Large language models are powerful tools that can transform products and services across industries. However, customizing a model to suit your unique business needs can feel overwhelming. What if you could build a model that seamlessly integrated into your product to enhance functionality, optimize performance, and deliver measurable business value? This guide on how to build your own multimodal LLM offers actionable insights on developing and scaling a custom large language model so you can achieve your goals while minimizing risks and resource usage.
Lamatic’s generative AI tech stack makes it easy to build, customize, and deploy large language models tailored to your precise business needs. With Lamatic, you can efficiently develop a model that enhances your product and delivers real value to your organization and users.
To Build or Not to Build Your Own LLM?
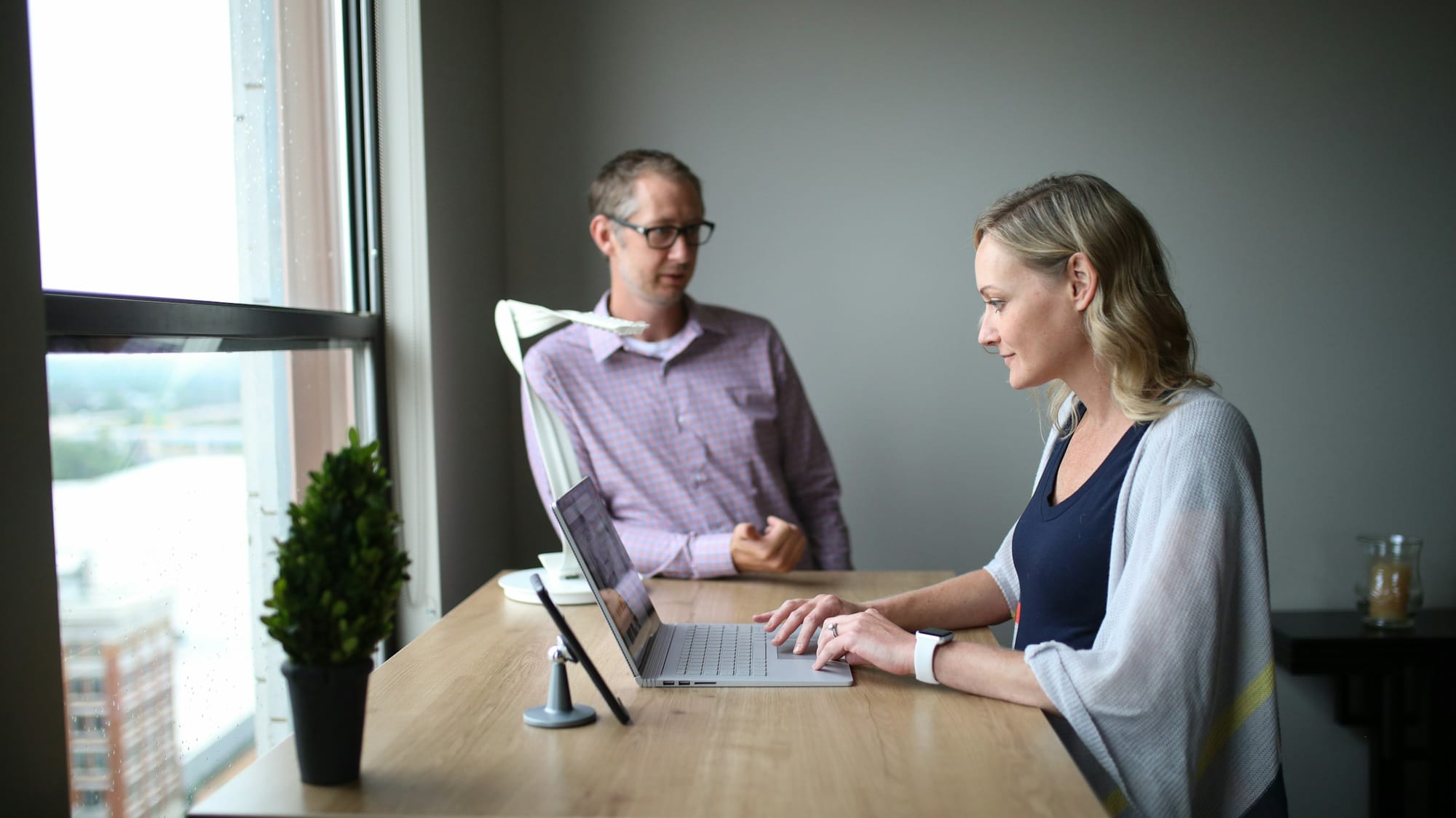
Every organization has unique needs, goals, and constraints. By developing its own large language model (LLM), an organization can create a custom solution that meets its specific requirements. Off-the-shelf models are one-size-fits-all, which is fine if a company doesn’t do anything niche or disruptive.
Tailoring LLMs to Your Business Needs
In today’s business climate, niche and disruptive are competitive differentiators. Third-party LLMs may not deliver the hoped-for performance. Building an LLM lets organizations custom-fit the solution to their needs, ensuring they understand the unique context and language of their industry.
Organizations can quickly tweak their models as market dynamics, customer behaviors, or business goals change. It’s harder to do when waiting for a third-party provider to release updates.
Control Over Data Privacy
While all companies dealing with data have privacy concerns and restrictions, some industries, like healthcare, have even greater regulations because they deal with sensitive data. Using a third-party LLM means jumping through hoops to ensure data privacy and introducing potential weak spots in security because of that same sharing.
Data Privacy and Security
Contracts certainly establish boundaries, but even then, they might be too risky. Building an in-house LLM lets these companies tightly grip their data. They can also more easily comply with strict regulations, like GDPR or HIPAA, when they control exactly where and how their data is handled.
Save Money By Building Your Own LLM
Even with advancements in the field, building an LLM is a significant investment. There are costs associated with research, development, and infrastructure. If a company needs to use the model a lot or has very specific requirements, this upfront investment can save money over time.
Licensing a third-party model continuously can get expensive, especially as usage grows. Companies that build also avoid dreaded vendor lock-in, which can come with:
- Surprise fees
- Rate hikes
- Changes in service
A custom LLM is one potential solution to this risk.
Optimize Business Performance
A custom build can really set a business apart from the competition. By training the model on proprietary data, companies create something unique—an AI that understands their customers, industry, and brand like no other. This can mean better, more relevant responses that boost customer satisfaction and loyalty.
Additional bonuses include:
- Intellectual Property: LLM ownership opens up new opportunities for licensing, patents, or even creating new products.
- Optimized Infrastructure: Think of the hardware and cloud environments. This gets the best performance possible without the limitations third-party LLMs sometimes carry.
- Full Transparency: In industries where decisions have significant implications, businesses need to understand exactly how their AI makes those decisions. Owning the model means full oversight, which builds trust and makes it easier to explain decisions to stakeholders.
Build to Future-Proof Your Business
The AI space changes quickly. Building an LLM could give companies more control to pivot without waiting for third-party providers to catch up. A custom model integrates more easily with existing tools, workflows, and processes. When those changes, the company could easily integrate the LLM with the change. It’s all about creating an AI product that works with the business and doesn’t demand the business shift components for the LLM.
Why Some Companies Might Not Build Their Own LLM
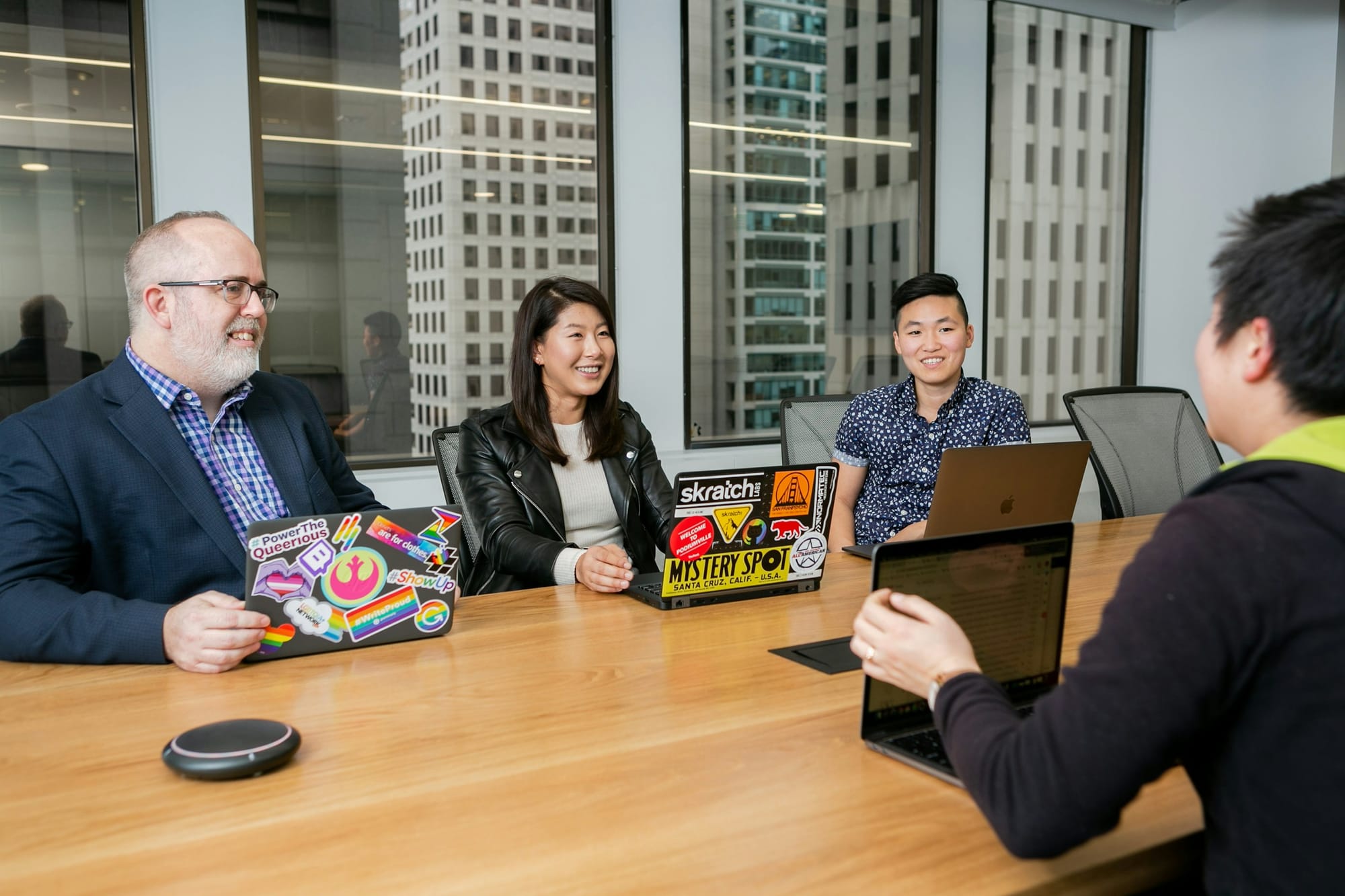
While the advantages of building an in-house LLM are clear, this path is unsuitable for every organization. Here’s why some companies might choose to stay with third-party solutions:
- Resource Limitations: Developing an LLM demands significant talent, technology, and capital investments. Companies lacking these resources or expertise may find the undertaking impractical. The high upfront costs and ongoing maintenance can be substantial barriers.
- Need for Rapid Deployment: If speed is of the essence, third-party LLMs offer a quicker route to deployment. Companies with immediate needs or short-term projects can benefit from the ready availability of third-party models, avoiding the lengthy development timeline associated with custom models.
- Low or Uncertain Usage: For organizations with low or uncertain demand for AI capabilities, the costs of building and maintaining an in-house model may outweigh the benefits. Third-party models provide a more flexible, cost-effective option, especially for infrequent or low-volume use.
- Non-Critical Applications: If the AI application is not mission-critical or requires only basic functionalities, third-party solutions may offer sufficient capabilities without the complexities of developing a custom model.
Weighing the Pros and Cons of Building Your Own LLM
Building an in-house LLM might have seemed like a moonshot a few years back, but today, it could be a strategic play for the right organizations. And the appeal is clear: more control over data, the ability to tailor every output, cost savings over time, and a unique competitive edge that future-proofs your business.
Related Reading
- LLM Security Risks
- What is an LLM Agent
- AI in Retail
- LLM Deployment
- How to Run LLM Locally
- How to Use LLM
- LLM Model Comparison
- AI-Powered Personalization
- How to Train Your Own LLM
How to Build Your Own LLM From Scratch
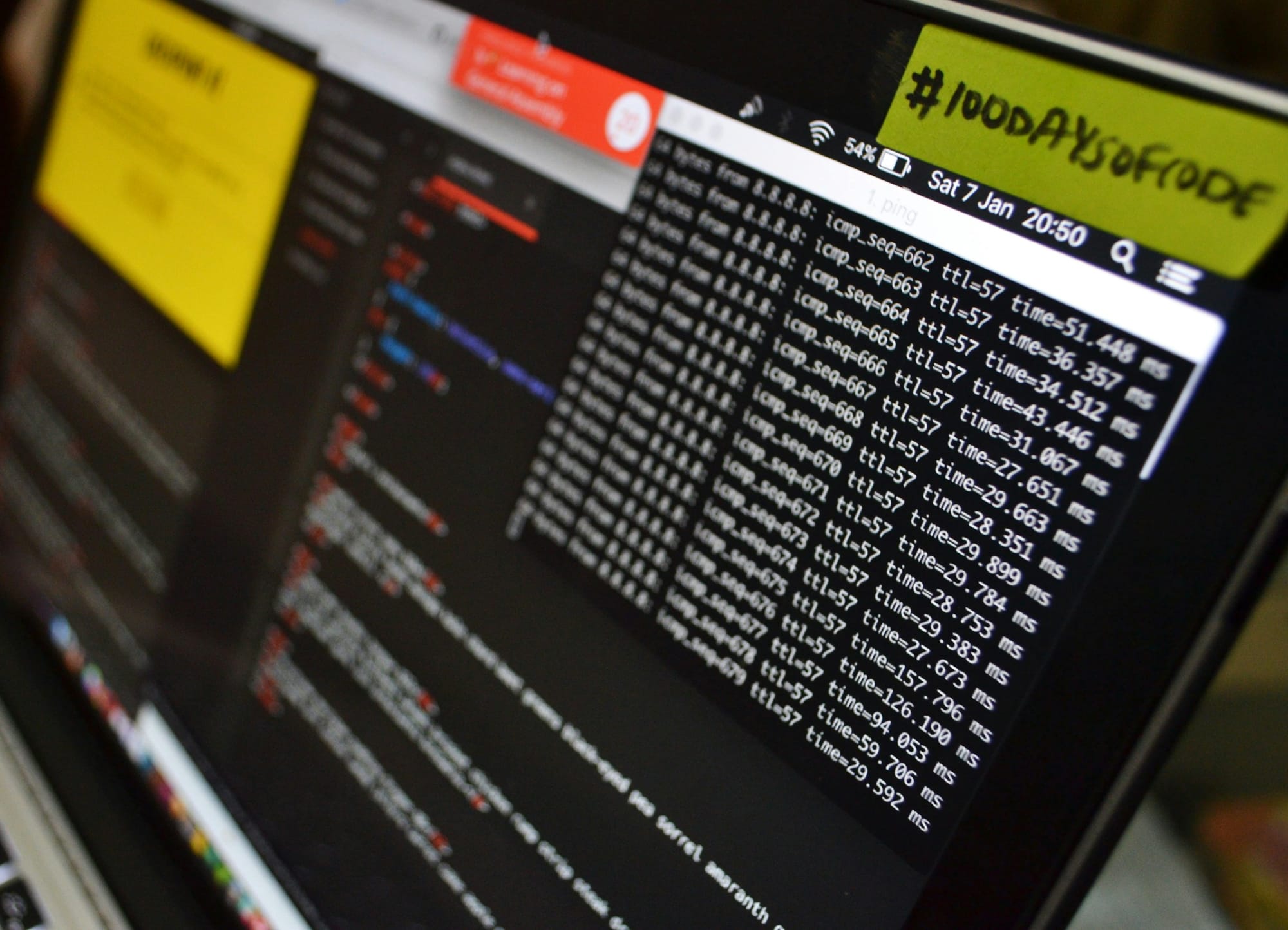
Define the Purpose of Your LLM
The first step in building a custom large language model (LLM) is defining its purpose. This is crucial for several reasons. First, it influences the size of the model. In general, the more complicated the use case, the more capable the required model—and the larger it needs to be, i.e., the more parameters it must have.
Resource Considerations
The more the number of parameters, the more training data you will need. The LLM’s intended use case also determines the type of training data you will need to curate. Once you have a better idea of how big your LLM needs to be, you will have more insight into the amount of computational resources required, i.e., memory, storage space, etc. In an ideal scenario, clearly defining your intended use case will determine why you need to build your own LLM from scratch rather than fine-tuning an existing base model.
Key reasons for creating your own LLM can include:
- Domain Specificity: Training your LLM with industry-specific data that aligns with your organization’s operations and workflow.
- Greater Data Security: Incorporating sensitive or proprietary information without fear of how it will be stored and used by an open-source or proprietary model.
- Ownership and Control: Retaining control over confidential data, you can improve your LLM over time—as your knowledge grows and your needs evolve.
Create Your Model Architecture
Having defined the use case for your LLM, the next stage is defining the architecture of its neural network. This is your model's heart, or engine, and will determine its capabilities and how well it performs at its intended task. The transformer architecture is the best choice for building LLMs because of its ability to:
- Capture underlying patterns and relationships from data
- Handle long-range dependencies in text
- Process input of variable lengths
Its self-attention mechanism allows it to process different parts of input in parallel, allowing it to utilize hardware, i.e., graphics processing units (GPUs), more efficiently than architectures that preceded it, e.g., recurrent neural networks (RNNs) and long short-term memory (LSTMs).
Using Transformer Architecture
The transformer has emerged as the current state-of-the-art neural network architecture and has been incorporated into leading LLMs since its introduction in 2017. Previously, an organization would have had to develop the components of a transformer on its own, which requires considerable time and specialized knowledge.
Today, there are frameworks specifically designed for neural network development that provide these components out of the box—Pytorch and TensorFlow are two of the most prominent.
Choosing a Deep Learning Framework
PyTorch is a deep learning framework developed by Meta renowned for its simplicity and flexibility, which make it ideal for prototyping. TensorFlow, created by Google, is a more comprehensive framework with an expansive ecosystem of libraries and tools that enable the production of scalable, production-ready machine learning models.
Creating the Transformer's Components
An LLM based on transformer architecture comprises multiple components that work together to understand and generate human language. The main components include:
Embedding Layer
This is where input enters the model and is converted into a series of vector representations that can be more efficiently understood and processed.This occurs over several steps:
- A tokenizer breaks down the input into tokens. In some cases, each token is a word but the current favored approach is to divide input into sub-word tokens of approximately four characters or ¾ words.
- Each token is assigned an integer ID and saved in a dictionary to build a vocabulary dynamically.
- Each integer is converted into a multi-dimensional vector, called an embedding, with each characteristic or feature of the token represented by one of the vector’s dimensions.
- A transformer has two embedding layers: one within the encoder for creating input embeddings and the other inside the decoder for creating output embeddings.
Positional Encoder
Instead of utilizing recurrence or maintaining an internal state to track the position tokens within a sequence, the transformer generates positional encodings and adds them to each embedding. This is a key strength of the transformer architecture, as it can process tokens in parallel instead of sequentially and better track long-range dependencies. Like embeddings, a transformer creates positional encoding for both input and output tokens in the encoder and decoder, respectively.
Self-Attention Mechanism
This is the most crucial component of the transformer – and what distinguishes it from other network architectures – as it is responsible for comparing each embedding against others to determine their similarity and semantic relevance. The self-attention layer generates a weighted representation of the input that captures the underlying relationships between tokens, which is used to calculate the most probable output.At each self-attention layer, the input is projected across several smaller dimensional spaces known as heads—hence, multi-head attention. Each head independently focuses on a different aspect of the input sequence in parallel, enabling the LLM to better understand the data in less time.
The original self-attention mechanism has eight heads, but you may decide on a different number based on your objectives. The more attention heads, the greater the required computational resources, which will constrain the choice to the available hardware. The encoder and decoder contain self-attention components: the encoder has one multi-head attention layer while the decoder has two.
Feed-Forward Network
This layer captures the input sequence's higher-level features, i.e., more complex and detailed characteristics, so the transformer can recognize the data’s more intricate underlying relationships. It is comprised of three sub-layers:
- First Linear Layer: This takes the input and projects it onto a higher-dimensional space (e.g., 512 to 2048 in the original transformer) to store more detailed representations.
- Non-Linear Activation Function: This introduces non-linearity into the model, which helps in learning more realistic and nuanced relationships. A commonly used activation function is the Rectified Linear Unit (ReLU).
- Second Linear Layer: This transforms the higher-dimensional representation back to its original dimensionality, compressing the additional information from the higher-dimensional space back to a lower-dimensional space while retaining the most relevant aspects.
Normalization Layers
This layer ensures the input embeddings fall within a reasonable range and helps mitigate vanishing or exploding gradients, stabilizing the language model and allowing for a smoother training process. In particular, the transformer architecture utilizes layer normalization, which normalizes the output for each token at every layer, as opposed to batch normalization, which normalizes across each portion of data used during a time step.
Layer normalization is ideal for transformers because it maintains the relationships between the aspects of each token and does not interfere with the self-attention mechanism.
Residual Connections
Also called skip connections feed the output of one layer directly into the input of another, so data flows through the transformer more efficiently. By preventing information loss, they enable faster and more effective training.During forward propagation, i.e., as training data is fed into the model, residual connections provide an additional pathway that ensures that the original data is preserved and can bypass transformations at that layer.
The Role of Residual Connections
During backward propagation, when the model adjusts its parameters according to its loss function, residual connections help gradients flow more easily through the network, helping to mitigate vanishing gradients, which become increasingly smaller as they pass through more layers.
Assembling the Encoder and Decoder
Once you have created the transformer’s components, you can assemble them to create an encoder and decoder.
Encoder
The encoder's role is to take the input sequence and convert it into a weighted embedding that the decoder can use to generate output. The encoder is constructed as follows:
- Embedding layer
- Positional encoder
- Residual connection that feeds into normalization layer
- Self-attention mechanism
- Normalization layer
- Residual connection that feeds into normalization layer
- Feed-Forward network
- Normalization layer
Decoder
The decoder takes the weighted embedding produced by the encoder to generate output, i.e., the tokens with the highest probability based on the input sequence and its learned parameters. The decoder has a similar architecture to the encoder, with a couple of key differences:
- It has two self-attention layers, while the encoder has one.
- It employs two types of self-attention:
- Masked Multi-Head Attention: Uses a causal masking mechanism to prevent comparisons against future tokens.
- Encoder-Decoder Multi-Head Attention: Each output token calculates attention scores against all input tokens, better establishing the relationship between the input and output for greater accuracy. This cross-attention mechanism also employs causal masking to avoid influence from future output tokens.
This results in the following decoder structure:
- Embedding layer
- Positional encoder
- Residual connection that feeds into normalization layer
- Masked self-attention mechanism
- Normalization layer
- Residual connection that feeds into normalization layer
- Encoder-Decoder self-attention mechanism
- Normalization layer
- Residual connection that feeds into normalization layer
- Feed-Forward network
- Normalization layer
Combine the Encoder and Decoder to Complete the Transformer
Having defined the components and assembled the encoder and decoder, you can combine them to produce a complete transformer.Transformers do not contain a single encoder and decoder – but rather a stack of each in equal sizes, e.g., six in the original transformer. Stacking encoders and decoders in this manner increases the transformer’s capabilities, as each layer captures the different characteristics and underlying patterns from the input to enhance the LLM’s performance.
Data Curation
Once you have built your LLM, the next step is compiling and curating the data that will be used to train it. This is an especially vital part of building an LLM from scratch because the data quality determines the model's quality. While other aspects, such as the model architecture, training time, and training techniques, can be adjusted to improve performance, bad data cannot be overcome. Consequences of low-quality training data include:
- Inaccuracy: A model trained on incorrect data will produce inaccurate answers.
- Bias: Any inherent bias in the data will be learned by the model.
- Unpredictability: The model may produce incoherent or nonsensical answers with it being difficult to determine why.
- Poor Resource Utilization: Ultimately, poor quality prolongs the training process, and incurs higher computational, personnel, and energy costs.
The Role of Data in LLM Performance
As well as requiring high-quality data, you also need vast amounts of data for your model to properly learn linguistic and semantic relationships to carry out natural language processing tasks. As stated earlier, a general rule of thumb is that the more performant and capable you want your LLM to be, the more parameters it requires – and the more data you must curate. To illustrate this, here are a few existing LLMs and the amount of data, in tokens, used to train them:
For better context, 100,000 tokens equate to roughly 75,000 words – or an entire novel. So GPT-3, for instance, was trained on the equivalent of 5 million novels’ worth of data.
Characteristics of a High-Quality Dataset
Let us look at the main characteristics to consider when curating training data for your LLM:
- Filtered for inaccuracies
- Minimal biases and harmful speech
- Cleaned – that the data has been filtered for:
- Misspellings
- Cross-domain homographs
- Spelling variations
- Contractions
- Punctuation
- Boilerplate text
- Markup, e.g., HTML
- Non-textual components, e.g., emojis
- Deduplication: Removing repeated information, as it could increase bias in the model
- Privacy Redaction: Removing confidential or sensitive data
- Diverse: containing data from various formats and subjects, e.g., academic writing, prose, website text, coding samples, mathematics, etc.
Preventing Overfitting in LLM Training
Another crucial component of creating an effective training dataset is retaining a portion of your curated data for evaluating the model. Suppose you use the same data with which you trained your LLM to evaluate it. In that case, you run the risk of overfitting the model, where it becomes familiar with a particular set of data and fails to generalize to new data.
Where Can You Source Data for Training an LLM?
There are several places to source training data for your language model. Depending on the amount of data you need, it is likely that you will draw from each of the sources outlined below:
Existing Public Datasets
Data that has been previously used to train LLM made available for public use. Prominent examples include:
- The Common Crawl: A dataset containing terabytes of raw web data extracted from billions of pages. It also has widely used variations or subsets, including RefinedWeb and C4 (Colossal Cleaned Crawled Corpus).
- The Pile: A popular text corpus that contains data from 22 data sources across 5 categories:
- Academic Writing: e.g., arXiv
- Online or Scraped Resources: e.g., Wikipedia
- Prose: e.g., Project Gutenberg
- Dialog: e.g., YouTube subtitles
- Miscellaneous: e.g., GitHub
- StarCoder: Close to 800GB of coding samples in various programming languages.
- Hugging Face: An online resource hub and community with over 100,000 public datasets.
- Private Datasets: A personally curated dataset you create in-house or purchase from an organization specializing in dataset curation.
- Directly From the Internet: Naturally, scraping data directly from websites en masse is an option, but this is ill-advised because it won’t be cleaned, is likely to contain inaccuracies and biases, and could feature confidential data.
Training Your Custom LLM
The training process for LLMs requires vast amounts of textual data being passed through its neural network to initialize its parameters, i.e., weights and biases. This is composed of two steps:
- Forward propagation
- Backward propagation
Understanding the Forward Propagation Process
During forward propagation, training data is fed into the LLM, which learns the language patterns and semantics required to predict output accurately during inference. The output of each layer of the neural network serves as the input to another layer, until the final output layer, which generates a predicted output based on the input sequence and its learned parameters.Backward propagation updates the LLM’s parameters based on its prediction errors. The model’s gradients, i.e., the extent to which parameters should be adjusted to increase accuracy, are propagated backward through the network.
The parameters of each layer are then adjusted to minimize the loss function. This algorithm calculates the difference between the target output and actual output, providing a quantitative measure of performance. This process iterates over multiple batches of training data, and several epochs, i.e., a complete pass-through of a dataset, until the model’s parameters converge to output that maximizes accuracy.
How Long Does It Take to Train an LLM From Scratch?
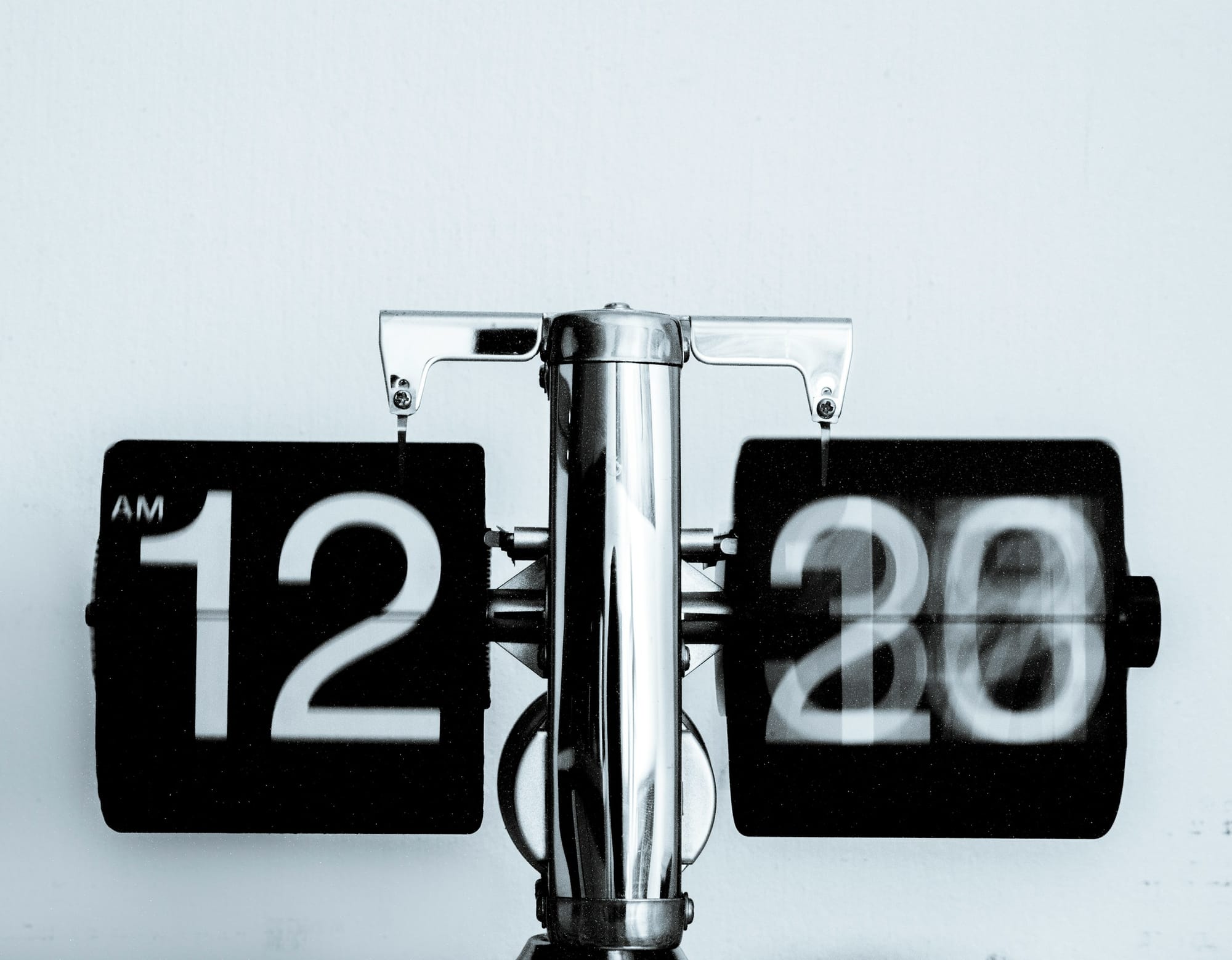
The training process for every model will be different – so there is no set amount of time taken to train an LLM. The amount of training time will depend on a few key factors:
- The complexity of the desired use case.
- The amount, complexity, and quality of available training data.
- Available computational resources.
Training Time
Training an LLM for a relatively simple task on a small dataset may only take a few hours, while training for more complex tasks with a large dataset could take months.
The Risk of Underfitting
Two challenges you must mitigate while training your LLM are underfitting and overfitting. Underfitting can occur when your model is not trained for long enough and the LLM needs more time to capture the relationships in the training data.
Avoiding Overfitting
Training an LLM for too long can result in overfitting, where it learns the patterns in the training data too well, and doesn’t generalize to new data. In light of this, the best time to stop training the LLM is when it consistently produces the expected outcome, and makes accurate predictions on previously unseen data.
LLM Training Techniques
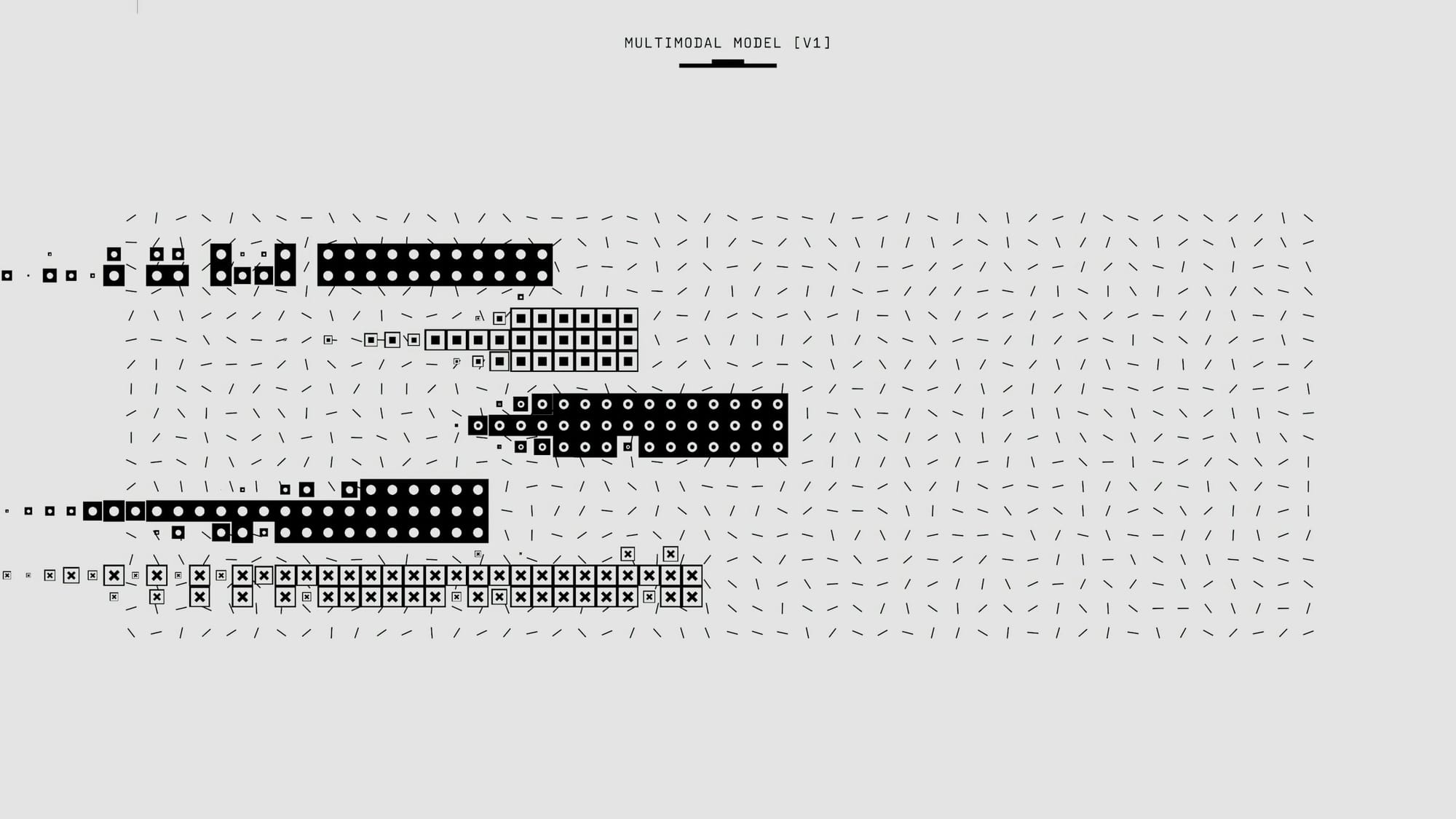
Parallelization
The process of distributing training tasks across multiple GPUs so they are carried out simultaneously. This expedites training times in contrast to using a single processor and efficiently uses GPUs' parallel processing abilities. There are several different parallelization techniques which can be combined for optimal results:
- Data Parallelization: The most common approach, which sees the training data divided into shards and distributed over several GPUs.
- Tensor Parallelization: Divides the matrix multiplications performed by the transformer into smaller calculations that are performed simultaneously on multiple GPUs.
- Pipeline Parallelization: Distributes the transformer layers over multiple GPUs to be processed in parallel.
- Model Parallelization: Distributes the model across several GPUs and uses the same data for each, so each GPU handles one part of the model instead of a portion of the data.
Gradient Checkpointing
Gradient checkpointing is a technique used to reduce the memory requirements of training LLMs. It is a valuable training technique because it makes it more feasible to train LLMs on devices with restricted memory capacity. Subsequently, by mitigating out-of-memory errors, gradient checkpointing helps make the training process more stable and reliable.
Forward Propagation
During forward propagation, the model’s neural network produces a series of intermediate activations: output values derived from the training data that the network later uses to refine its loss function. Though all intermediate activations are calculated with gradient checkpointing, only a subset of them are stored in memory at defined checkpoints.
Backward Propagation
During backward propagation, the intermediate activations that were not stored are recalculated. Nevertheless, only the subset (stored at the checkpoint) needs to be recalculated instead of recalculating all the activations. Although gradient checkpointing reduces memory requirements, the tradeoff is that it increases processing overhead; the more checkpoints used, the greater the overhead.
LLM Hyperparameters
Hyperparameters are configurations you can use to influence how your LLM is trained. In contrast to parameters, hyperparameters are set before training begins and aren’t changed by the training data. Tuning hyperparameters is essential to the training process because it provides a controllable and measurable method of altering your LLM’s behavior to better align with your expectations and defined use case.
Notable hyperparameters include:
- Batch Size: A batch is a collection of instances from the training data, which are fed into the model at a particular timestep. Larger batches require more memory but also accelerate the training process as you get through more data at each interval. Conversely, smaller batches use less memory but prolong training. Generally, it is best to go with the largest data batch your hardware will allow while remaining stable, but finding this optimal batch size requires experimentation.
- Learning Rate: How quickly the LLM updates itself in response to its loss function, i.e., its frequency of incorrect prediction, during training. A higher learning rate expedites training but could cause instability and overfitting. A lower learning rate, in contrast, is more stable and improves generalization – but lengthens the training process.
- Temperature: Adjusts the range of possible output to determine how “creative” the LLM is. Represented by a value between 0.0 (minimum) and 2.0 (maximum), a lower temperature will generate more predictable output, while a higher value increases the randomness and creativity of responses.
Fine-Tuning Your LLM
After training your LLM from scratch with larger, general-purpose datasets, you will have a base or pre-trained language model. To prepare your LLM for your chosen use case, you likely have to fine-tune it. Fine-tuning further trains a base LLM with a smaller, task or domain-specific dataset to enhance its performance on a particular use case.Fine-tuning methods broadly fall into two categories: full fine-tuning and transfer learning:
- Full Fine-Tuning: Where all of the base model’s parameters are updated, creating a new version with altered weighting. This is the most comprehensive way to train an LLM for a specific task or domain but requires more time and resources.
- Transfer Learning: This involves leveraging the significant language knowledge acquired by the model during pre-training and adapting it for a specific domain or use case.
Transfer learning requires many or all of the base LLM’s neural network layers to be “frozen” to limit which parameters can be tuned. The remaining layers – or, often, newly added – unfrozen layers are fine-tuned with the smaller fine-tuning dataset – requiring less time and computational resources than full fine-tuning.
How Do You Evaluate Large Learning Models?
The Large Language Model evaluation can't be subjective. Instead, it has to be a logical process to evaluate the performance of LLMs. Considering the evaluation in classification or regression challenges scenarios, comparing actual tables and predicted labels helps understand how well the model performs. Often, we look at the confusion matrix for this. But what in the case of LLM? They generate text.Don't worry! There are two approaches to evaluating LLMs:
1. Intrinsic Methods
Conventional language models were evaluated using intrinsic methods like bits per character, perplexity, BLUE score, etc. These metric parameters track the performance on the language aspect, i.e., how good the model is at predicting the next word.
- Perplexity: Perplexity measures how well an LLM can predict the next word in a sequence. Lower perplexity indicates better performance.
- BLEU Score: The BLEU score measures how similar the text generated by an LLM is to a reference text. A higher BLEU score indicates better performance.
- Human Evaluation: Human evaluation involves asking human judges to rate the quality of the text generated by an LLM. This can be achieved using various assessments, such as fluency, coherence, and relevance.
It is equally important to note that no one-size-fits-all evaluation metric exists. Each metric has its own strengths and weaknesses. Therefore, it is essential to use a variety of different evaluation methods to get a wholesome picture of the LLM's performance.Here are some additional considerations for evaluating LLMs:
- Dataset Biasing: LLMs are trained on large datasets of text and code. If these datasets are biased, then the LLM will also be limited. It is essential to be aware of the potential for bias in the dataset and to take steps to mitigate it.
- Safety: LLMs can generate harmful content, such as hate speech and misinformation. Therefore, it is essential to develop protection mechanisms to prevent this.
- Transparency: It is essential to be transparent about how LLMs are trained and evaluated. This will help build trust in LLMs and ensure they are used responsibly.
2. Extrinsic Methods
With advancements in LLMs nowadays, extrinsic methods are becoming the top pick for evaluating their performance. The suggested approach is to look at their performance in different tasks like reasoning, problem-solving, computer science, mathematical problems, competitive exams, etc.EleutherAI launched a framework termed Language Model Evaluation Harness to compare and evaluate LLM's performance. HuggingFace integrated the evaluation framework to weigh open-source LLMs created by the community. This framework evaluates LLMs across four different datasets. The final score is an accumulation of scores from each dataset.
Here are the parameters:
- A12 Reasoning: This is a collection of science questions created for elementary school students.
- MMLU: This is a comprehensive test that evaluates the multitask precision of a text model. It sheaths 57 different tasks, including subjects like U.S. history, math, law, and much more.
- TruthfulQA: This test assesses a model's tendency to create accurate answers and skip generating false information commonly found online.
- HellaSwag: This is a test that challenges state-of-the-art models to make common-sense inferences that are easy for humans, with 95% precision.
Deploying the LLM
It's time to deploy the LLM in a production environment. You can choose serverless technologies like AWS Lambda or Google Cloud Functions to deploy the model as a web service. Besides, you can use containerization technologies like Docker to package our model and its dependencies in a single container.
Related Reading
- How to Fine Tune LLM
- LLM Function Calling
- LLM Prompting
- What LLM Does Copilot Use
- LLM Evaluation Metrics
- LLM Use Cases
- LLM Sentiment Analysis
- LLM Evaluation Framework
- LLM Benchmarks
- Best LLM for Coding
Key Considerations in Creating an LLM
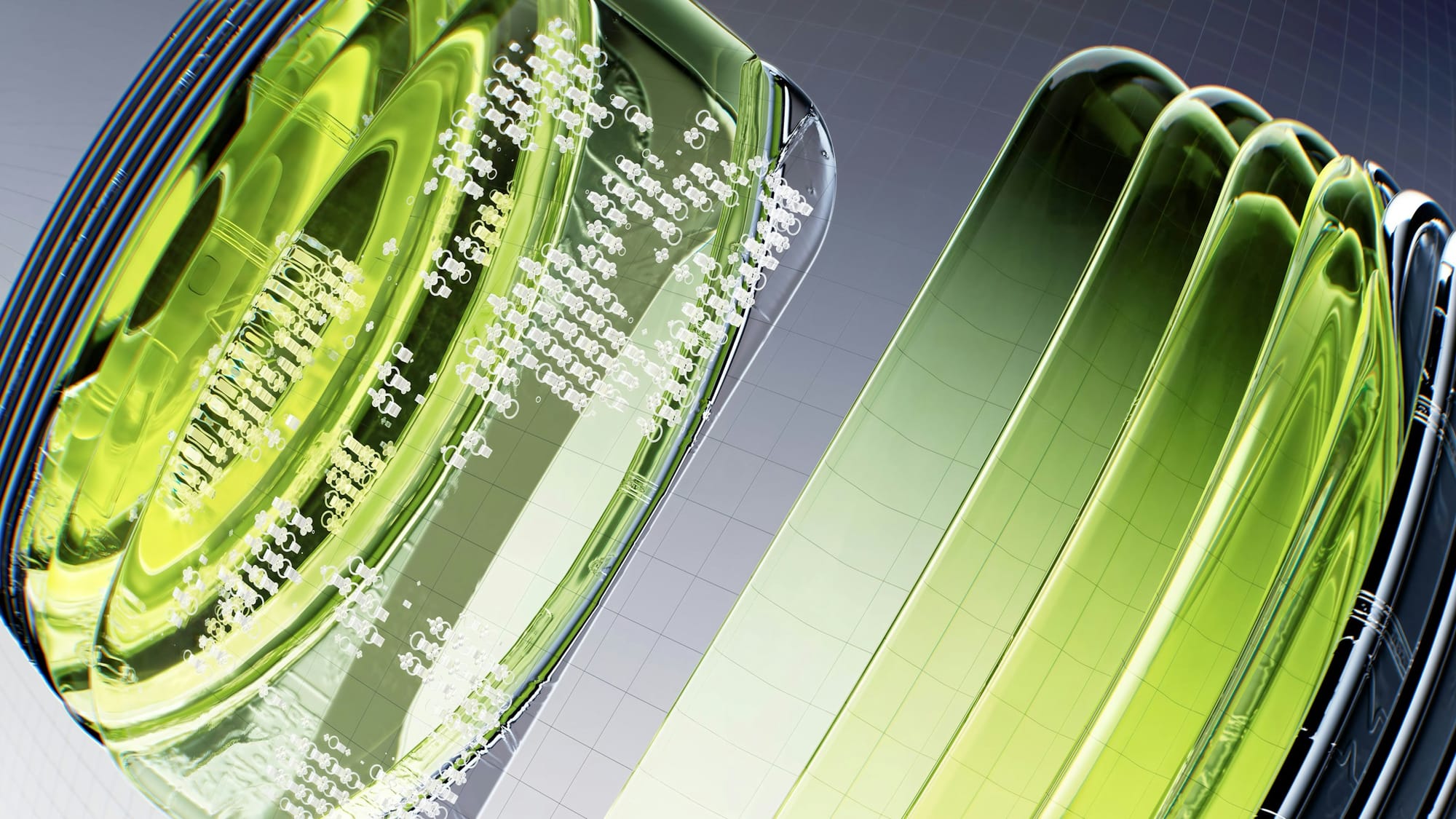
Data Collection and Quality: The First Hurdle to Building Your Own LLM Model
To train a robust large language model, you need high-quality data. Collecting, organizing, and processing this data is often the first step in the LLM development process. Practical training of a satisfactorily performing LLM entails using a massive amount of data with high variety. Collection of such data is a challenging endeavor.
The data needs to be diverse in the topics discussed, languages used, and environments in which the information was available online. Controlling the content of the data collected is essential so that data errors, biases, and irrelevant content are kept to a minimum. Low-quality data impacts the quality of further analysis and the models built, affecting the LLM's performance.
Computational Resources: Why You Need a Powerful Computer to Build Your Own LLM
Training LLMs, especially those with billions of parameters, requires large amounts of computation. This includes GPUs or TPUs, which are pricey and heavily energy-intensive. Coordinating and expanding computational resources to accommodate numerous training procedures can sometimes be technically complex and laborious.
Model Complexity: Choose Your Architecture Wisely When Building an LLM
It is crucial to correctly select the LLM architecture (for example, autoregressive, autoencoding, or combined ones) depending on the concrete problem that will be solved. Each architecture has advantages and disadvantages, and a wrong decision can lead to poor results.
Tweaking the hyperparameters (for instance, learning rate, batch size, number of layers, etc.) is a very time-consuming process that has a decided influence on the result. It requires experts, and this usually entails a considerable amount of trial and error.
Data Privacy and Security: Protect Sensitive Information When Building Your Own LLM
When processing sensitive data during the training phase, privacy issues can occur. The importance of enforcing measures such as federated learning and differential privacy cannot be overemphasized. However, they increase the difficulty level. Complying with data protection regulations (for example, GDPR, CCPA) is obligatory.
This requires proper data and documentation management so that an organization will not be harmed by legal actions. Data privacy and security are critical in creating an LLM, as they involve ensuring compliance with regulations like GDPR and preventing sensitive data leaks during the training phase.
Cost Management: LLMs Are Expensive to Build and Maintain
The capital investment required to create an LLM model for data acquisition, computing resources, and talent is huge. These costs may be expensive for SMEs, which may not be able to meet them as effectively as big organizations.
Additional costs accompany the maintenance and improvement of the LLM. Since developing the LLM was not a one-time process, sustaining and enhancing it also has recurring expenses. Efficiency of resource management is needed to prevent these costs from escalating.
Expertise and Talent: You Need Knowledgeable People to Build LLMs
Developing and especially tuning an NLP model such as an LLM entails knowledge in machine learning, data science, and, more specifically, NLP. Securing such talent is quite a process, especially when the market is competitive and human resources must endure a learning curve before the candidate is hired.
The field in which LLMs are concentrated is dynamic and developing very fast. One has to constantly learn to remain informed of current research and available technological solutions. It is about constant development.
Ethical Considerations: Build an LLM That Is Fair and Unbiased
It is important to eliminate bias in the model and reflect on its potential for presenting a fair outcome. This includes paying particular attention to the data used during training and measures put in place to counteract this. Staying ahead of the curve regarding how LLMs are employed and created is a continuous challenge due to the significant danger of having LLMs that spread information unethically.
Start Building GenAI Apps for Free Today with Our Managed Generative AI Tech Stack
Lamatic provides a managed generative AI tech stack that helps teams implement generative AI technologies efficiently. Our solution includes managed generative AI middleware, a custom GraphQL API, and a low-code agent builder that streamlines the process of building AI applications.
We also incorporate an automated generative AI workflow to ensure production-ready deployments, integrated vector database support, and edge deployment via Cloudflare workers. Starting with Lamatic's generative AI tech stack can get your business up and running with AI capabilities without accruing technical debt or hassle.
Accelerate AI Adoption
Lamatic can help your company rapidly implement generative AI technologies with less risk and technical debt. Our solution offers an automated workflow to ensure production-ready deployments and helps teams get started with building AI applications quickly.