revolutionizing business processes by automating tasks like document processing and data entry, increasing efficiency and allowing human employees to focus on complex tasks.
Generative AI Use Cases
- Automated Content Creation: Generative AI tools are employed in media and advertising for automated content generation, from news to marketing copy, streamlining content creation.
- AI-Generated Art: Generative AI excels in digital art and music. Learning from existing content, these tools can produce unique artworks and musical compositions, marking a new phase in creative AI.
- Synthetic Data Generation: A key role of Gen AI is in generating synthetic data for training machine learning models, especially valuable in scenarios where real-world data is limited or privacy-sensitive.
- Automated Content Moderation: Generative AI models are increasingly used for moderating content on social media and digital platforms, analyzing vast user-generated content to filter inappropriate or harmful material.
Incorporating traditional AI or advanced Generative AI across different sectors illustrates their transformative capabilities, from the Google Assistant in our daily lives to advanced analytics in industry. These technologies are redefining the boundaries of user interaction, creative expression, and business efficiency.
Impact of AI and Generative AI on Various Industries
The revolution brought about by Artificial Intelligence and Generative AI is fundamentally altering operations, strategies, and consumer interactions across various industries.
McKinsey’s research underscores these technologies as major economic drivers, potentially adding trillions to the global economy. Deloitte’s report shows that 42% of companies are experimenting with Gen AI, with 15% actively incorporating it into their strategies.
Another Salesforce survey reveals that 70% of Gen Z engages with Gen AI applications, indicating a generational shift in technology use. This widespread adoption across industries and demographics highlights the transformative power of Artificial intelligence.
Here’s how AI and Generative AI are reshaping key sectors, with notable examples of applications in each.
Banking and Finance
Due to Gen AI, McKinsey anticipates a potential annual value addition of $200 billion to $340 billion in banking.
Examples include AI solutions like:
- ZestFinance: uses AI for credit underwriting.
- Kasisto’s KAI: a conversational AI platform enhancing customer service in banking.
Gen AI is transforming the sector with innovations like Upstart, a lending platform using AI to streamline loan processing and risk assessment.
Retail and Consumer Goods
The potential impact of Gen AI in retail and consumer goods ranges from $400 billion to $660 billion annually. AI applications like Shopify’s AI-powered recommendation engine exemplify the growing trend of AI features in mobile apps, enhancing user engagement and personalization. Gen AI further elevates this sector with tools like Persado, which uses AI to generate optimized marketing language, enhancing customer engagement and sales.
Media, Technology, and Entertainment
In media and technology, AI is integral for content creation and audience engagement. Examples include Adobe Sensei, an AI and machine learning platform that powers creative tools. Gen AI integrates with platforms like Runway ML, enabling creators to generate unique visual effects and artworks, expanding creative possibilities.
Pharmaceuticals and Life Sciences
AI’s role in pharmaceuticals and life sciences is exemplified by platforms like Atomwise, which uses AI for drug discovery, and DeepMind’s AI solutions for protein folding problems. Generative AI accelerates these processes with applications like Insilico Medicine, specializes in AI-driven drug discovery and aging research.
Trends in AI and Gen AI
From the latest AI trends in mobile apps to the expansive capabilities of Generative AI, the evolving landscape of Artificial Intelligence is paving the way for innovative changes.
Generative Video and Multimodal Models
The leap from static images and texts to generative video is a milestone in Artificial Intelligence evolution. Tools like Deepfake technology and Adobe’s Project Aero, which allow for the creation of realistic video content top the list.
Multimodal models, such as OpenAI’s DALL-E, which can interpret and generate multi-format content, are also gaining prominence. This fusion of AI capabilities enhances user experience and is expected to revolutionize industries like advertising and entertainment, offering more immersive and interactive content.
The Generative Design Boom in Product Development
Generative design transforms fields such as engineering and architecture, particularly in CAD software like Autodesk Fusion 360. By inputting design goals and parameters, AI algorithms provide numerous innovative solutions, some of which may not be attainable through traditional methods. This cuts down development time and opens up a realm of creative possibilities, potentially reducing material costs and environmental impact.
Large Language Models (LLMs)
GPT-3 and similar models are revolutionizing how we interact with AI. They are not just about text generation but also about understanding context and nuances in human language. This technology finds applications in diverse fields, from creating educational content to assisting in legal document preparation.
Autonomous Agents
The development of autonomous agents promises a new era of AI applications. These agents, capable of making decisions and learning independently, are set to transform sectors like transportation (autonomous vehicles), healthcare (robotic surgery assistants), and even personal assistance (advanced AI assistants).
Open Models and Proprietary Models
The growing sophistication of open-source AI models is leveling the playing field. Tools like TensorFlow and PyTorch are making advanced AI technologies accessible to a broader audience, fostering innovation and creativity. This trend also influences cloud computing, with an increasing demand for cloud-based AI platforms and storage solutions to support these open-source models.
Related Reading
- How to Build AI
- GenAI Applications
- Generative AI Customer Experience
- Generative AI Automation
- Generative AI Risks
- How to Create an AI App
- AI Product Development
- GenAI Tools
- Enterprise Generative AI Tools
- Generative AI Development Services
Which Is Better, Generative AI or Artificial Intelligence?
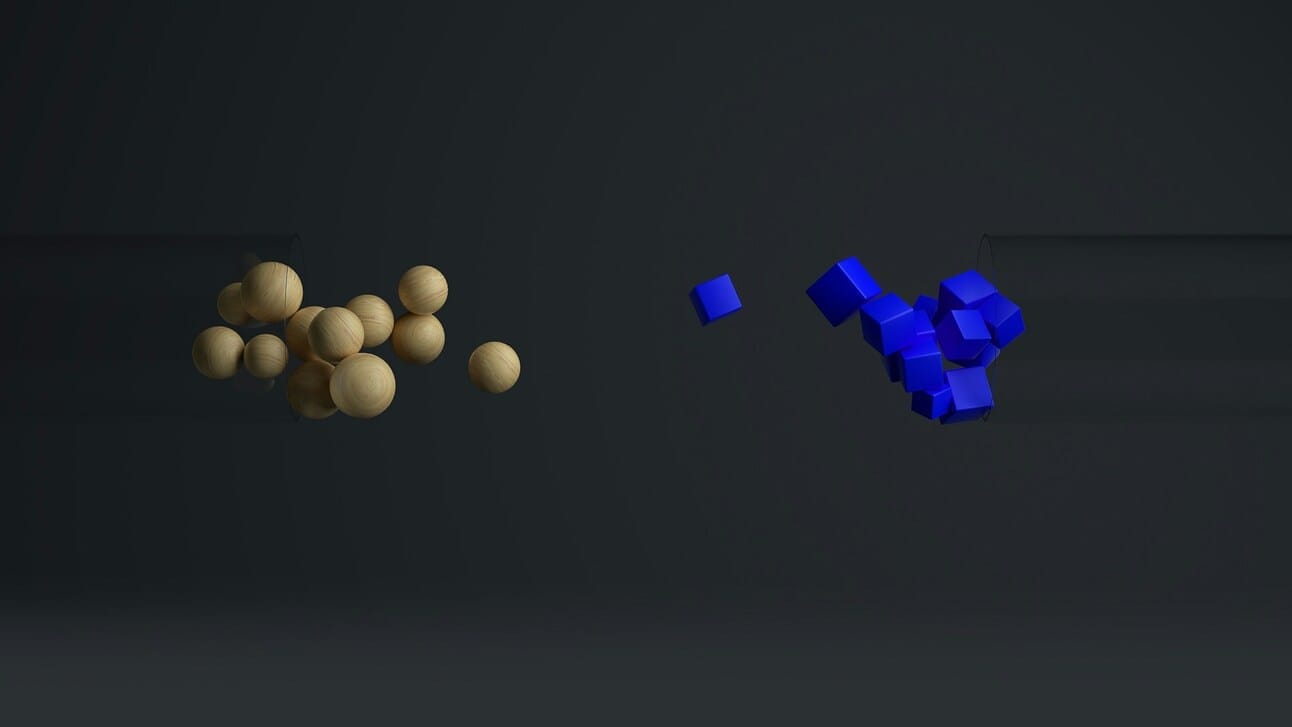
The choice between Generative AI and broader AI depends on the user's or industry's specific needs. Generative AI excels at producing creative content, such as:
- Generating text
- Images
- Code
Broader AI is used for various tasks, including decision-making, predictive analytics, and automation. Neither is inherently "better," but each is more suited to different tasks and industries.
Generative AI and Traditional AI are Not Competitors
Generative AI offers us a broader paintbrush, but its applications differ from traditional AI's. The former is used to create content that concerns many industries, including:
- Entertainment
- E-commerce
- Marketing
We also rely on traditional AI to perform specific analyses and predictions. Generative and traditional AI will likely complement one another in problem-solving, making predictions based on patterns, and generating original output based on those predictions.
Ethical Considerations Around AI and Gen AI
Transparency and Accountability: The Key to Understanding Gen AI
Transparency and accountability are among the foremost ethical considerations in AI and Gen AI. As AI systems, especially Gen AI, become more complex and widely used, it becomes crucial to understand how these systems arrive at certain decisions or outputs. The call for transparency is about making these processes clear to all stakeholders, ensuring that errors, biases, or potential misuses can be identified and addressed.
For example, the European Union’s AI Act mandates more substantial transparency obligations for high-risk AI systems, including Gen AI, requiring public information on training data and model registration.
Bias and Fairness: Can We Trust Gen AI?
Gen AI systems learn from large datasets, often reflecting societal biases. This can lead to AI models perpetuating or amplifying these biases, resulting in unfair or discriminatory outcomes. For instance, hiring algorithms might favor male candidates for technical positions due to historical data trends. Addressing these biases involves using diverse and representative datasets, identifying and correcting biased data points, and employing techniques like bias elimination and adversarial training.
Intellectual Property and Misinformation: Who Owns the Content Produced by Gen AI?
Generative AI poses unique challenges regarding intellectual property rights and spreading misinformation. As these systems can generate new content, questions arise about the ownership of this content and the potential for creating misleading or false information. Developing ethical guidelines and legal frameworks to address these issues is critical to harnessing Gen AI's positive potential while mitigating its risks.
Related Reading
- Gen AI Architecture
- Generative AI Implementation
- Gen AI Platforms
- Generative AI Challenges
- Generative AI Providers
- How to Train a Generative AI Model
- Generative AI Infrastructure
- AI Middleware
- Top AI Cloud Business Management Platform Tools
- AI Frameworks
- AI Tech Stack
Start Building GenAI Apps for Free Today with Our Managed Generative AI Tech Stack
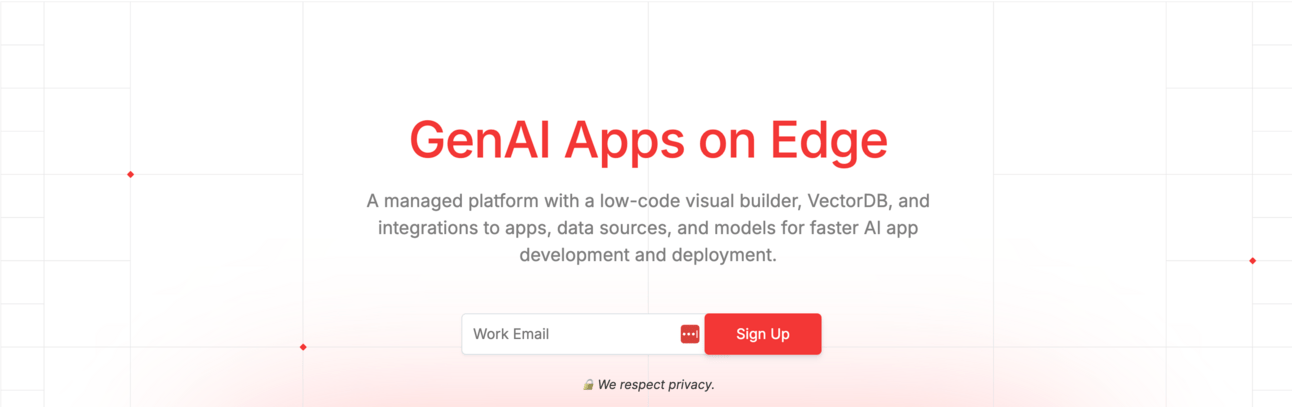
Lamatic offers a comprehensive managed generative AI tech stack. Our solution provides:
- Managed GenAI Middleware
- Custom GenAI API (GraphQL)
- Low Code Agent Builder
- Automated GenAI Workflow (CI/CD)
- GenOps (DevOps for GenAI)
- Edge deployment via Cloudflare workers
- Integrated Vector Database (Weaviate)
Lamatic empowers teams to rapidly implement GenAI solutions without accruing tech debt. Our platform automates workflows and ensures production-grade deployment on the edge, enabling fast, efficient GenAI integration for products needing swift AI capabilities.
Start building GenAI apps for free today with our managed generative AI tech stack.