Let's say you're about to kick off a new generative AI project. You've settled on a goal, gathered your team, and even created a nifty timeline. But when you drill down into the specifics of your project, you realize you need to choose the right AI model to help you achieve your objectives. This is no small task. AI models come in all shapes and sizes, each with its strengths and weaknesses. Two of the most promising and widely publicized models are foundation models and large language models (LLMs). The nuances of the Foundation Model vs multimodal LLM discussion can help you decide what type of AI model will best serve your project. In this article, we'll unpack the key similarities and differences between foundation models and LLMs to help you confidently choose the right model to enhance your generative AI product's functionality and performance.
Lamatic’s generative AI tech stack is another valuable tool that can help you achieve your goal of understanding the key differences between foundation models and LLMs so that you can make informed, strategic decisions when selecting the right AI model for your specific needs.
What is a Foundation Model in Generative AI?
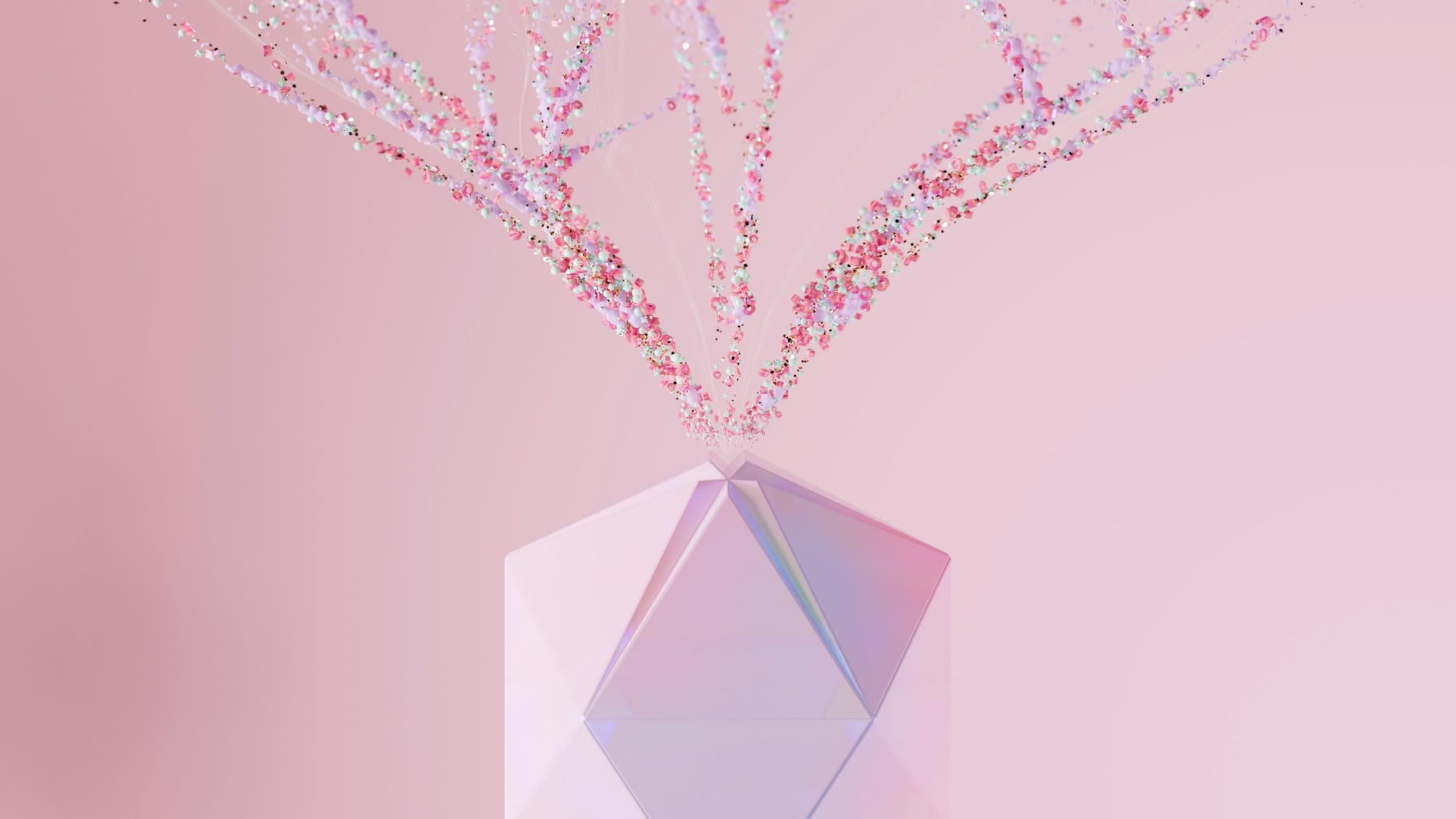
Trained on massive datasets, foundation models (FMs) are large deep-learning neural networks that have changed the way data scientists approach machine learning (ML). Rather than develop artificial intelligence (AI) from scratch, data scientists use a foundation model as a starting point to develop ML models that power new applications more quickly and cost-effectively.
Researchers coined the term foundation model to describe ML models trained on a broad spectrum of generalized and unlabeled data and capable of performing a wide variety of general tasks such as:
- Understanding language
- Generating text and images
- Conversing in natural language
What Makes Foundation Models Unique?
A unique feature of foundation models is their adaptability. Based on input prompts, these models can perform a wide range of disparate tasks with a high degree of accuracy. Some tasks include:
- Natural language processing (NLP)
- Question answering
- Image classification
The size and general-purpose nature of FMs make them different from traditional ML models, which typically perform specific tasks, like:
- Analyzing text for sentiment
- Classifying images
- Forecasting trends
You can use foundation models as base models for developing more specialized downstream applications. These models culminate over a decade of work that saw them increase in size and complexity.
The Rapid Evolution of Foundation Models
BERT, one of the first bidirectional foundation models, was released in 2018. It was trained using 340 million parameters and a 16 GB training dataset. Only five years later, in 2023, OpenAI trained GPT-4 using 170 trillion parameters and a 45 GB training dataset.
According to OpenAI, the computational power required for foundation modeling has doubled every 3.4 months since 2012. Today’s FMs, such as:
- Large language models (LLMs)
- Claude 2 and Llama 2
- Text-to-image model
Stable Diffusion from Stability AI, can perform a range of tasks out of the box spanning multiple domains, like:
- Writing blog posts
- Generating images
- Solving math problems
- Engaging in dialog
- Answering questions based on a document
Why Foundation Models Matter
Foundation models are poised to change the machine learning lifecycle significantly. Although it costs millions of dollars to develop a foundation model from scratch, they’re useful in the long run. It’s faster and cheaper for data scientists to use pre-trained FMs to develop new ML applications rather than train unique ML models from the ground up.One potential use is automating tasks and processes, especially those that require reasoning capabilities.
Here are a few applications for foundation models:
- Customer support
- Language translation
- Content generation
- Copywriting
- Image classification
- High-resolution image creation and editing
- Document extraction
- Robotics
- Healthcare
- Autonomous vehicles
How Do Foundation Models Work?
Foundation models are a form of generative artificial intelligence (generative AI). They generate output from one or more inputs (prompts) in the form of human language instructions. Models are based on complex neural networks including:
- Generative adversarial networks (GANs)
- Transformers
- Variational encoders
The Shared Principles of Network Functioning
Although each type of network functions differently, the principles behind how they work are similar. An FM generally uses learned patterns and relationships to predict the next item in a sequence. For example, with image generation, the model analyzes the image and creates a sharper, more clearly defined version of the image. Similarly, with text, the model predicts the next word in a string of text based on the previous words and its context. It then selects the next word using probability distribution techniques.
Foundation models use self-supervised learning to create labels from input data. This means no one has instructed or trained the model with labeled training data sets. This feature separates LLMs from previous ML architectures using supervised or unsupervised learning.
What Can Foundation Models Do?
Foundation models, even though are pre-trained, can continue to learn from data inputs or prompts during inference. This means that you can develop comprehensive outputs through carefully curated prompts. FMs can perform tasks include language processing, visual comprehension, code generation, and human-centered engagement.
Language Processing
These models have remarkable capabilities to answer natural language questions and even the ability to write short scripts or articles in response to prompts. They can also translate languages using NLP technologies.
Visual Comprehension
FMs excel in computer vision, especially with regard to identifying images and physical objects. These capabilities may be used in applications such as autonomous driving and robotics. Another capability is the generation of images from input text, as well as photo and video editing.
Code Generation
Foundation models can generate computer code in various programming languages based on natural language inputs. It’s also feasible to use FMs to evaluate and debug code. Learn more about AI code generation.
Human-centered Engagement
Generative AI models use human inputs to learn and improve predictions. An important and sometimes overlooked application is their ability to support human decision-making. Potential uses include clinical diagnoses, decision-support systems, and analytics.Another capability is the development of new AI applications by fine-tuning existing foundation models.
Speech to Text
Since FMs understand language, they can be used for speech to text tasks such a transcription and video captioning in a variety of languages.
What Are Examples of Foundation Models?
The number and size of foundation models on the market have grown at a rapid pace. There are now dozens of models available.
Here is a list of prominent foundation models released since 2018.
BERT
Released in 2018, Bidirectional Encoder Representations from Transformers (BERT) was one of the first foundation models. BERT is a bidirectional model that analyzes the context of a complete sequence then makes a prediction. It was trained on a plain text corpus and Wikipedia using 3.3 billion tokens (words) and 340 million parameters. BERT can answer questions, predict sentences, and translate texts.
GPT
The Generative Pre-trained Transformer (GPT) model was developed by OpenAI in 2018. It uses a 12-layer transformer decoder with a self-attention mechanism. And it was trained on the BookCorpus dataset, which holds over 11,000 free novels. A notable feature of GPT-1 is the ability to do zero-shot learning.
GPT-2 released in 2019. OpenAI trained it using 1.5 billion parameters (compared to the 117 million parameters used on GPT-1). GPT-3 has a 96-layer neural network and 175 billion parameters and is trained using the 500-billion-word Common Crawl dataset. The popular ChatGPT chatbot is based on GPT-3.5. And GPT-4, the latest version, launched in late 2022 and successfully passed the Uniform Bar Examination with a score of 297 (76%).
Amazon Titan
Amazon Titan FMs are pretrained on large datasets, making them powerful, general-purpose models. They can be used as is or customized privately with company-specific data for a particular task without annotating large volumes of data. Initially, Titan will offer two models. The first is a generative LLM for tasks such as summarization, text generation, classification, open-ended Q&A, and information extraction. The second is an embeddings LLM that translates text inputs including words, phrases, and large units of text into numerical representations (known as embeddings) that contain the semantic meaning of the text.
While this LLM will not generate text, it is useful for applications like personalization and search because by comparing embeddings the model will produce more relevant and contextual responses than word matching. To continue supporting best practices in the responsible use of AI, Titan FMs are built to detect and remove harmful content in the data, reject inappropriate content in the user input, and filter the models’ outputs that contain inappropriate content such as hate speech, profanity, and violence.
AI21 Jurassic
Released in 2021, Jurassic-1 is a 76-layer auto-regressive language model with 178 billion parameters. Jurassic-1 generates human-like text and solves complex tasks. Its performance is comparable to GPT-3.In March 2023, AI21 Labs released Jurrassic-2, which has improved instruction following and language capabilities.
Claude
Claude 3.5 Sonnet
Anthropic’s most intelligent and advanced model, Claude 3.5 Sonnet, demonstrates exceptional capabilities across a diverse range of tasks and evaluations while also outperforming Claude 3 Opus.
Claude 3 Opus
Opus is a highly intelligent model with reliable performance on complex tasks. It can navigate open-ended prompts and sight-unseen scenarios with remarkable fluency and human-like understanding. Use Opus to automate tasks, and accelerate research and development across a diverse range of use cases and industries.
Claude 3 Haiku
Haiku is Anthropic’s fastest, most compact model for near-instant responsiveness. Haiku is the best choice for building seamless AI experiences that mimic human interactions. Enterprises can use Haiku to moderate content, optimize inventory management, produce quick and accurate translations, summarize unstructured data, and more.
Cohere
Cohere has two LLMs: one is a generation model with similar capabilities as GPT-3 and the other is a representation model intended for understanding languages. While Cohere has only 52 billion parameters, it outperforms GPT-3 in many respects.
Stable Diffusion
Stable Diffusion is a text-to-image model that can generate realistic-looking, high-definition images. It was released in 2022 and uses diffusion models that use noising and denoising technologies to learn how to create images.The model is smaller than competing diffusion technologies, like DALL-E 2, so it does not need an extensive computing infrastructure. Stable Diffusion will run on a normal graphics card or a smartphone with a Snapdragon Gen2 platform.
BLOOM
BLOOM is a multilingual model with a similar architecture to GPT-3. It was developed in 2022 as a collaborative effort involving over a thousand scientists and the Hugging Space team. The model has 176 billion parameters and training took three and a half months using 384 Nvidia A100 GPUs. Although the BLOOM checkpoint requires 330 GB of storage, it will run on a standalone PC with 16 GB of RAM. BLOOM can create text in 46 languages and write code in 13 programming languages.
Hugging Face
Hugging Face is a platform that offers open-source tools to build and deploy machine learning models. It acts as a community hub, and developers can share and explore models and datasets. Membership for individuals is free, although paid subscriptions offer higher access levels. You have public access to nearly 200,000 models and 30,000 datasets.
What Are the Challenges with Foundation Models?
Foundation models can coherently respond to prompts on subjects they haven’t been explicitly trained on. But they have specific weaknesses.
Here are some of the challenges facing foundation models:
- Infrastructure requirements: Building a foundation model from scratch is expensive and requires enormous resources, and training may take months.
- Front-end development: For practical applications, developers need to integrate foundation models into a software stack, including tools for prompt engineering, fine-tuning, and pipeline engineering.
- Lack of comprehension: Although they can provide grammatically and factually correct answers, foundation models need help comprehending the context of a prompt. And they aren’t socially or psychologically aware.
- Unreliable answers: Answers to questions on certain subjects may need to be more reliable and sometimes appropriate, toxic, or incorrect.
- Bias: Bias is a distinct possibility as models can pick up hate speech and inappropriate undertones from training datasets. To avoid this, developers should carefully filter training data and encode specific norms into their models.
Related Reading
- LLM Security Risks
- What is an LLM Agent
- AI in Retail
- LLM Deployment
- How to Run LLM Locally
- How to Use LLM
- LLM Model Comparison
- AI-Powered Personalization
- How to Train Your Own LLM
Foundation Model vs LLM Insights for Better AI Decisions
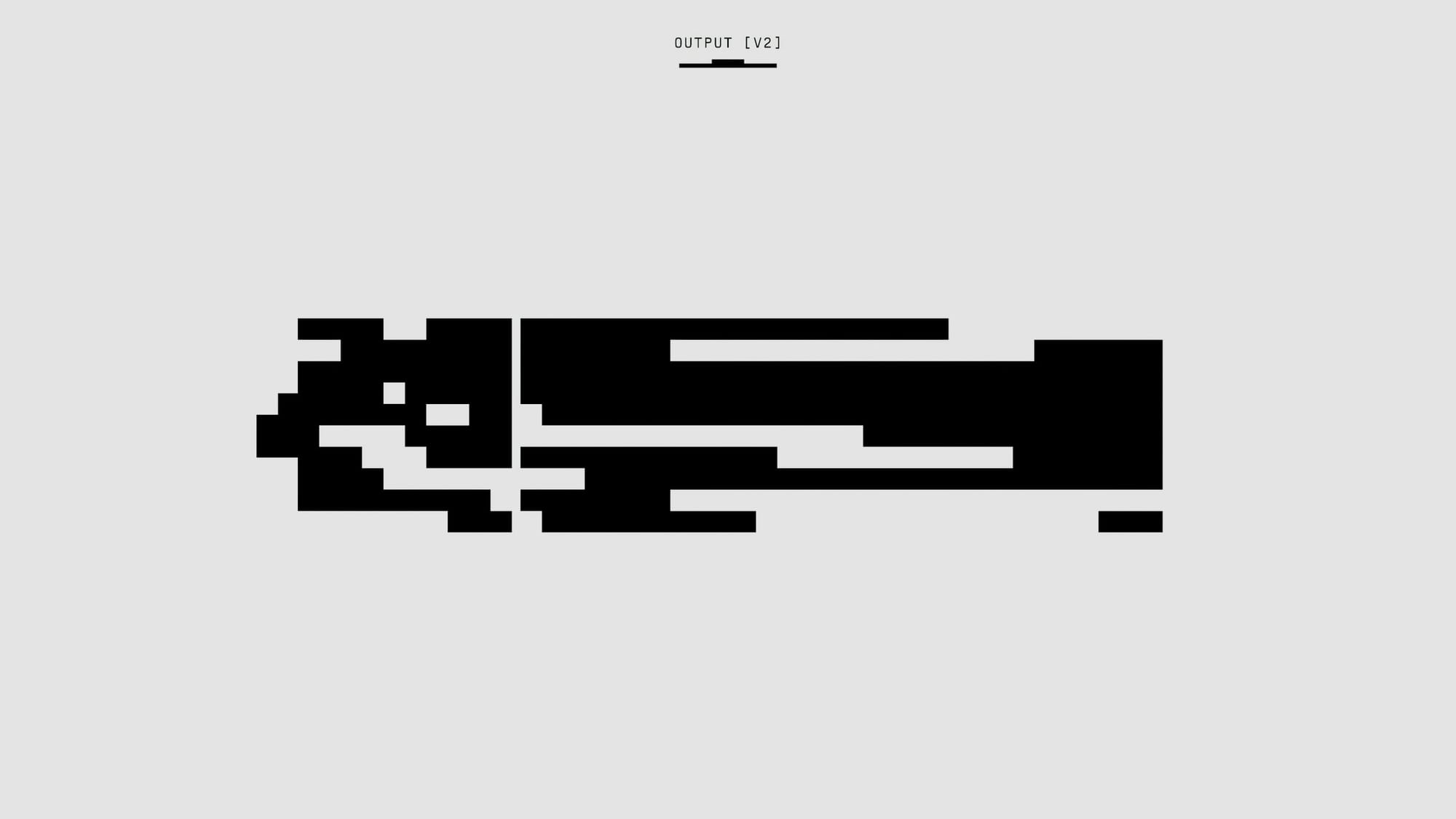
Foundation models, often called base models or pre-trained models, provide the architectural basis for more specialized models.
- These foundational models are trained on massive amounts of text data and learn to understand and generate human-like text.
- They are typically large in size and have a vast vocabulary.
- They have a general understanding of language but require further training tailored to specific tasks.
There are many other foundational models available, both proprietary and open-source. Examples of foundational models include:
- GPT-3
- BERT
- PaLM
- LLaMa
These models can handle a wide range of natural language processing tasks and can be fine-tuned for specific applications.
What are Large Language Models?
Large language models, or LLMs, are instances or specific use cases of foundational models. They are large language models based on a foundational architecture but are fine-tuned and specialized for generating human-like text in conversational interactions. LLMs like ChatGPT are designed for:
- Chatbots
- Virtual assistants
- Text-based dialog systems
They have undergone additional training to become more coherent, context-aware, and suitable for natural language conversations. LLMs have been fine-tuned on a wide range of dialog data, improving their ability to maintain context and generate appropriate conversation responses.
How Foundation Models and LLMs Are Different
The key differences between LLMs and FMs lie in their scope and application potential. While LLMs are specialized for text-based tasks, FMs are designed to handle multiple forms of data, making them more versatile in cross-domain applications.
FMs often require more complex training procedures and larger datasets to effectively learn from different modalities, whereas LLMs primarily focus on optimizing text data processing. This makes FMs potentially more powerful but also more resource-intensive in terms of data, computing power, and development time.
Applications and Benefits of LLMs
LLMs have found applications across a diverse range of industries, demonstrating their versatility and power.
- Healthcare sector: LLMs interpret patient data, assist in diagnosis, and even generate medical documentation —enhancing the efficiency and accuracy of healthcare services.
- Legal field: These models revolutionize document review and contract analysis, automating tasks that traditionally required extensive human labor.
- Customer service: LLMs power chatbots and virtual assistants, providing increasingly indistinguishable responses from human agents.
Their ability to process and understand large volumes of text unlocks insights from previously inaccessible data or too costly to analyze manually. This capability can lead to more informed decision-making and innovation. LLMs can be fine-tuned for specific tasks, allowing organizations to tailor the models to their unique needs, enhancing their utility and effectiveness.
Applications and Benefits of FMs
Foundational models have various applications across various industries, driving innovation and efficiency. For example:
- Healthcare: FMs analyze medical images, patient notes, and genetic information to assist in diagnosis and personalized treatment plans.
- Automotive industry: They contribute to developing autonomous vehicles by processing and interpreting real-time data from multiple sensors and cameras.
The benefits of FMs are extensive:
- Multimodal integration: FMs seamlessly integrate and interpret data from various sources, providing a holistic view of complex situations. This capability is especially valuable in fields like security and surveillance, where quick, accurate visual and textual data analysis is critical.
- Scalability: Their generalized nature allows FMs to be scaled across different tasks and domains without extensive retraining. This makes them cost-effective and adaptable solutions for businesses leveraging AI across multiple areas.
- Enhanced accuracy: By training on diverse data types, FMs often achieve higher accuracy in tasks involving complex data interpretations than models trained on single data types.
- Innovation: FMs encourage innovation by making experimenting with new AI applications easier. Industries such as entertainment and media utilize FMs for tasks like content generation, recommendation systems, and interactive customer experiences.
- Accessibility: FMs make powerful AI tools more accessible to nonexperts, enabling more users to develop custom applications without deep technical knowledge of AI or machine learning.
Key Differences Between Foundation Models and LLMs
The key differences between LLMs and FMs lie in their scope and application potential. While LLMs are specialized for text-based tasks, FMs are designed to handle multiple forms of data, making them more versatile in cross-domain applications. FMs often require more complex training procedures and larger datasets to effectively learn from different modalities, whereas LLMs primarily focus on optimizing text data processing. This makes FMs potentially more powerful and resource intensive in terms of data, computing power, and development time.
Related Reading
- How to Fine Tune LLM
- How to Build Your Own LLM
- LLM Function Calling
- LLM Prompting
- What LLM Does Copilot Use
- LLM Evaluation Metrics
- LLM Use Cases
- LLM Sentiment Analysis
- LLM Evaluation Framework
- LLM Benchmarks
- Best LLM for Coding
Choosing the Right Model for Your Needs
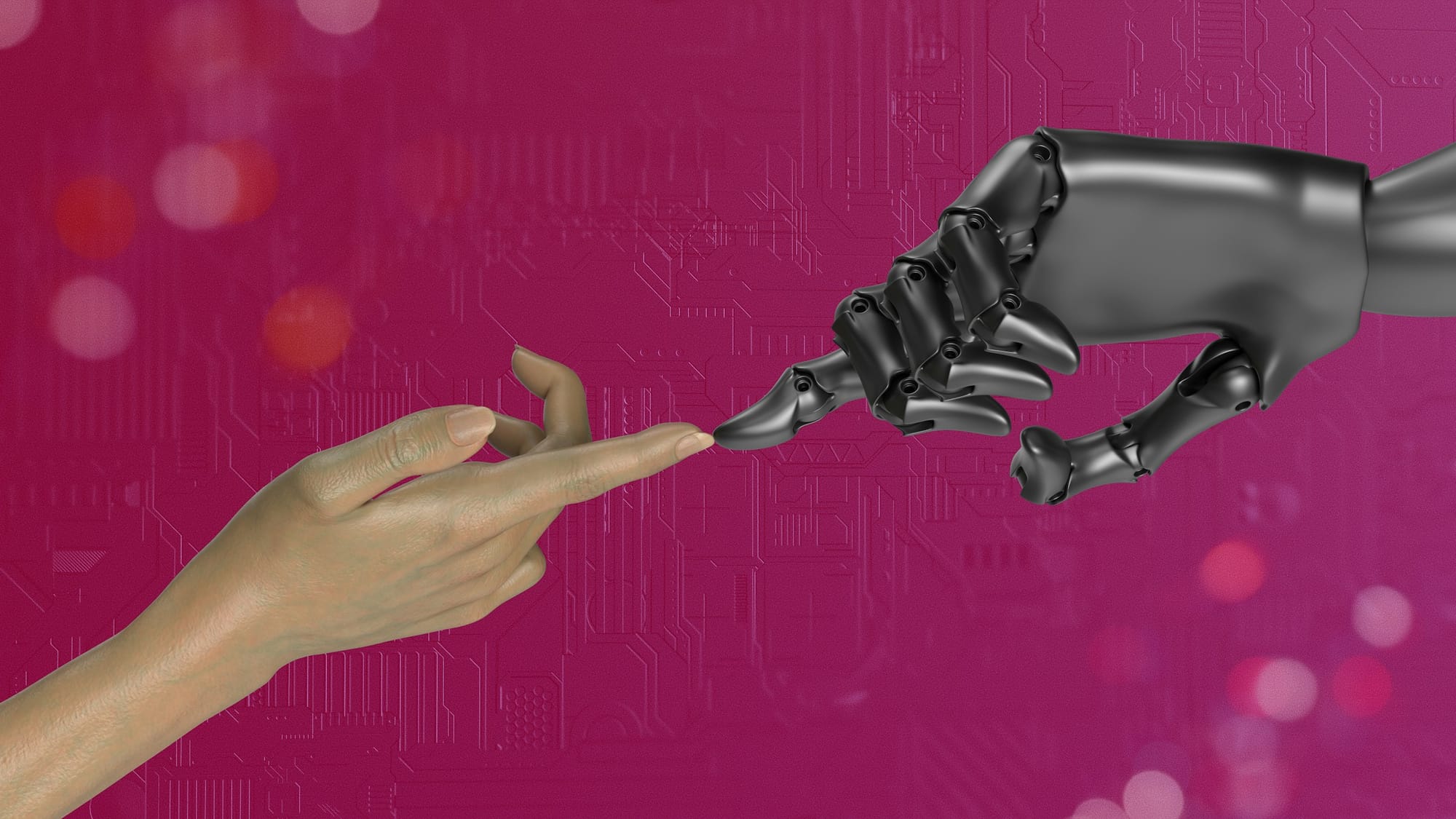
Data Types and Project Requirements: LLMs vs FMs
When your project focuses on text, you will benefit from the performance and accuracy of large language models. These models are trained to understand and generate human language and are particularly effective at tasks like natural language understanding, text generation, and language translation. LLMs excel in areas like:
- Content creation
- Chatbots
- Legal document analysis
Foundation models are more suitable if your project requires handling multiple data types—such as images, audio, and text. FMs are designed to integrate and interpret diverse data formats, making them excellent for complex applications like medical diagnostics involving imaging and notes, multimedia content analysis, or any scenario where insights need to be gleaned from various data sources.
Scope of Application: LLMs vs FMs
Large language models (LLMs) are highly specialized and offer depth in linguistic capabilities. If your project's success depends on deep language understanding and generation, LLMs could provide more refined outcomes. FMs offer a more versatile framework for broader applications that require flexibility across different types of data. They can adapt to various scenarios, reducing the need for multiple specialized models.
Computational and Financial Resources: LLMs vs FMs
Due to their multimodal nature, training and deploying foundation models generally demand more computational power and data than large language models. The cost associated with these resources can be significant, and managing such models is more complex. If resource constraints are a concern and if the project goals can be met with text-only analysis, choosing an LLM might be more practical and cost-effective.
Longevity and Scalability: LLMs vs FMs
Consider how future-proof and scalable the solution needs to be. Foundation models, with their ability to handle diverse data types and adapt to different tasks, might offer a longer lifespan, as they can easily be extended to new applications. This makes them a better choice for organizations investing in solutions that can evolve with emerging technologies and requirements.
Accuracy and Performance: LLMs vs FMs
FMs might offer superior performance in environments where integrating data types can lead to more accurate or insightful outcomes. In contrast, the specialized nature of LLMs might yield higher accuracy in purely text-based applications.
Related Reading
- LLM Quantization
- LLM Distillation
- LLM vs SLM
- Best LLM for Data Analysis
- Rag vs LLM
- ML vs LLM
- LLM vs Generative AI
- LLM vs NLP
Start Building GenAI Apps for Free Today with Our Managed Generative AI Tech Stack
Lamatic helps teams implement GenAI solutions that are fast, efficient, and production-ready. Our managed Generative AI Tech Stack automates workflows to eliminate tech debt and ensure reliable deployment for products that require quick AI integration. With Lamatic, you can build GenAI applications for free today.
GenAI Middleware: The Brains Behind GenAI Integration
GenAI middleware serves as a bridge between existing systems and Generative AI applications. The technology handles data exchange and automates workflows to streamline operations. Lamatic’s GenAI middleware takes the complexity out of integrating GenAI into business applications, providing teams with the tools to build custom, production-grade solutions that meet their specific needs.
Custom GenAI APIs: Tailored Connectivity for Your Business
Every business is unique, with its processes, workflows, and data. So it makes sense that GenAI solutions should be tailored to your organization. Lamatic’s custom GenAI APIs (GraphQL) allow for seamless GenAI integration into existing business applications, so you can avoid the headaches of using off-the-shelf solutions that don’t meet your requirements.
Low Code Agent Builders: Simplifying GenAI Development
Building GenAI applications from scratch requires a lot of technical know-how. Even configuring off-the-shelf solutions can be complicated. Lamatic’s low code agent builder simplifies the process, allowing teams to create GenAI applications with little to no coding. This intuitive tool comes pre-loaded with GenAI templates to help you get started quickly and customize your application to meet your unique business needs.
Automated GenAI Workflows: CI/CD for GenAI Applications
Like any software, GenAI applications will need updates and maintenance after deployment. With Lamatic’s automated GenAI workflows, you can ensure your applications remain bug-free and up-to-date with the latest features. Our CI/CD capabilities help streamline routine operations, automate testing, and reduce human error to help your team maintain production-ready applications.
GenOps: DevOps for GenAI
GenOps is the evolution of DevOps for Generative AI. Its goal is to help organizations minimize the technical complexities of integrating GenAI into business applications. Lamatic’s GenOps capabilities help teams streamline operations, automate workflows, and ensure reliable deployment to reduce tech debt and improve business productivity.
Edge Deployment via Cloudflare Workers: Fast and Secure GenAI Solutions
Running GenAI applications can require a lot of computing power, leading to latency issues that frustrate developers and end users. Lamatic’s edge deployment via Cloudflare Workers ensures your GenAI applications are responsive, reducing lag time and improving overall performance. With our solution, your applications can run on the edge, close to your users' location, to provide fast and secure GenAI solutions.
Integrated Vector Database: Weaviate
Weaviate is an open-source vector database that helps organizations store and manage unstructured data for AI applications. Lamatic’s managed tech stack comes with a pre-configured Weaviate database to help GenAI applications quickly retrieve relevant data in real-time. This allows for:
- Faster responses
- Improved accuracy
- Better overall performance