AI can transform how businesses operate, unlocking new opportunities and boosting productivity. Yet, implementing AI solutions comes with significant costs. The average AI project takes 13 months to complete and costs $2.7 million. These figures likely vary based on the project's complexity, goals, and the organization's existing tech capabilities. Knowing how to budget for AI development costs can help organizations prepare for and succeed with their AI implementations. This article will explore the average costs of AI development and offer practical strategies for optimizing and managing AI development costs.
Lamatic’s generative AI tech stack can help readers achieve their objectives by providing clear insights and practical strategies for budgeting and optimizing AI development expenses.
How Much Does It Cost to Build an AI Solution in 2024?
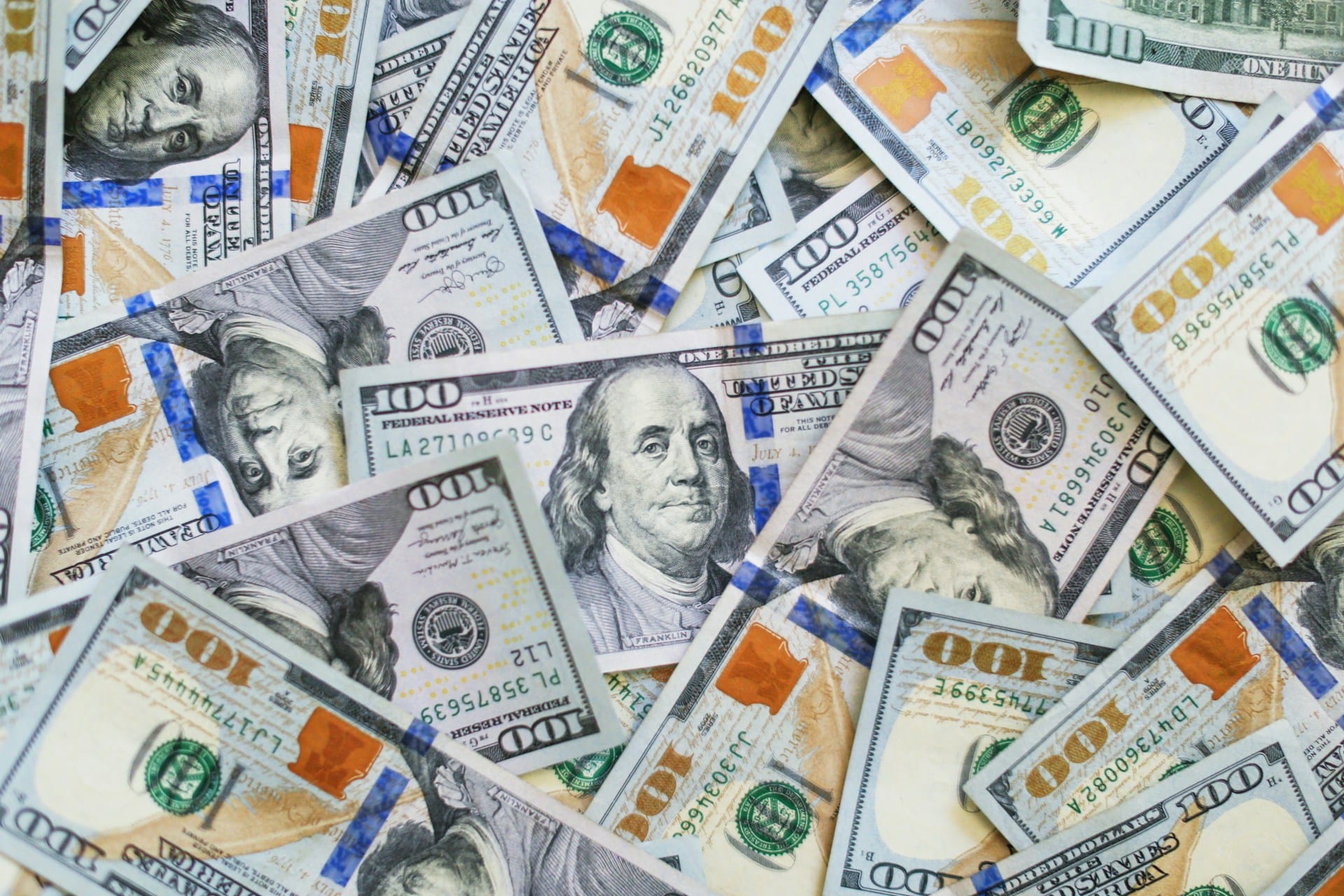
In 2024, the demand for artificial intelligence (AI) solutions continues to grow. Businesses use it to:
- Enhance efficiency
- Drive innovation
- Stay competitive
Not to mention the tons of AI startup ideas that are being brought to life every month and appearing on the market. AI is becoming essential, as it offers big benefits like:
- Boosting revenue
- Improving supply chains
- Simplifying hiring
- Personalizing customer experience
The Growing AI Market and Investment
Companies that adopt AI early will gain an edge as it spreads across industries. So it's no wonder global AI spending reached $154 billion in 2023, with banking leading at $20.6 billion and retail at $19.7 billion. The financial sector is expected to invest even more in AI between 2024 and 2027, while the AI technology market is projected to surpass $1.8 trillion by 2030.
The main challenge, especially for smaller businesses, is the cost of building an AI solution. It can vary widely based on factors like:
- Type of AI
- Project complexity
- Needed resources
Whether you're a small startup creating a simple chatbot or a large company developing an advanced machine learning model, AI can be a significant investment. Let's break down the cost of implementing artificial intelligence in 2024, what influences these costs, and tips on how businesses can manage their AI investment wisely.
Average Cost of AI Development
How much does it cost to make an AI? For more straightforward AI projects, expenses typically start at around $5,000. For more advanced, technology-intensive solutions, costs can exceed $500,000. So, the cost of developing an AI solution can vary based on the project's complexity and scope.
According to AI development projects reviewed on Clutch, most projects fall within the $10,000 to $49,000 range. This range usually covers developing basic AI tools like:
- Chatbots
- AI companions
- Simple machine-learning models
The Cost of AI Consulting Services
In addition to development costs, consulting services also contribute to the overall budget. These services are typically priced based on the consultant's expertise and hourly rate, ranging from $200 to $350 per hour. The average hourly cost for AI development typically ranges between $25 and $49.
Understanding the Components of AI Costs
AI solutions also come with various ongoing costs. So, it’s vital to understand what affects the price of artificial intelligence before starting a project. Here’s a breakdown of what to expect:
Hardware Costs
The preeminent cloud platforms generally used to deploy and manage AI systems are:
- AWS (Amazon Web Services)
- Microsoft Azure
- Google Cloud Platform
Let’s assess the key differences:
Cloud infrastructure from these popular 2024 vendors gives access to powerful resources:
- GPUs
- TPUs
- Other high-performance computing devices
All three major cloud service providers, AWS, Azure, and GCP, offer reliable services, making them the best options in the cloud computing market. The choice between these three depends on:
- Specific business needs
- Preferences
- Existing technology stacks
Incidentally, the cost of AI hardware has come down due to chip advances and increased manufacturing scale. Even small businesses and startups can now use these powerful GPUs, TPUs, and other resources to deploy their AI models more cheaply. The cost of cloud computing for AI is variable and depends on several factors, including:
- Equipment configuration
- Duration of use
- Availability of discounts or volume commitments
Hourly pricing for artificial intelligence GPU instances ranges from $0.50 to $10, while TPU usage costs range from $2 to $40 per hour.
Software Costs
The AI software landscape has evolved to become not only diverse, but also cost-effective. Initial investment requirements have significantly dropped, as many popular open-source frameworks have become available royalty-free. These include:
- TensorFlow
- PyTorch
- Keras
Organizations can still pay for commercial enterprise AI platforms, tools, and services to accelerate model development, deployment, and management. As for subscriptions, the typical AI software cost will depend on the functionality and support required. It ranges from $1,000 to $100,000 per month. Examples of such platforms:
Data Costs
High-quality, relevant datasets are imperative for AI, especially in specialized fields, where data acquisition often requires substantial investment. In 2024, AI data acquisition and preparation costs can range from:
- $10,000 for a small pilot project
- $1 million for a large-scale
AI Development and Implementation Costs
According to IDC, global AI spending is projected to reach $110 billion in 2024, reflecting a 28% increase from 2023. Major growth is anticipated in:
- Financial services
- Retail
- Healthcare
Development Costs
Developing AI-based solutions costs range from hundreds to tens of millions of dollars, depending on:
- Project scale and complexity
- Industry specifics and use cases
Factors Affecting AI Development Costs
Data collection and management: AI uses large data sets to make accurate predictions and decisions. This includes the cost of:
- Collecting data
- Storing data
- Processing data
Factors Influencing the Total Cost of AI
- User Interface (UI) and Experience (UX) Design: Creating intuitive and attractive user interfaces for AI applications is essential for adoption and user satisfaction.
- Algorithm Development and Training: AI requires significant investments in research, computing resources, and expertise.
- Deployment and Infrastructure: The cost of deploying AI solutions and the necessary infrastructure, such as cloud services or on-premises servers, can vary depending on the scope and complexity of the implementation.
- Regulatory Compliance: Ensuring AI applications comply with industry standards and privacy regulations increases costs.
- Scalability and Maintenance: Ongoing costs include scaling AI solutions to handle growing data and user loads and maintaining and updating systems to keep them efficient and up-to-date.
- Implementation Costs: Once an AI system is developed, additional costs are associated with implementing and deploying it. The cost of implementing and operating AI solutions can be 50-200% of the initial development costs, depending on:
- Scale
- Complexity
- Support requirements
What exactly does this include?
- Hardware and Infrastructure: Servers, storage, and networking equipment are needed to run the AI system.
- Integration: Integrate and deploy the AI system with existing systems and processes.
- Training and Change Management: Train end users and stakeholders on how to use the AI system effectively.
- Scalability and Maintenance: Ongoing costs include scaling AI solutions to handle growing data and user loads and mAI ntAI ning and updating systems to keep them efficient and up-to-date.
It’s unequivocally necessary to calculate the cost of AI development services as accurately as possible in advance so that you don't waste time searching for a development team and get frustrated when the project's total cost doesn't fit your budget.
Cost Breakdown By AI Solutions
Natural Language Processing (NLP) Costs
- Model development: $50,000 to $500,000, depending on complexity and scalability
- System integration: $10,000 to $100,000 for seamless compatibility with existing systems
- Monthly hosting and support: $1,000 to $10,000
Computer Vision (CV) Costs
- Model development: $100,000 to $1 million, depending on project complexity
- Hardware integration (cameras, sensors): $20,000 to $200,000
- Monthly hosting and support: $2,000 to $20,000
Predictive Model Costs
- Model development: $75,000 to $750,000, depending on complexity
- Business data integration: $25,000 to $250,000
- Monthly hosting and support: $5,000 to $50,000
Robotic Process Automation (RPA) Costs
- Bot implementation: $20,000 to $200,000, depending on scale
- Business application integration: $10,000 to $100,000
- Monthly licensing and support: $1,000 to $10,000
Cloud Vs. On-Premises AI Solutions
Incessantly, more companies are turning to cloud solutions to improve operational efficiency. They’re often used to improve and simplify their operations. Cloud solutions consist of software, applications, and tools stored on servers and accessed over the Internet. By storing data and running applications on servers or personal devices, users can access everything they need from anywhere they have an internet connection.
Here's what makes cloud solutions so attractive:
- Cost-Effective: With cloud services, you only pay for what you use, eliminating hardware and software costs. You don't need an IT team to maintain them.
- Scale on Demand: You can quickly scale your resources to meet the needs of your business. Whether you need to scale up or down.
- Improve Collaboration: Cloud applications are accessed over the Internet, making team collaboration seamless regardless of location.
- Automatic Updates: Providers update cloud solutions, so you always have the latest features and security updates without having to do anything.
Choosing between cloud and on-premises storage is an essential financial decision for businesses. Both options have advantages, but cost is often a significant consideration.
Let's compare the costs of cloud and on-premises solutions to see which one offers the best bang for the buck:
You can choose between cloud-based or on-premises AI solutions depending on your organization's needs. A thorough cost-benefit analysis will help you determine the best option.
Related Reading
- How to Build AI
- Gen AI vs AI
- GenAI Applications
- Generative AI Customer Experience
- Generative AI Automation
- Generative AI Risks
- AI Product Development
- GenAI Tools
- Enterprise Generative AI Tools
- Generative AI Development Services
Factors Influencing AI Development Cost
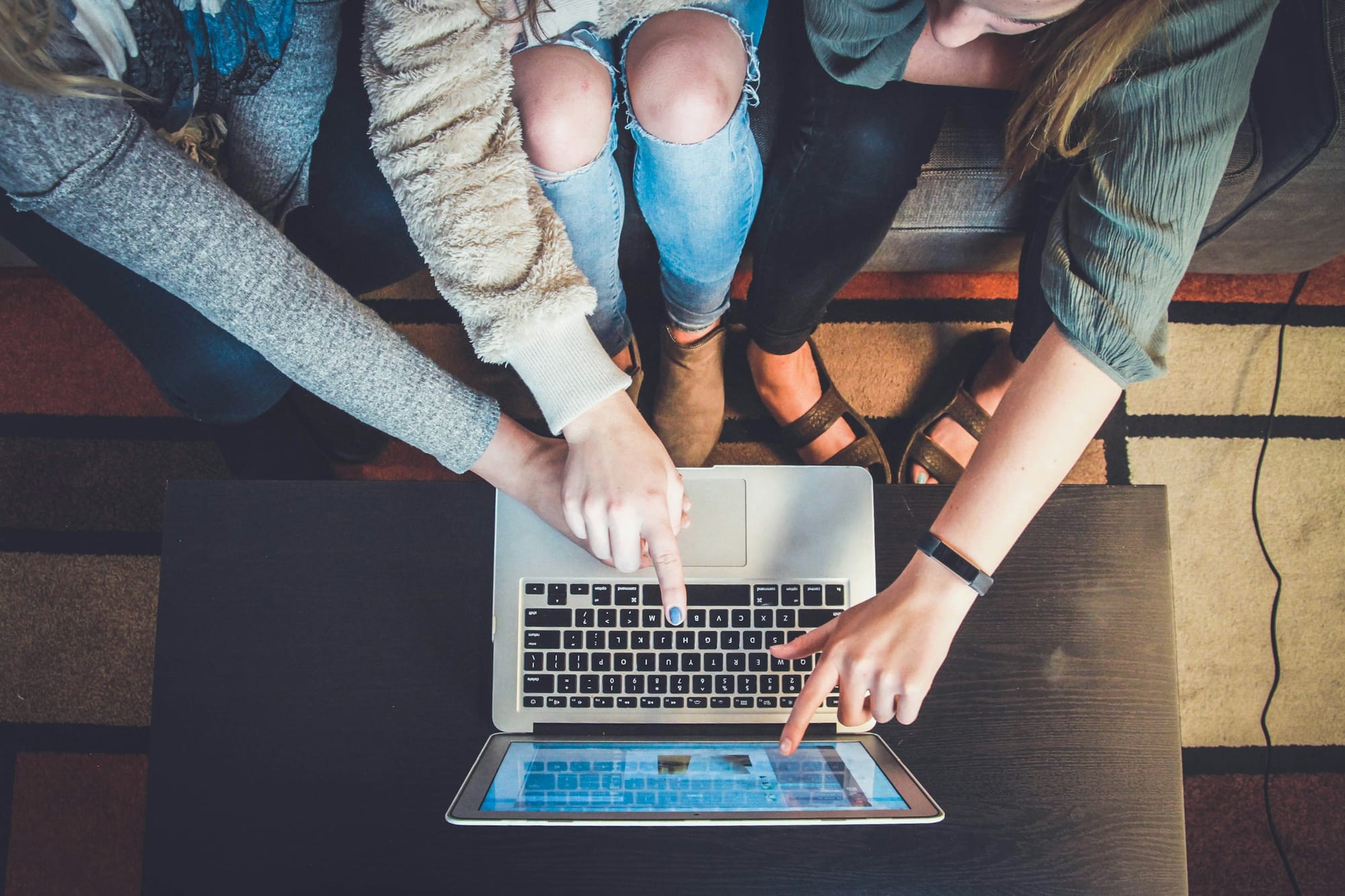
The Type of AI: Why the Kind of AI Solution You Choose Matters
The type of AI is one of the most significant determinants of cost. Different types of AI systems have varying complexities, resource needs, and development times, influencing the AI pricing and overall expense. Let's take a look at the most popular types of AI:
Rule-Based Systems
AI systems that operate based on predefined rules or logic. These systems follow "if-then" statements to respond to user inputs and do not adapt or learn from new data.
- Example: Building an basic AI chatbot that provides scripted responses based on user queries.
- Cost: It typically ranges from $10,000. This lower cost is due to the system's simplicity, which requires minimal data and processing power.
Machine Learning (ML) Models
These AI systems use algorithms to analyze data, learn patterns, and make predictions or decisions without explicit programming for each scenario.
- Example: An email spam filter that learns from examples of spam and non-spam emails.
- Cost: Development costs range from $50,000.
Deep Learning (DL) and Neural Networks
Deep learning is a type of machine learning that uses multi-layered neural networks to understand complex patterns in data. It's especially good at handling tasks that involve a lot of data and complex structures, like recognizing images and speech.
- Example: A facial recognition system that identifies individuals in real time.
- Cost: These systems can cost anywhere from $100,000. The high AI cost is due to the requirement for large datasets, advanced hardware like GPUs, and extensive training periods.
Natural Language Processing (NLP) and Computer Vision
NLP is an AI that enables computers to understand and generate human language. While computer vision allows machines to interpret and analyze visual data.
- Example: An NLP-based virtual assistant like Siri or Alexa.
- Cost: The cost of artificial intelligence solutions of this type typically ranges from $150,000.
These systems require complex algorithms and vast amounts of data, increasing development time and resource needs.
Project Complexity: How In-Depth a Project Are You Planning to Build?
How much does artificial intelligence cost if you're aiming for something more intricate? Note that the complexity of an AI project significantly affects its costs, too. As AI systems advance from basic to sophisticated solutions, development and maintenance expenses rise. Here's a quick comparison:
Aspect
- Simple AI System: Personal Finance Chatbot
- Advanced AI System: Predictive Maintenance for Industrial Equipment
Complexity
- Low: Uses pre-built platforms like Google Dialogflow or IBM Watson Assistant
- High: Involves complex model training, extensive data collection, and advanced infrastructure
Costs
- Generally Lower: Dialogflow's free standard plan and IBM Watson Assistant’s free tier help keep AI costs manageable
- Higher: Includes expensive data collection, powerful computational resources, and scalable deployment systems
Infrastructure
- Basic, including integration with existing systems
- Advanced infrastructure is required, affecting the cost of AI
Maintenance
- Minimal ongoing maintenance
- Ongoing costs for system monitoring and updates
The Type of Data and Dataset Size: Why AI Systems Need Data to Function
Data is the backbone of any AI system, and the dataset's type and size influence the artificial intelligence cost for development as well. Here's how:
Data Collection and Preparation
- Example: Collecting and cleaning customer transaction data for an AI-driven fraud detection system.
- Cost: This phase can be resource-intensive, with costs ranging from $10,000 depending on data complexity and volume.
Efficient collection and preparation are a must for developing an effective AI model.
Dataset Size
- Example: Training an AI model on millions of labeled images for a computer vision application.
- Cost: Larger datasets drive up costs significantly, ranging from $50,000. This is due to increased storage needs, higher processing power, and longer training times.
The amount of data provided, like years of diverse stock market data versus a few days' worth, can affect the AI's performance and predictive accuracy.
Data Labeling
- Example: Labeling medical images for a diagnostic AI tool.
- Cost: Labeling costs can range from $30,000, particularly if domain-specific expertise is needed. Accurate labeling is essential for training high-quality models.
Data Security and Privacy
- Example: Implementing data encryption and access control for a financial AI application.
- Cost: Ensuring data security and privacy can add between $20,000 to the development cost. The amount depends on the required security measures and data sensitivity.
The effectiveness of an AI model is closely tied to the size and quality of its dataset. Larger datasets generally lead to better performance but imply higher costs. To manage these expenses, using open-source datasets (e.g., Google Cloud Public Datasets, Kaggle, AWS Registry of Open Data) can be a win. Pre-trained AI tools like ChatGPT or DALL-E 3 can foster the development process and reduce initial costs. These models are often ready for immediate use but can be fine-tuned with specific data to enhance results further.
Algorithm Accuracy and Fluency: How the Requirements of an AI System Affect Its Costs
The desired accuracy and fluency of an algorithm, real-time performance, and continuous learning requirements also substantially impact AI app development cost. As these requirements become more stringent, the complexity and expenditure associated with these systems increase.
First, Accuracy Requirements
Higher accuracy demands more advanced models, larger datasets, and extensive testing and tuning. How much does AI cost for higher accuracy? It influences the need for high-quality data and sophisticated techniques to reduce errors. For instance, developing AI for self-driving cars requires extremely high accuracy to ensure safety. This involves using complex models such as deep neural networks trained on vast amounts of sensor data.
Testing these models in various scenarios adds substantial costs. For example, Waymo (a Google subsidiary) reportedly spent over $2 billion on R&D to achieve high accuracy and safety.
Second, Real-Time Performance
Real-time performance requires optimization for speed and reliability. This typically involves:
- Specialized hardware
- Efficient algorithms
- Rigorous testing
All of them increase development and operational costs. For example, drones for real-time navigation and object avoidance need fast, reliable AI algorithms. Ensuring that these algorithms perform well in real-time conditions requires powerful processors and real-time data processing capabilities, leading to higher development and deployment costs.
Third, Continuous Learning and Improvement
AI systems continuously learning from new data require ongoing updates and infrastructure to support this learning. This adds complexity to both the algorithm and the supporting systems and increases overall costs.
The High Cost of Continuous AI Improvement
Imagine streaming services like Netflix or YouTube. They continuously use AI to refine their recommendation algorithms based on user interactions. Implementing such systems involves maintaining large-scale infrastructure for:
- Data collection
- Model retraining
- Deployment
Due to the need for constant updates and scalability, the cost of these systems can exceed millions of dollars annually.
Infrastructure and Development Tools: Why the Tools You Choose Matter
How much does it cost to develop artificial intelligence based on your choice of tools? The selected infrastructure and tools can also drive up the cost of AI development.
Computing Power
Training complex AI models, like those used for deep learning, requires powerful hardware. For instance, using GPUs from AWS or Google Cloud services can be expensive. Alternatively, investing in on-premises hardware, such as NVIDIA A100 GPUs, involves a big upfront cost but may be cheaper in the long run.
Development Tools and Frameworks
Tools like TensorFlow and PyTorch are free and open-source, but skilled staff may be needed to use them effectively. Proprietary tools, such as IBM Watson or Microsoft Azure, offer more features and support but come with higher costs.
Testing and Validation
Testing AI systems thoroughly is crucial. For example, developing self-driving cars requires detailed simulations, which can be very costly. Similarly, financial AI models need to be stress-tested against market conditions, which involve significant expense.
Deployment and Maintenance
Deploying and maintaining AI solutions after development also incurs costs. For instance, scaling a chatbot or recommendation system requires ongoing monitoring and updates, adding to the overall expense.
Costs of Building Custom AI vs. Pre-Built or Ready-Made AI Solutions
The good news: you don't need to create things from scratch each time. There are pre-built options for everyday tasks and operations. They can be a great option if you don't need an innovative solution and seek to improve the workflows.
Pre-Built or Ready-Made AI Solutions
Ready-made AI solutions are pre-built tools and platforms designed to address common business needs. They are generally more affordable and quicker to deploy because they require minimal customization. They are ideal for businesses looking to integrate AI capabilities without the time and expense associated with building a system from scratch. The most popular ready-made AI solution for customer service is chatbots (in fact, up to 60% of users utilize chatbots on e-commerce sites). Ready-made chatbots are designed to handle customer inquiries, provide support, and automate responses to frequently asked questions.
How much does AI software cost in this case? These chatbots are relatively inexpensive, with costs typically ranging from $10,000 to $20,000, depending on the customization and integration required. The main spending for ready-made AI solutions:
- Initial Setup: The initial costs for setting up a ready-made AI solution are generally low, often ranging from $5,000.
- Ongoing Costs: These include subscription fees, licensing costs, and occasional upgrades, typically adding up to $1,000 to $10,000 annually, depending on the service and its usage.
- Support: It is important for ensuring smooth operation, effective troubleshooting, and adding changes. Typically, the costs for this stage start around $2,000.
The main advantages of ready-made AI solutions are their cost-effectiveness and ease of deployment. Nevertheless, when comparing custom vs. off-the-shelf development, the latter is limited in terms of flexibility and the ability to address unique or highly specific business challenges. It can also present scalability problems and introduce dependency risks.
Custom AI Development
Custom AI development means creating AI solutions designed specifically for a business's unique needs. While custom software development is generally more expensive than using off-the-shelf options, it offers more flexibility, scalability, and better alignment with business goals.
Custom AI for Personalized Recommendations
For instance, we have personalized recommendation engines. An e-commerce platform that wants to offer highly customized product recommendations, such as Amazon's recommendation engine, would require a custom AI solution. Developing such a system could cost up to $100,000, depending on the algorithms' complexity, the data sets' size, and the level of personalization required.
Advanced NLP for Virtual Assistants
Another example is natural language processing (NLP) for virtual assistants. A business developing a virtual assistant like Apple's Siri or Google Assistant would need a highly customized NLP solution.
The cost of developing such an AI system could range from $150,000 to more, depending on the desired accuracy, fluency, and ability to understand and respond to a wide range of human languages and contexts. The main spending for custom AI solutions:
- Development Team: Developing custom AI typically requires a team of data scientists, machine learning engineers, software developers, and domain experts. Salaries for these roles can range from $80,000 to $200,000 per year per person.
- Data Acquisition and Preparation: High-quality data is crucial for training AI models. Depending on the type of data required, the costs for data acquisition, cleaning, and labeling range from $10,000 to $20,000.
- Algorithm Development and Training: Developing custom algorithms is a time-intensive process that can involve multiple iterations. Costs for this stage can start from $20,000.
- Infrastructure Costs: Custom AI projects often require substantial computational resources, such as cloud services or high-performance computing hardware. Infrastructure costs could be $5,000 and up depending on the project size.
- Testing and Validation: Ensuring the AI system works as intended involves rigorous testing and validation. Count on around $15,000 or more.
- Maintenance and Support: Custom AI systems require regular updates, performance monitoring, and maintenance, which can add more than $5,000 per year.
Custom AI development is ideal for businesses with unique challenges or those looking to gain a competitive edge through innovative, AI-driven solutions. Still, the costs are higher due to the:
- Need for specialized expertise
- Extensive development time
- Ongoing maintenance and updates
Balancing Cost and Customization
The AI solution you choose, ready-made or custom-built, will have a big impact on the cost of your AI project. Ready-made options are cheaper and faster to deploy for general needs, while custom AI offers the flexibility and precision needed for complex challenges. Businesses should consider their needs, goals, and budget carefully to decide which approach is best for their AI investment.
In-House vs. Outsourcing AI Development: Which Option Will You Choose?
Choosing between in-house and outsourced AI development is also crucial. What kind of development will be cheaper, in-house or with a third-party provider? In-house development gives more control and customization but is more expensive and requires specialized skills.
Outsourcing can be cheaper and quicker, but with less control and potential risks to intellectual property.
See the table to get ready for the in-house vs. outsourcing decision regarding the more optimal cost of implementing AI:
Aspect
- In-House AI Development
- Outsourcing AI Development
- Control and Customization
- Full control and customization
- Limited customization; vendor's solution
Alignment with Business Goals
- High alignment with internal goals
- Potential misalignment with vendor's approach
Initial Costs
- Higher: Investment in recruitment, talent, and infrastructure Lower: Generally lower upfront costs
Talent Acquisition
- Requires hiring specialized personnel
- Access to skills without long-term hiring
Infrastructure Investment
- Requires substantial hardware and cloud resources
- Provided by the vendor
Development Time
- Longer: Time-consuming to build and develop
- Shorter: Faster delivery from established vendors
Maintenance and Updates
- Ongoing internal costs and effort
- Handled by the vendor
Communication
- Easier within the organization
- Potential challenges with vendor communication
Vendor Dependency
None: Full control Dependent on vendor's reliability
Knowledge Retention and Intellectual Property Risks
- Internal Expertise & IP Ownership
- Builds internal expertise and ownership of intellectual property
- Lower risks due to full control over IP
- Vendor Expertise & Shared IP
- Expertise and IP with the vendor
- Higher risks are higher: shared IP risks
Flexibility
- Less flexible in scaling resources
- Easier to scale the team based on needs
Related Reading
- Gen AI Architecture
- Generative AI Implementation
- Gen AI Platforms
- Generative AI Challenges
- Generative AI Providers
- How to Train a Generative AI Model
- Generative AI Infrastructure
- AI Middleware
- Top AI Cloud Business Management Platform Tools
- AI Frameworks
- AI Tech Stack
7 Tips on Cutting the AI Development Cost
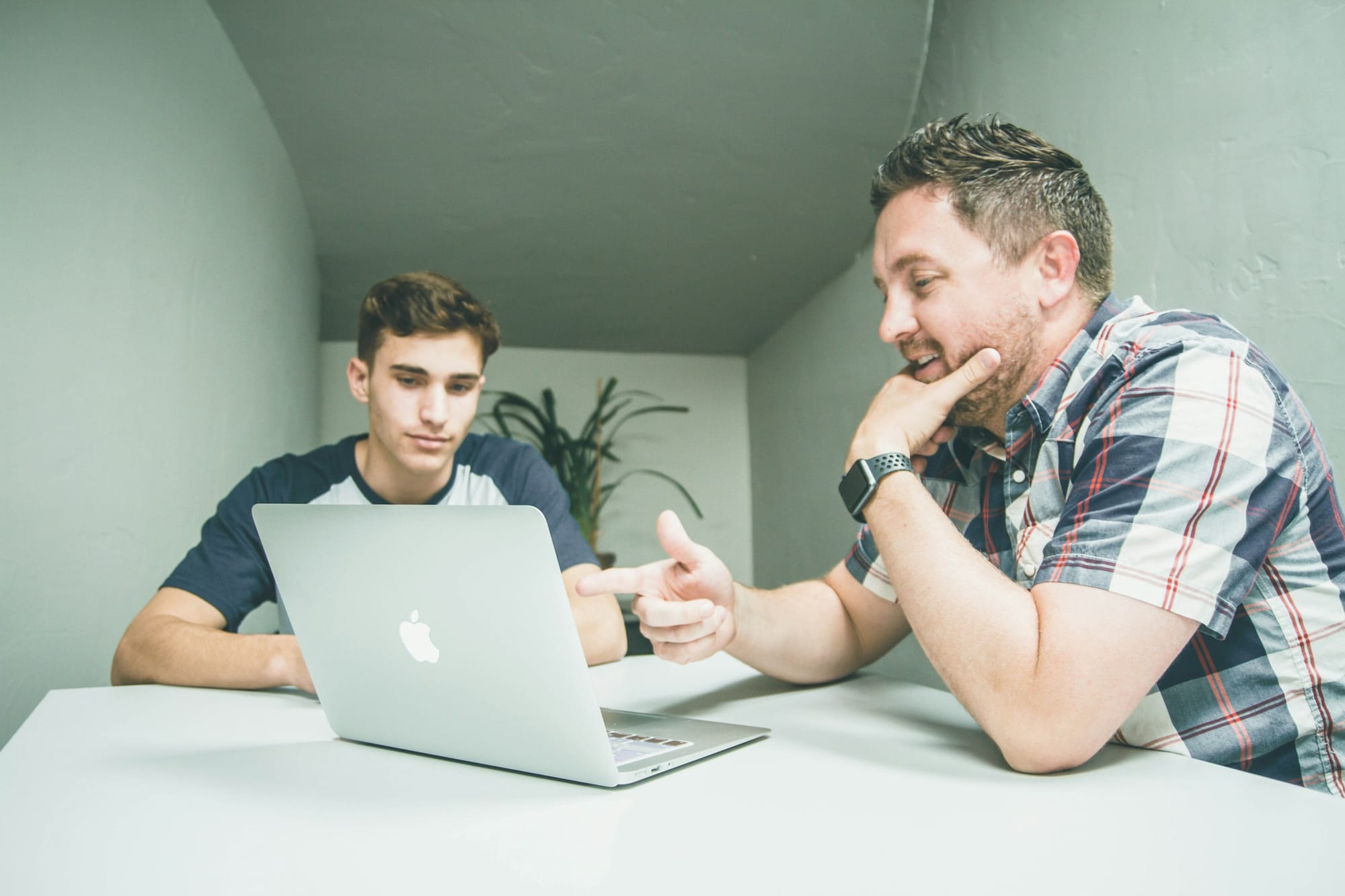
1. Adopt a Lean AI Development Approach
Start with a lean approach to minimize costs. Focus on high-value features that solve core business problems and begin with a Minimum Viable Product (MVP) that includes only these essential features. This allows you to build on a solid foundation without overspending early on.
2. Use Pre-Trained AI Models
To save money, leverage pre-trained models from libraries like TensorFlow Hub or OpenAI’s GPT. These models provide a starting point that you can fine-tune for specific needs, reducing the time and cost of training from scratch.
3. Consider Hiring Remote Developers
Hiring remote developers can reduce costs by tapping into a global talent pool where the cost of living may be lower. Platforms like Upwork make it easier to find and manage remote AI developers.
4. Implement Agile Methodologies
Using agile methodologies can enhance cost management and improve product quality. Practices like iterative development and continuous testing help catch issues early, reducing expensive rework later. Handling an AI project in smaller segments can improve cost estimation accuracy and allow for adjustments as the project progresses. This is especially useful in AI development, where challenges can be unpredictable.
5. Outsource Non-Core AI Development Tasks
Consider outsourcing routine tasks such as data labeling or maintenance to specialized providers. This approach can be more cost-effective than using your in-house team for these non-core functions. Find out more about outsourcing app development costs in a separate blog post.
6. Leverage Microservices and Reusable Components
Utilize modular, reusable components and microservices architecture to save on development time and costs. This approach allows each part of your project to be developed, tested, and deployed independently, enhancing overall efficiency.
7. Set Up a Robust CI/CD Pipeline
You can also opt for a Continuous Integration/Continuous Deployment (CI/CD) pipeline and significantly reduce costs by automating testing and deployment. This helps find bugs earlier and speeds up the release process, improving the agility and cost-effectiveness of your development efforts.
Start Building GenAI Apps for Free Today with Our Managed Generative AI Tech Stack
Building AI applications cost money. Lamatic’s generative AI tech stack minimizes the costs of AI development. With our managed middleware, custom APIs, and GenOps solutions, your team will easily avoid technical debt and rapidly build production-ready AI applications. Start building GenAI apps for free today with our managed generative AI tech stack.
Custom GenAI APIs, Low-Code Builders, and Automated Workflows
Lamatic’s generative AI tech stack accelerates development with intuitive tools that:
- Save time
- Reduce complexity
- Enhance collaboration
For instance, our custom GenAI APIs simplify integrations, while our low-code agent builder enables anyone to create AI applications without prior coding knowledge. With automated workflows, you can streamline your processes for continuous integration and deployment (CI/CD).
GenAI Development Without the Headaches
AI development can come with technical debt that creates headaches for your team. Lamatic’s generative AI tech stack helps you avoid these issues by providing production-ready solutions. This way, you can build AI applications without accruing technical debt that leads to more complex problems down the road.
Related Reading
- AI Application Development
- Best AI App Builder
- AI Development Platforms
- SageMaker Alternatives
- Gemini Alternatives
- LangChain Alternatives
- Flowise AI