Generative AI is one of the most promising technologies of our time, potentially transforming entire industries. But, as with any emerging technology, challenges come with the territory. For instance, let’s say you’ve developed a product that integrates generative AI to personalize user experiences. It’s not long before you realize AI produces biased outputs that reinforce stereotypes. Not only is this problematic for the users, but it can also get you into hot water with regulators and destroy your product’s reputation. This scenario illustrates some of the generative AI challenges developers face when building AI to function safely, securely, and in compliance with standards. This article will offer valuable insights to help you address these concerns as you work to integrate generative AI into your product, ensuring it operates ethically and without compromising user trust or organizational values.
At Lamatic, we provide solutions to help businesses successfully tackle generative AI challenges, so you don’t have to go it alone. Our Generative AI tech stack offers a structured approach to building generative AI products, focusing on safety, security, and compliance.
What is the Generative AI Adoption Rate in 2024?
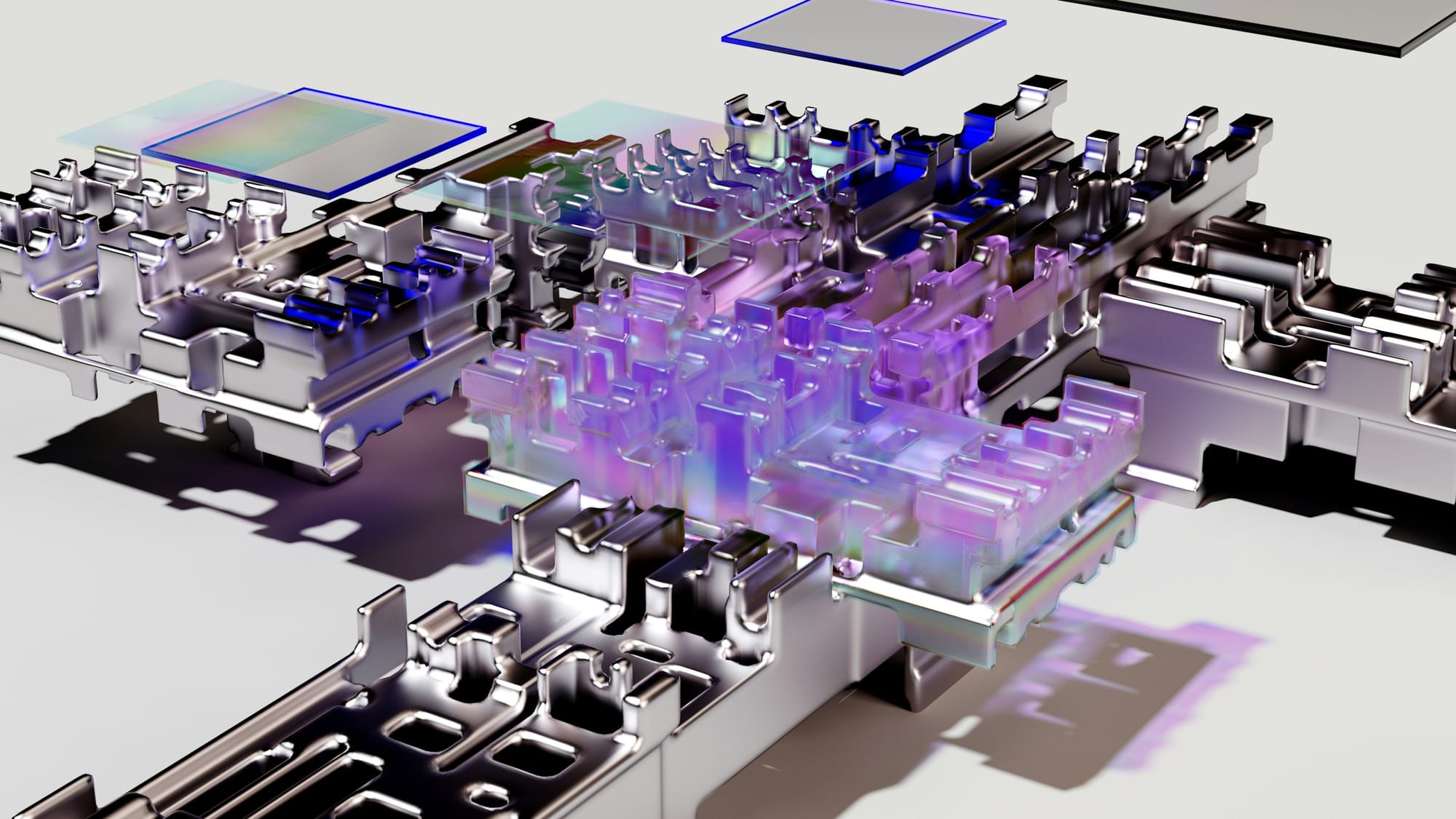
The current state of generative AI adoption is impressive. If 2023 was the year the world discovered generative AI, 2024 was when organizations began using and deriving business value from this new technology.
In the latest McKinsey Global Survey on AI, 65 percent of respondents report that their organizations regularly use generative AI, nearly double the percentage from our previous survey just ten months ago. Respondents’ expectations for generative AI’s impact remain as high as last year, with three-quarters predicting that generative AI will lead to significant or disruptive change in their industries in the years ahead.
Who’s Using Generative AI in 2024?
Organizations already see material benefits from generative AI use, reporting cost decreases, and revenue jumps in the business units deploying the technology. The survey also provides insights into the risks presented by generative AI, most notably inaccuracy and the emerging practices of top performers to mitigate those challenges and capture value.
Generative AI Adoption Surges
Interest in generative AI has also highlighted a broader set of AI capabilities. For the past six years, AI adoption by respondents’ organizations has hovered around 50 percent. This year, the survey finds that adoption has jumped to 72 percent.
And the interest is truly global in scope. Our 2023 survey found that AI adoption did not reach 66 percent in any region; however, this year, more than two-thirds of respondents in nearly every region say their organizations are using AI. Looking by industry, the biggest increase in adoption can be found in professional services.
Responses suggest that companies now use AI in more parts of the business. Half of respondents say their organizations have adopted AI in two or more business functions, up from less than a third of respondents in 2023.
Investments in Generative AI and Analytical AI Are Beginning to Create Value
The latest survey also shows how different industries are budgeting for generative AI. Responses suggest that organizations in many industries are about equally as likely to invest more than 5 percent of their digital budgets in generative AI as in nongenerative, analytical AI solutions. In most industries, larger shares of respondents report that their organizations spend more than 20 percent on analytical AI than on generative AI. Most respondents, 67 percent, expect their organizations to invest more in AI over the next three years.
Regarding motivations for adopting generative AI tooling, the top reasons remain consistent between 2023 and 2024. Respondents cite generative AI's ability to expedite processes through automation (88 percent), lower costs (68 percent), and improve results through workflow optimization (58 percent) as reasons for adoption. In 2024, even more importance was placed on expediting processes (15 percentage point increase) and cutting costs (13 percentage point increase), suggesting that companies have seen tangible benefits in their workloads and expenses thanks to generative AI technologies.
McKinsey Commentary
In 2024, generative AI is no longer a novelty. Nearly two-thirds of respondents to our survey report that their organizations regularly use generative AI, nearly double what our previous survey found just ten months ago, and four in ten are using generative AI in more than two business functions. The technology’s potential is no longer in question. And while most organizations are still in the early stages of their journeys with generative AI, we are beginning to get a picture of what works and what doesn’t in implementing—and generating actual value with the technology.
One thing we’ve learned
The business goal must be paramount. In our work with clients, we ask them to identify their most promising business opportunities and strategies and then work backward to potential generative AI applications. Leaders must avoid the trap of pursuing tech for tech’s sake. The greatest rewards also will go to those who are not afraid to think big. As we’ve observed, the leading companies are the ones that are focusing on reimagining entire workflows with generative AI and analytical AI rather than simply seeking to embed these tools into their current ways of working.
For that to be effective, leaders must be ready to manage change at every step. And they should expect that change to be constant:
- Enterprises will need to design a robust
- Cost-efficient
- Scalable
Generative AI stack for years to come. They’ll also need to draw on leaders from throughout the organization. Realizing the profit-and-loss impact of generative AI requires close partnerships with HR, finance, legal, and risk to constantly readjust resourcing strategies and productivity expectations.
Data Security Tops Concerns Amid Growing Adoption
If the benefits of generative AI are becoming more visible, so are the risks. Security has emerged as a top concern as the public becomes more aware of generative AI's drawbacks, from the legal risks surrounding training data for large language models to the ongoing problem of hallucinations. Seventy-two percent of respondents cite data security as their main worry when implementing generative AI in the workplace, up 40 percent since last year. This is a big shift from 2023, where "unclear value" was cited as the biggest risk in adopting generative AI.
Related Reading
- How to Build AI
- Gen AI vs AI
- GenAI Applications
- Generative AI Customer Experience
- Generative AI Automation
- Generative AI Risks
- How to Create an AI App
- AI Product Development
- GenAI Tools
- Enterprise Generative AI Tools
- Generative AI Development Services
10 Key Generative AI Challenges To Address for Responsible Use
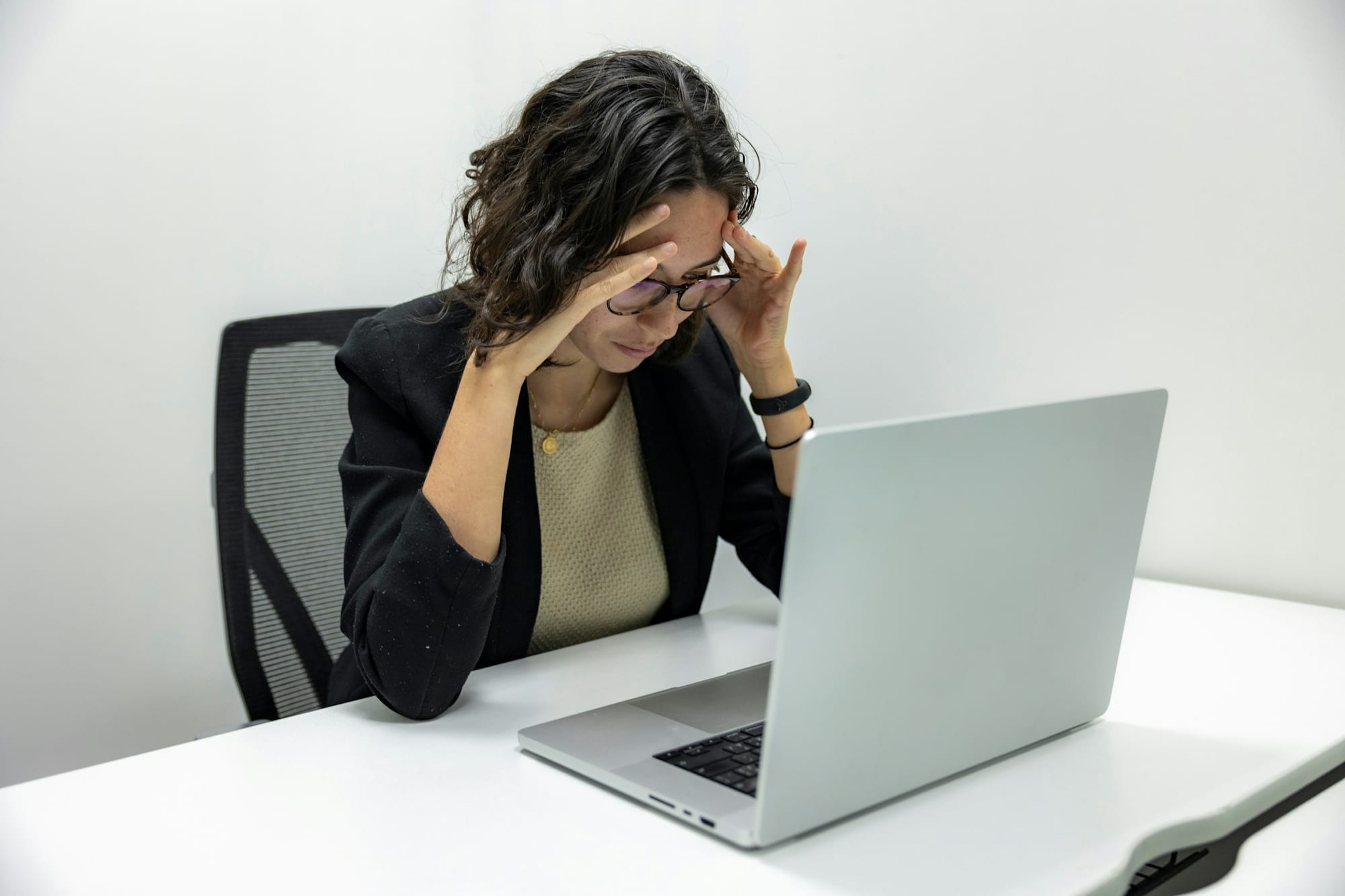
1. Handling Technical Complexity: The Challenge of Massive AI Models
Generative AI models can contain billions or even trillions of parameters. This makes them a complex undertaking for the typical business. "These models are impractically large to train for most organizations," said Arun Chandrasekaran, vice president, analyst, and tech innovation at Gartner.
He said that the necessary computing resources can make this technology expensive and ecologically unfriendly, so most near-term adoption will likely see businesses consume generative AI through cloud APIs with limited tuning. Chandrasekaran added that the difficulty in creating models leads to another issue: the concentration of power in a few deep-pocketed entities.
2: Tackling Legacy Systems: Integrating New Tech with Old
Incorporating generative AI into older technology environments could raise additional issues for enterprises. IT leaders will face decisions on whether to integrate or replace older systems. For example, Pablo Alejo, partner at consultancy West Monroe, said financial institutions considering how a language model could be used to determine fraud will probably find the emerging technology at odds with how their current systems handle that task.
Legacy systems "have a very specific way of doing that, and now you've got generative AI that's leveraging way different types of thinking," Alejo explained. "Organizations have to find new ways to either create integrations or adopt new capabilities, with new technologies, that enable them to reach the same outputs, or outcomes, faster and more effectively."
3: Avoiding Technical Debt: Don't Let Generative AI Add to Your Problems
Generative AI could join legacy systems as technical debt if businesses fail to achieve significant change through its adoption. An enterprise deploying AI models for customer support might declare an optimization victory because human agents will handle fewer cases.
However, according to Bill Bragg, CIO at enterprise AI SaaS provider SymphonyAI, workload reduction needs to go further. He noted that a business would need to significantly reduce the number of agents in front-line support roles to justify the investment in AI. "If you don't take something away, how have you optimized?" Bragg said. "All you've done is add more debt to my processes."
4: Reshaping Some of the Workforce: Generative AI Will Change Jobs, Not Eliminate Them
Generative AI will likely restructure how work gets done in many fields, a prospect that raises job-loss concerns. An article on the Chinese video game industry states job opportunities for artists are vanishing as companies employ AI-based image generators. "If you don't have a team working on understanding [generative AI's] capability and taking advantage of it, you are risking obsolescence." Pablo Alejo is a partner at West Monroe, but some executives suggest it's not all doom and gloom.
AI might reduce the number of agents in the customer support example, but the technology would also create other roles, Bragg said. He reasoned that a business would need staff to oversee and improve the AI-assisted customer experience. Bragg referred to this transition as "going from the doer to the trainer." Similarly, Alejo said generative AI will remove some types of jobs but also "open up brand new types of jobs that those same people can take advantage of."
5: Monitoring for Potential Misuse and AI Hallucinations: Keep an Eye on What AI is Generating
AI models lower the cost of content creation. That helps businesses but also helps threat actors who can more easily modify existing content to create deepfakes. Digitally altered media can closely mimic the original and be hyperpersonalized. "This includes everything from voice and video impersonation to fake art, as well as targeted attacks," Chandrasekaran said.
While threat actors can misuse generative AI systems, the models can lead users astray: AI hallucinations provide misinformation and make up facts. Depending on the domain, hallucination rates could be 10% to 20% of the responses of the AI tools, Chandrasekaran added.
6: Keeping Tabs on Legal Concerns and Algorithmic Bias: Staying Ahead of the Risks
The emerging technology can also bump into intellectual property issues, exposing businesses to legal action. "Generative AI models have the added risk of seeking training data at massive scale, without considering the creator's approval, which could lead to copyright issues," Chandrasekaran said. Algorithmic bias is another source of legal risk.
Generative AI models, when trained on faulty, incomplete, or unrepresentative data, will produce systemically prejudiced results. AI bias spreads through the systems and influences decision-makers relying on the results, potentially leading to discrimination. Flawed AI models "can propagate downstream bias in the data sets, and the homogenization of such models can lead to a single point of failure," Chandrasekaran said.
7: Providing Coordination and Oversight: Governance is Key to Responsible AI
Newer technologies often require organizations to launch centers of excellence (CoE) to focus on effective adoption and rollout. Such centers could play an important role in generative AI. "If you don't have a team working on understanding this capability and taking advantage of it, you are risking obsolescence," Alejo warned. "Centers of excellence should exist across every industry, across every organization."
Such a specialized group can also craft policies governing the acceptable use of generative AI. Alejo advised that the CoE "should lead policy design and decisions for how different individuals across an organization can use it." The center, he added, should enlist the review and input of key stakeholders, including legal, IT, risk, and, potentially, other departments such as marketing, HR, and R&D.
8: AI Ethics and Responsibility: Who is Accountable for AI's Decisions?
With AI's rapid uptake across various sectors, complex ethical and responsibility questions emerge. Transparent decision-making by generative AI (gen AI) tools becomes particularly essential, along with being able to explain those decisions to those impacted. Legally speaking, AI-driven decisions can pose risks of noncompliance with existing regulations.
Europe's General Data Protection Regulation (GDPR) includes an individual right of explanation, which permits individuals to demand clarity for automated decisions made about them. The Algorithmic Accountability Act mandates that companies in the US conduct impact evaluations of high-risk AI systems. Biases in AI may lead to discriminatory outcomes and violate regulations. Additionally, countries around the globe are developing specific AI laws that should ensure their systems align with these standards.
9: Establishing Return on Investment: Can You Measure Success?
Establishing ROI with artificial intelligence investments can be complex. Benefits, like improved process efficiencies or customer service enhancement, may not easily translate to specific financial metrics. The investments usually wait to bear fruit - necessitating long-term thinking from companies when measuring return.
10: Dependence on the 3rd Party Platform: Don't Get Stuck Using Outdated Tech
Given its rapid expansion, companies employing generative AI technology may find it challenging. What would you do if your government suddenly outlawed one product model or another that was more affordable, potent, and suitable for your use case? To keep pace with changes that arise over time, you must always remain prepared to adapt quickly enough to remain effective and relevant in business operations.
Related Reading
- Gen AI Architecture
- Generative AI Implementation
- Gen AI Platforms
- Generative AI Providers
- How to Train a Generative AI Model
- Generative AI Infrastructure
- AI Middleware
- Top AI Cloud Business Management Platform Tools
How to Overcome the Challenges in Generative AI
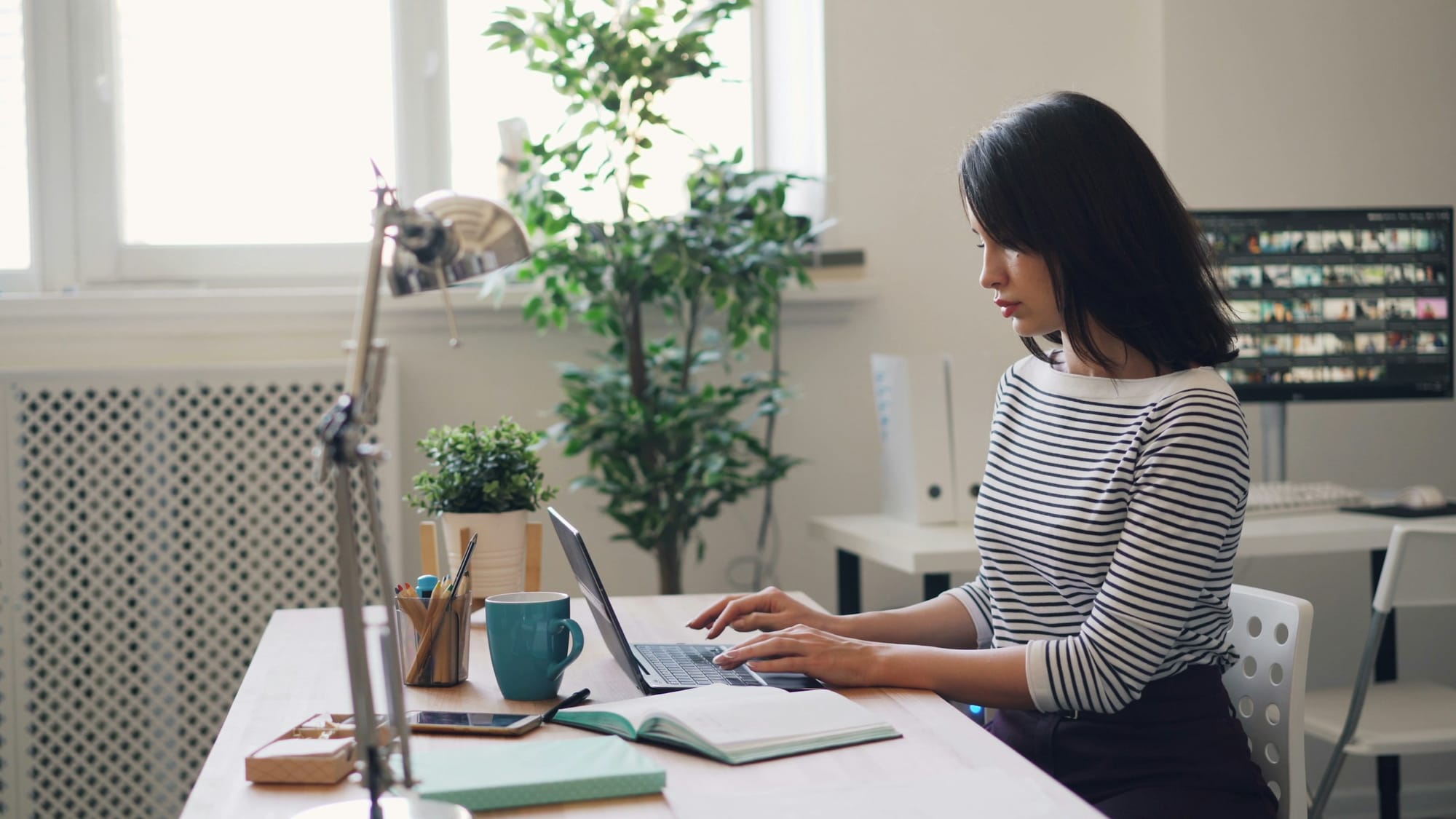
Quality of Output Depends on Data Inputs
Generative AI systems depend heavily on data. If input data is biased, incomplete, or erroneous, Generative AI’s outputs may reflect those flaws, rendering them unreliable or harmful.
This relationship means that Generative AI results correlate directly to the data quality used during the model's initial training. The good news is that organizations can improve the quality of data and, in turn, the quality of Generative AI outputs by implementing actionable strategies, such as:
- Data Auditing: Regularly review and sanitize data for inaccuracies and biases.
- Diverse Datasets: Look beyond internal data to enable more holistic responses. For example, news data can provide real-world context to inform customer data analysis.
- Human-led Tuning: Implement feedback loops to allow manual adjustment of the inference model to optimize performance over time continuously.
Ethical Concerns & Accountability in the Use of Generative AI
As helpful as generative AI can be, it can produce content that sometimes blurs ethical lines, potentially leading to misinformation, misrepresentation, or misuse. Determining who is accountable when an AI system produces harmful or misleading content becomes critical. Putting guardrails in place can help:
- Ethical Frameworks: Establish robust ethical guidelines and usage policies to help ensure generative AI is used responsibly.
- Transparency: Maintain transparency in AI operations and decision-making processes. This transparency should also extend to customers. If generative AI is used in a chatbot or other customer-facing platforms, telling users up front helps you build trust.
- Accountability Measures: Implement mechanisms to trace and audit AI-generated content. And it wouldn’t hurt to provide digital literacy labs. Just as users needed to learn how to conduct internet searches, identify reliable sources, and apply critical thinking when the World Wide Web burst into public view, digital literacy is crucial for effective use of generative AI.
Maintaining Legal & Regulatory Compliance in the Dynamic Environment of Generative AI
With the rapid advancement of generative AI, legal and regulatory frameworks will be in a constant state of flux, making adherence challenging. AI operations might inadvertently breach regional or global regulations, leading to legal ramifications. Strategies to consider include:
- Policy Updates: Keep abreast of global policy changes and adapt operations accordingly. For example, the EU is well on its way to creating transparency requirements and other safeguards after passing a bill in June 2023. Legislators in the U.S. also have generative AI on their radar.
- Legal Expertise: Engage legal professionals experienced in AI, copyright compliance, and technology law.
- Compliance Audits: Regularly audit AI operations and outputs for compliance with existing and emerging regulations. In addition, validate that the third-party data you source comes from a provider that works with publishers and stays in scope with licensing agreements to ensure data is sourced ethically and legally.
Maintaining Authenticity & Originality When Using Generative AI
There's a risk with generative AI that the content produced might mirror existing works, undermining authenticity and originality. Furthermore, differentiating between AI-generated and human-made content becomes increasingly difficult, raising concerns about genuineness in various fields. To help ensure what’s being generated meets your standards, consider:
- Regular Auditing: Auditing content generated by generative AI appears more than once for a good reason. Frequent assessments are a necessity as use and capabilities of generative AI grow. In this case, auditing for originality helps mitigate the risk of inauthentic or sub-standard outputs.
- Innovation Inclusion: Continuously integrate new data and ideas to fuel innovative outputs. If the data fueling generative AI isn’t evolving, your outputs won’t evolve either.
- Plagiarism Checks: Use advanced plagiarism-detection tools to ensure content authenticity.
Enabling Accessibility & Usability Where Generative AI Offers Greatest Value Potential
AI tools, especially sophisticated ones, might present steep learning curves or lack accessibility features. This can hinder adoption across varied user demographics, limiting the technology's reach and potential benefits. Develop generative AI solutions with the user in mind with these strategies:
- User-Centered Design: Adopt a user-centered design philosophy to make applications intuitive.
- Accessibility Features: Integrate features that ensure accessibility for differently abled individuals.
- User Education: Provide ample resources and training to facilitate easy adoption among users. Live demos with Q&As, as well as recorded demos and other training materials can help internal or external users get the most value from data delivery and generative AI tools.
Ensuring Security & Privacy
The vast amount of data utilized by AI systems poses significant security risks, and there's potential for misuse or breaches. Additionally, protecting the privacy of individuals whose data is used for training or operations becomes paramount. Whether you’re concerned about IP leakage or accidental use of sensitive, private, or proprietary information, establishing a strong security foundation can help:
- Robust Encryption: Adopt top-tier encryption technologies to secure data inputs and outputs.
- Privacy Policies: Develop and enforce rigorous data privacy policies, including a framework for allowable datasets and data anonymization recommendations.
- Regular Security Audits: Conduct frequent security audits and updates, particularly for data with higher risks, such as personally identifiable information (PII).
Ensuring Scalability & Adaptability
As your organization increases adoption and use of generative AI, ensure your solutions are designed to scale and adapt accordingly. Doing so without compromising efficiency, speed, or accuracy becomes a complex endeavor, so keep these tips in mind:
- Modular Design: Build AI systems with modular architectures to facilitate scalability.
- Staged Rollout: Some departments, such as those associated with creativity like marketing, have a natural affinity with generative AI. By starting with familiar use cases first, you can build interest and buy-in for further expansion.
- Future-Proof Strategies: Develop strategies that cater to future expansions and adaptability.
- Resource Planning: Implement strategic resource planning to accommodate secure growth. As PWC notes, “The key to rapid ROI and far-reaching transformation through generative AI is a focus on discipline, scale, and trust.”
Addressing the Societal Impact and Public Perception of Generative AI
The rapid rise of AI technologies has led to both awe and skepticism among the public. On any given day, you can find plenty of print and broadcast news covering generative AI, likely running the gamut from the greatest thing since sliced bread to robotic doom and gloom.
Balancing technological advancements with societal impacts is crucial, as is managing public and internal perceptions to ensure trust and beneficial integration.
- Public Engagement: Engage with the public and stakeholders to build trust and gather feedback.
- Social Impact Analysis: Assess and address the societal impacts of AI applications, particularly in areas where inadvertent bias could negatively impact your organization.
- Ethical Operations: Ensure operations align with societal norms and ethical considerations.
Related Reading
- AI Application Development
- Best AI App Builder
- AI Development Platforms
- AI Development Cost
- SageMaker Alternatives
- Gemini Alternatives
- LangChain Alternatives
- Flowise AI
Start Building GenAI Apps for Free Today with Our Managed Generative AI Tech Stack
Lamatic offers a comprehensive Generative AI tech stack that empowers teams to rapidly implement GenAI solutions without accruing tech debt. Our platform provides:
- Managed GenAI Middleware
- Custom GenAI API (GraphQL)
- Low Code Agent Builder
- Automated GenAI Workflow (CI/CD)
- GenOps (DevOps for GenAI)
- Edge deployment via Cloudflare workers
- Integrated Vector Database (Weaviate)
Lamatic empowers teams to rapidly implement GenAI solutions without accruing tech debt. Our platform automates workflows and ensures production-grade deployment on the edge, enabling fast, efficient GenAI integration for products needing swift AI capabilities.
Start building GenAI apps for free today with our generative AI tech stack.
Benefits of Lamatic’s Generative AI Stack
Lamatic’s Generative AI Stack helps teams accelerate the implementation of AI features:
- Its low-code interface simplifies development by allowing users to build applications with minimal coding.
- The stack also automates processes like testing and deployment so teams can focus on building innovative applications instead of getting bogged down in operational details.
- Lamatic’s solution eliminates tech debt by providing a production-ready framework that ensures GenAI applications are reliable and scalable.