Data analysis has become critical in determining successful outcomes across various tasks and sectors. However, as data analysis methods become more sophisticated, many organizations need help to keep pace. For instance, in a recent survey, only 31% of respondents reported being satisfied with their organization’s current data analysis capabilities. Rapid artificial intelligence (AI) advancements, particularly large language models (LLMs), will likely boost data analysis outcomes. In particular, multimodal LLMs can sift through various data types to produce faster and more accurate actionable insights. This article will help you identify the “best LLM for data analysis” to enhance data analysis capabilities with minimal complexity and maximum impact.
One valuable tool to help you achieve your objectives is Lamatic’s generative AI tech stack. This solution streamlines identifying and selecting LLMs for data analysis so you can integrate the best platforms into your product and enhance performance with minimal fuss.
How LLMs Help with Data Analysis
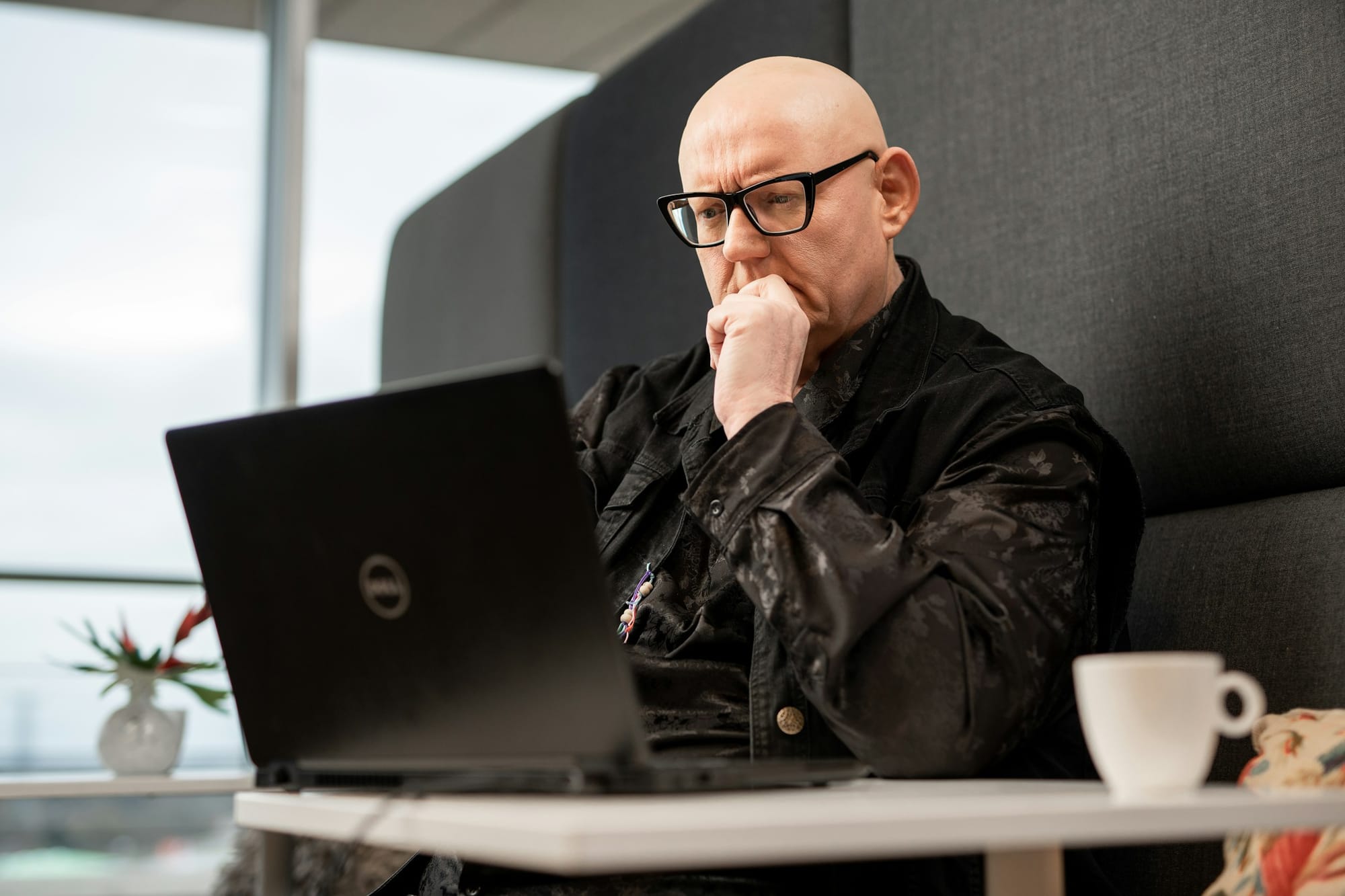
1. Unpacking Large Language Models and Their Role in Data Analysis
Large language models, or LLMs, are artificial intelligence that can understand and generate human language. They learn language skills by analyzing vast amounts of text data to recognize patterns and relationships. LLMs can:
- Summarize
- Translate
- Answer questions about text data, among other tasks
LLMs can change how we extract insights from data in their ability to process vast amounts of unstructured data, such as:
- Text documents
- Social media posts
- Customer reviews
- And more
This creates new possibilities for analyzing and understanding natural language data.
2. LLMs Change Data Analysis with New Approaches
LLMs bring a new level of sophistication to data analysis techniques. Traditional methods often rely on rule-based algorithms that require explicit instructions.
LLMs can independently learn patterns and relationships within the data. This means that analysts can focus on asking the right questions and let the LLM handle the complex task of understanding the context and extracting meaningful insights.
3. The Benefits of Using LLMs for Data Analysis
Using LLMs in data analysis has several advantages. LLMs can process unstructured data, which makes up a significant portion of available information. Organizations can better understand customer sentiment by:
- Analyzing text data
- Identifying emerging trends
- Extracting critical information
LLMs may struggle with complex reasoning or domain-specific knowledge, and organizations should be cautious about potential hallucinated answers. LLMs are also good at complex language tasks such as text classification and sentiment analysis. They can accurately categorize documents, identify sentiments, and provide valuable insights for decision-making.
LLMs can create human-like text, making them useful for content creation, chatbots, and automated customer service. This helps businesses improve their operations and customer interactions. LLMs can be fine-tuned for specific industries or use cases, allowing organizations to leverage their domain expertise and tailor the models to their unique needs. This customization makes the insights from LLMs more relevant and actionable, leading to better decision-making and planning.
4. Real-World Applications for LLMs in Data Analysis
What exactly can LLMs do for data analysis? Let’s take a look at several labor-intensive knowledge tasks companies can streamline.
- Customer Sentiment Analysis: LLMs can detect nuances in textual data and interpret the semantics of written content at a massive scale.
- Sales Analytics: Instead of relying on dashboards and SQL queries, business analysts can interact with CRM, ERP, and other data sources via a conversational interface.
- Market Intelligence: Combining textual and numerical data allows business analysts to identify developing trends, patterns, and potential growth opportunities.
- Sustainability Reporting: LLMs can facilitate data extraction and management and can be configured for automatic document generation and validation.
- Due Diligence: LLMs help better identify risks, inconsistencies, and critical insights that leaders need to know to make better deals.
- Fraud Investigation: With LLMs, analysts get high-precision anomaly detection capabilities and conversational assistance in investigation efforts.
Related Reading
- LLM Security Risks
- What is an LLM Agent
- AI in Retail
- LLM Deployment
- How to Run LLM Locally
- How to Use LLM
- LLM Model Comparison
- AI-Powered Personalization
- How to Train Your Own LLM
Top 22 Platforms for the Best LLM for Data Analysis
1. Alteryx
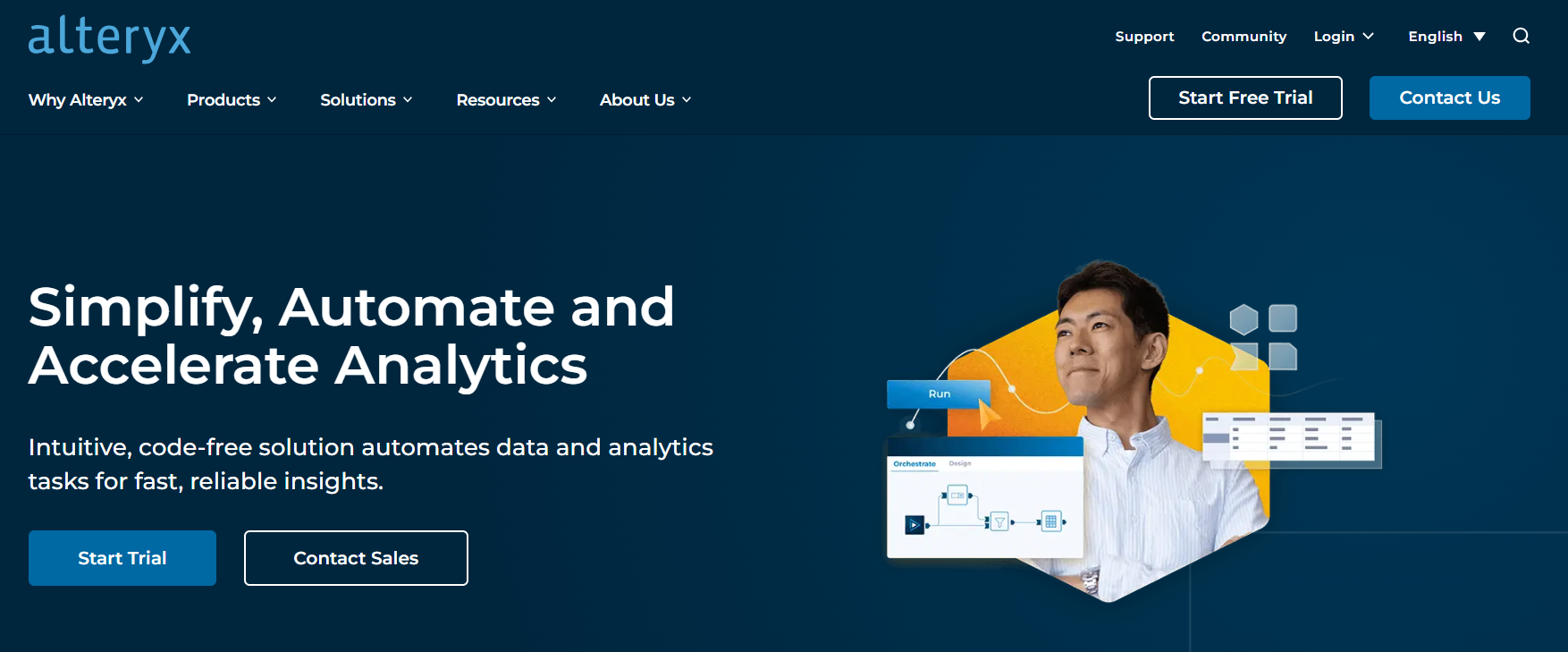
The Alteryx platform now incorporates a no-code AI studio to let users create analytics apps using custom business data. Data can then be queried through a natural language interface, which includes access to models such as OpenAI’s GPT-4. Built on its Alteryx AiDIN engine, the platform differentiates itself from competitors with a user-friendly approach to predictive analytics and insight generation.
A key feature is its Workflow Summary Tool, which interprets complex workflows into simple-to-understand natural language explanations and summaries. You can also specify how you want your reports to be output, for example, in email or PowerPoint formats, to target your desired audience. Alteryx is a great choice for analysts and SME users looking for an all-around platform for creating custom analytics apps and generating predictive insights.
2. Microsoft Power BI
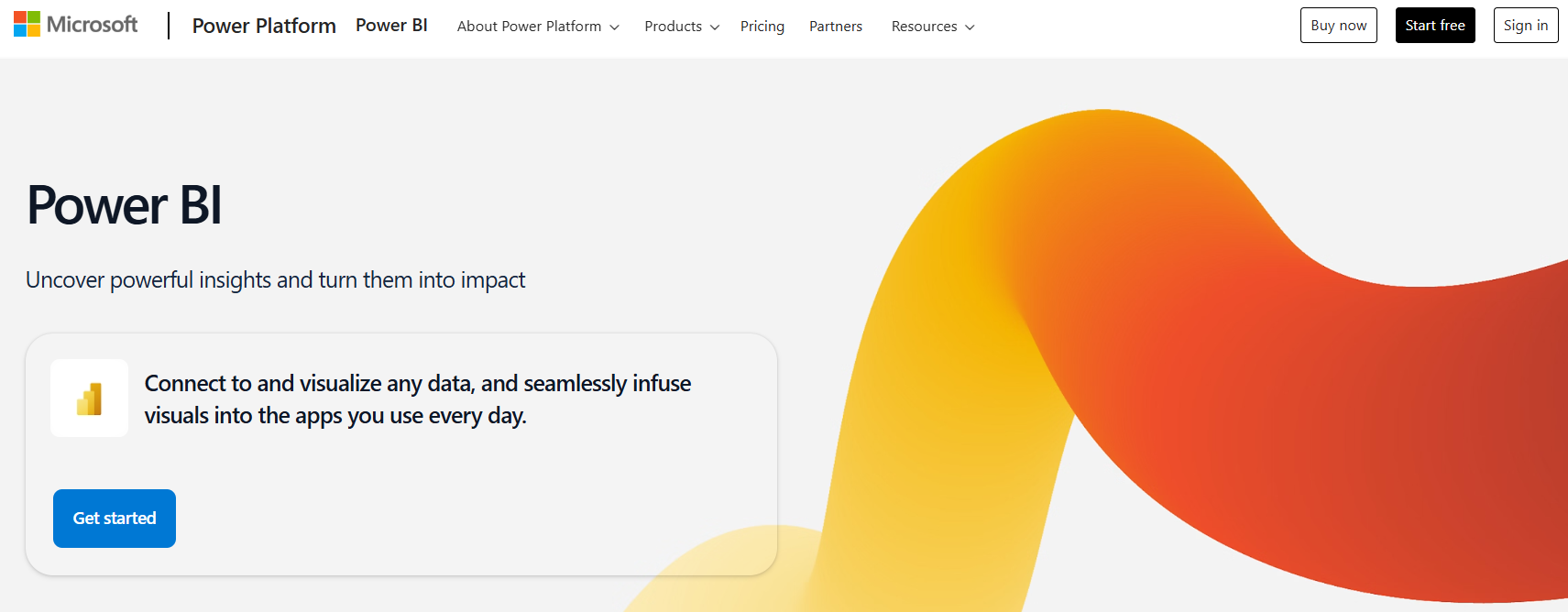
This industry-standard analytics package has been enhanced with the power of generative AI. It harnesses Microsoft’s Co-Pilot technology and models developed with OpenAI, including customized versions of its powerful GPT-4 models.
The platform integrates numerous technologies, including Microsoft’s Fabric AI-powered analytics framework and Azure Synaps, which allows it to integrate seamlessly with data warehouse and other big data technologies like Apache Spark. This creates an end-to-end analytics solution suitable for the largest enterprise workloads, enabling anything from simple analytics to building and deploying your machine learning models on Azure. It’s a good choice for enterprise users needing flexible cloud integrations and dealing with extensive data workloads.
3. Tableau Pulse
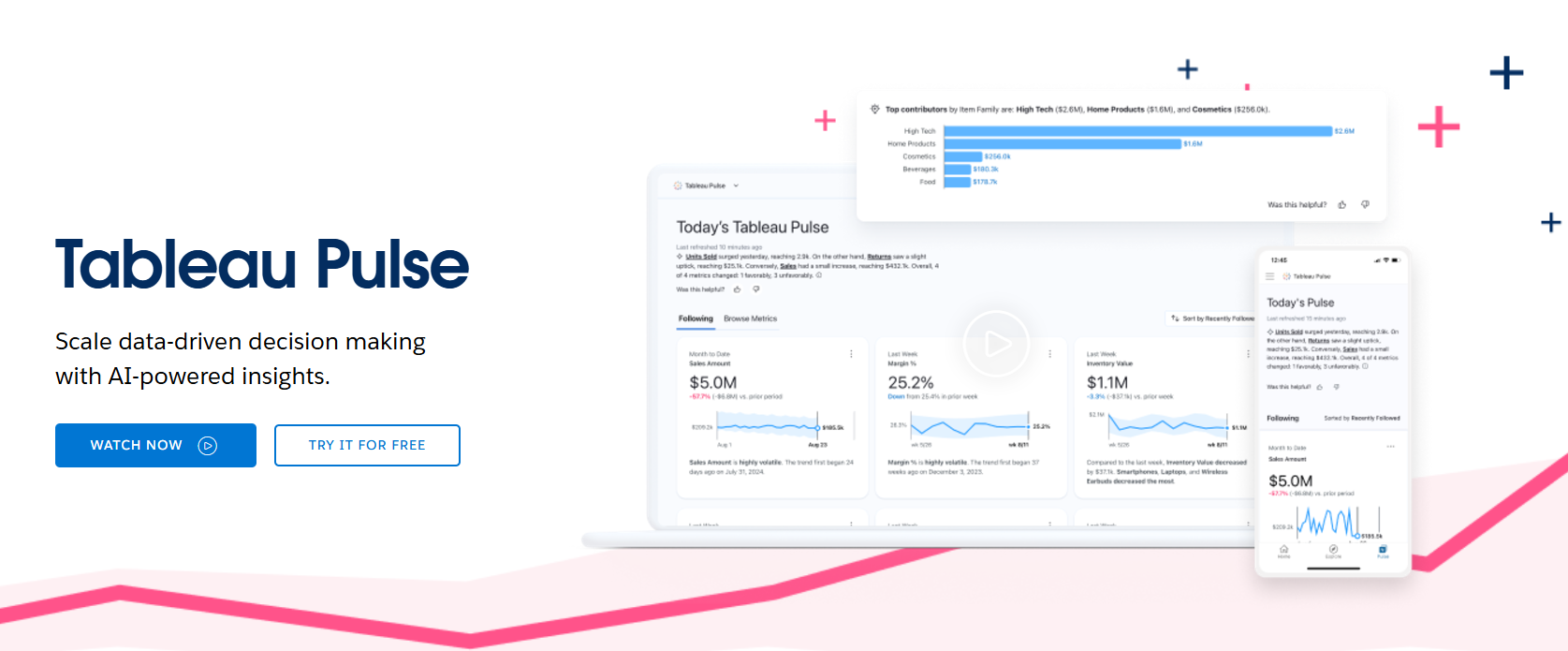
Tableau is one of the world’s most popular data visualization tools and now features Tableau Pulse, built on Salesforce’s Einstein models, for AI-powered insights. Its Insights Platform allows automated analysis of datasets, extracting insights and trends in natural language while generating visualizations.
The package aims to facilitate data-driven decision-making and improve productivity by giving business users access to in-depth analytics at their fingertips. Security and ethics are also important when working with data, So Salesforce has integrated its Einstein Trust Layer guardrails with Tableau Pulse for peace of mind. Ideal for decision-makers who want to create user-friendly and detailed visualizations.
4. Qlik
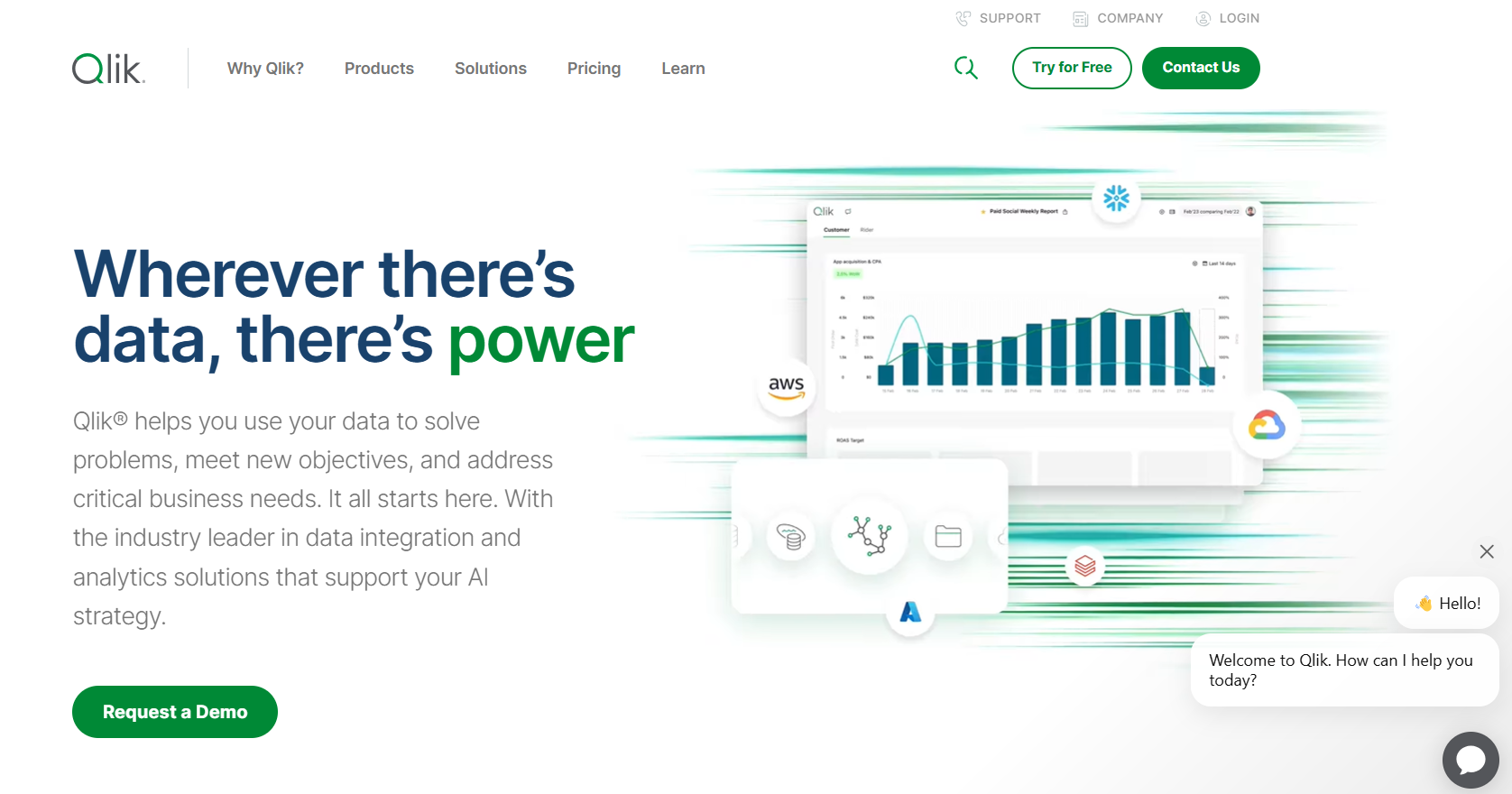
Another established analytics and data platform that now lets users embed generative AI analytics content into their reports and dashboards through its Qlik Answers assistant. It features automated summaries of key data points, natural language reporting and various integrations with third-party tools and platforms.
Emphasis is placed on explainability to ensure that insights can always be supported with sources and citations. The Qlik platform is particularly useful for users who want to analyze large volumes of unstructured data, such as text or videos. Thanks to Qlik Answers, this can all be done with natural language.
5. Sisense AI
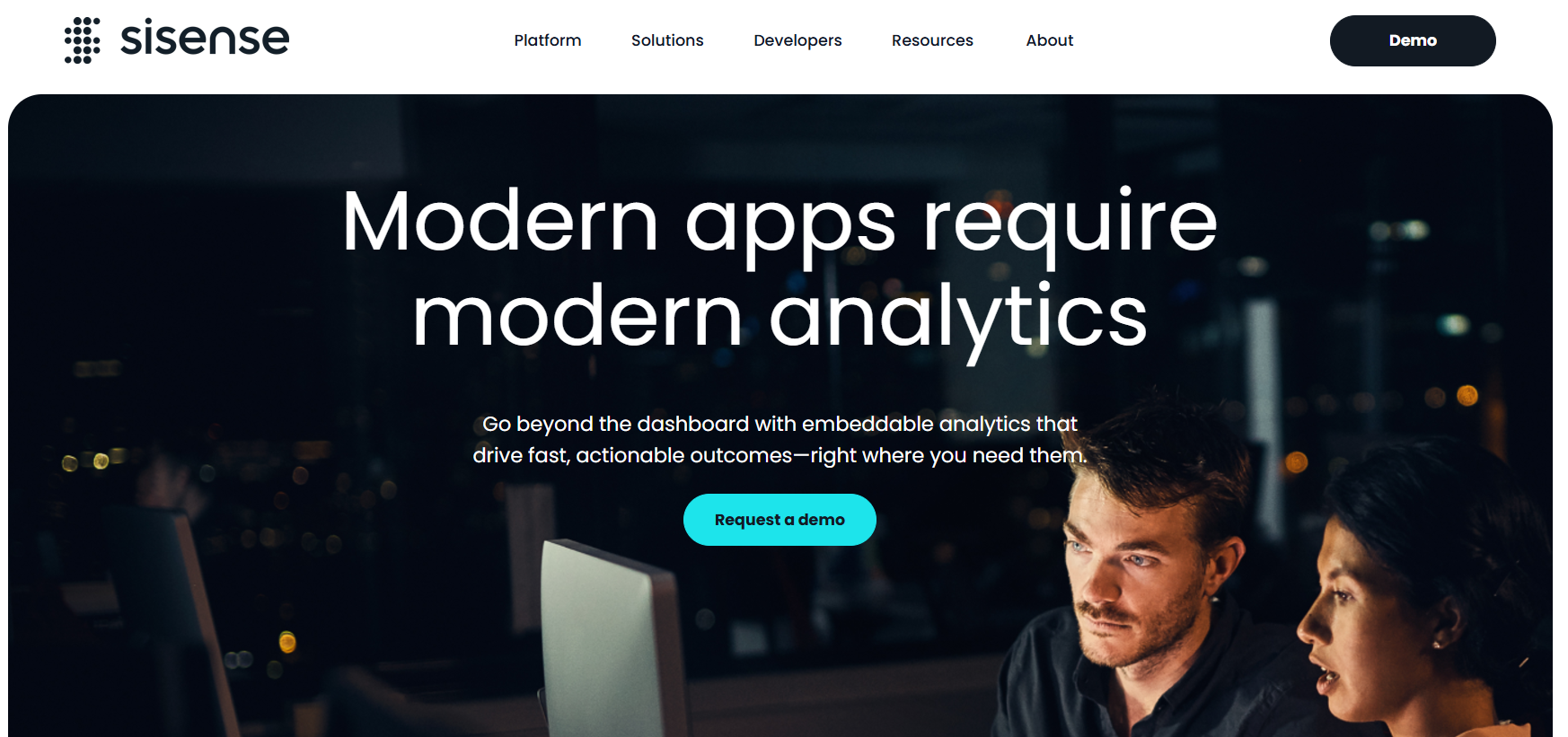
Sisense lets you embed conversational analytics directly into your BI tools and applications. This simplifies automating processes, including data preparation and building analytics dashboards and reports.
Sisense is a widely used platform with a user base that spans research scientists to business analysts. It is designed to make analytics accessible to anyone, regardless of their level of expertise. This makes it useful for anyone needing a powerful analytics engine that can be deployed quickly and efficiently.
6. Guanaco
Generative Universal Assistant for Natural-language Adaptive Context-aware Omnilingual Outputs, or Guanaco, is a popular LLM developed by researchers at the University of Washington. It is based on Meta's LLaMA model but is much more efficient to train and run. Guanaco can be trained on a single GPU in one day, requiring only 5 GB of GPU RAM. This makes it much more accessible to researchers and developers who do not have access to large computing clusters.
7. BLOOM
BLOOM (Bayesian Large Language Model) is an open-source, multilingual, and multimodal LLM developed by BigScience, an international collaboration of over 1,000 researchers.
BLOOM is the first multilingual LLM trained in complete transparency and is available for free under the Apache 2.0 license. It is trained on an enormous dataset of text and code and has over 176 billion parameters.
8. Vicuna
Vicuna is a large language model (LLM) developed by the LMSYS project. It is based on Meta's LLaMA models and fine-tuned on a dataset of user-shared conversations collected from ShareGPT.
According to preliminary evaluation using GPT -4 as a judge, Vicuna-13B outperforms other models like LLaMA and Stanford Alpaca in more than 90% of instances. Vicuna-13B also achieves more than 90% quality of OpenAI ChatGPT and Google Bard.
9. Alpaca
With around 26K GitHub stars, Alpaca is another popular LLM fine-tuned on Meta’s LLaMA 7B model. It was trained on 52K instruction-following demonstrations generated in the style of self-instruct using text-davinci-003. The shorter outputs of text-davinci-003 are reflected in Alpaca's responses, often shorter than ChatGPT's.
However, the model exhibits a few common language model problems, including toxicity, hallucination, and stereotypes. For instance, Alpaca incorrectly claims that the capital of Tanzania is Dar es Salaam, which was the capital until 1974 when Dodoma replaced it.
10. GPT4All
GPT4All is an ecosystem that trains and deploys robust and customized large language models that run locally on consumer-grade CPUs. The GPT4All model aims to be the best instruction-tuned assistant-style language model that any person or enterprise can freely use, distribute, and build on.
This model is a 3GB 8GB file you can download and plug into the GPT4All open-source ecosystem software. Nomic AI developed and trained the GPT4All-J and GPT4All-J-LoRA datasets.
11. OpenChatKit
OpenChatKit is an open-source large language model (LLM) developed by Together, LAION, and Ontocord under an Apache-2.0 license that includes source code, model weights, and training datasets.
It is based on the EleutherAI GPT-NeoX-20B model and fine-tuned on a massive dataset of user-shared instructions and conversations. OpenChatKit is designed to be a powerful tool for creating chatbots and other natural language processing applications.
13. Flan-T5
FLAN-T5 model is an encoder-decoder model that has been pre-trained on various unsupervised and supervised tasks for which each task is converted into a text-to-text format. Flan-T5 checkpoints have also been publicly released, which achieve strong few-shot performance even compared to larger models, such as PaLM 62B.
The Flan-T5 model excels in various NLP tasks, such as question-answering, text classification, and language translation. FLAN-T5 is designed to be highly configurable, enabling developers to modify it to suit their particular requirements. Google’s Flan-T5 is available via five pre-trained checkpoints:
- Flan-T5 small
- Flan-T5-base
- Flan-T5-large
- Flan-T5-XL
- Flan-T5 XXL.
14. GPT-2
GPT-2 (Generative Pre-trained Transformer 2) is a cutting-edge open-source large language model (LLM) developed by OpenAI, taking the world by storm. With a staggering 1.5 billion parameters, GPT-2 represents a significant advancement in natural language processing capabilities.
It has gained widespread attention due to its ability to generate consistent and contextually relevant text. From news articles to creative writing, GPT-2 can produce human-like content that captivates readers. Its power lies in its understanding of context and its consistency throughout lengthy text passages. With its fine-grained control, users can influence the generated output, making it a versatile tool for various applications.
15. GPT-3
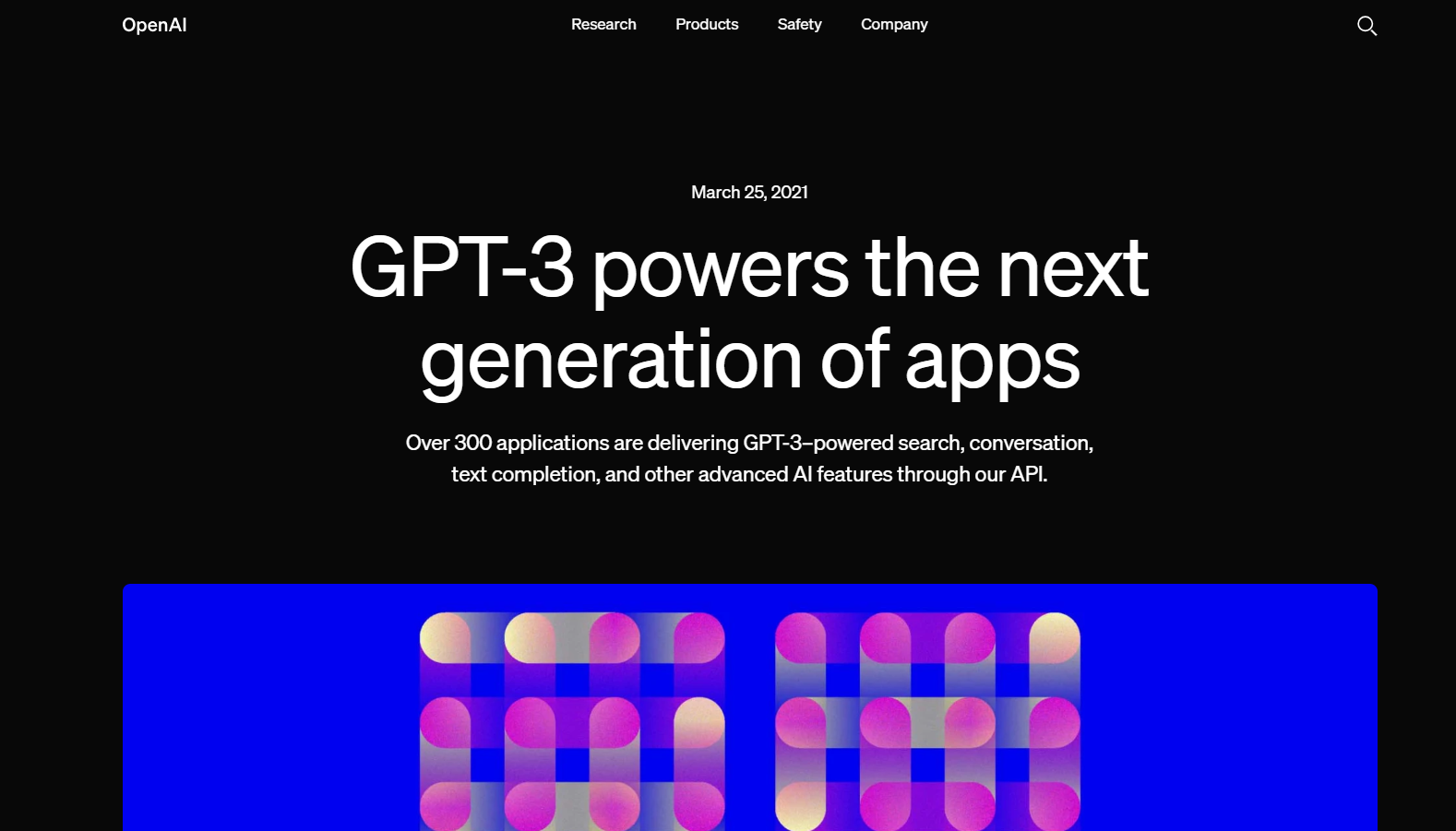
GPT-3 (Generative Pre-trained Transformer 3) is an advanced open-source Large Language Model (LLM) developed by OpenAI. It is renowned for its remarkable ability to generate contextually relevant human-like text across various tasks and domains.
With 175 billion parameters, the vast capacity of GPT-3 for understanding and generating human-like language has revolutionized the field of natural language processing, setting new benchmarks for LLM performance. GPT-3 is also estimated to be 116 times larger than its predecessor, GPT-2.
16. Dolly
Dolly is a popular large language model (LLM) introduced by Databricks in 2023. It is based on the Transformer architecture and has 12 billion parameters, making it one of the largest LLMs. Dolly was trained on a massive dataset containing 15,000 high-quality human-generated prompt/response pairs designed explicitly for instruction tuning large language models.
This gives Dolly a wide range of knowledge and allows it to perform various tasks, such as text generation, question answering, and code generation. Dolly is already being used by multiple companies, including Databricks, IBM, and Amazon, and it is also used in several research projects.
17. RoBERTa
RoBERTa (Robustly Optimized BERT approach) is an advanced open-source large language model (LLM) that builds upon BERT's success and enhances its performance. Developed by Facebook AI, RoBERTa focuses on pre-training models to understand language's deep contextual representations.
With 160 GB of unlabeled text data (10 times more than BERT) and 6 billion parameters, RoBERTa's pre-training process involves absorbing vast linguistic knowledge. This intensive learning provides RoBERTa with a deep understanding of language patterns and contextual relationships, thus making it a powerhouse for processing and analyzing human-like text. Its robustness and versatility have made RoBERTa a go-to choice for cutting-edge language applications.
18. T5
Another popular open-source LLM is the Google-developed T5 (Text-to-Text Transfer Transformer) Large Language Model (LLM). With a whopping 11 billion parameters, T5 is one of the largest and most powerful language models ever created. What sets T5 apart is its unique approach to transforming various language tasks into a text-to-text format, enabling it to handle multiple tasks using a single unified framework.
Unlike traditional models designed for specific tasks, T5 is trained to convert text inputs into text outputs, making it highly versatile. With its large-scale pre-training on diverse data sources, T5 has achieved great performance across various language tasks, making it a powerful tool for solving complex natural language processing problems.
19. XLNet
XLNet is another popular open-source Large Language Model (LLM) that has gained popularity in natural language processing. Introduced by researchers at Google AI, XLNet is based on the Transformer architecture and uses an innovative permutation-based training approach. Unlike traditional LLMs, XLNet considers all possible permutations of words within a sentence during training, allowing it to capture bidirectional context effectively. This unique training approach enables XLNet to overcome the limitations of previous models and achieve high-level performance on various language tasks.
XLNet has surpassed previous models on multiple benchmarks, including the GLUE benchmark, where it scored 89.8, outperforming BERT and other popular LLMs. With its robust pre-training and fine-tuning features, XLNet excels in text classification, language generation, and document ranking tasks.
20. Transformer-XL
Developed by researchers at Google AI, Transformer-XL is another commonly used open-source large language model (LLM) that addresses traditional Transformers' limitations when handling long-range dependencies. It introduces a unique architecture that enables capturing context beyond fixed-length segments.
Using recurrence mechanisms, such as memory states and relative positional encodings, Transformer-XL can efficiently process and understand sequences of arbitrary lengths up to 80% longer than conventional models. This allows the model to perform well in tasks requiring long-range dependencies, such as document understanding, language modeling, and machine translation.
21. FALCON
Falcon (or Falcon 40B) is a large language model developed by the Technology Innovation Institute (TII) in Abu Dhabi. It was introduced in May 2023 and is one of the most powerful LLMs available. Falcon has 40 billion parameters and is trained on a massive high-quality text and code dataset, including 1,000 billion tokens from the RefinedWeb enhanced with curated corpora.
Falcon even comes with Instruct versions called Falcon-7B-Instruct and Falcon-40B-Instruct, which are fine-tuned on conversational data and can be worked with directly to create chat applications.
22. ELECTRA
ELECTRA is an open-source Large Language Model (LLM) built on Tensorflow that presents an innovative approach to pre-training transformer-based models. ELECTRA introduces a new “Discriminator-Generator” training method that optimizes efficiency and performance. Instead of predicting the original masked tokens, ELECTRA trains a discriminator to differentiate between real and replaced tokens generated by a generator model. This approach allows ELECTRA to generate high-quality results while using only 10-20% of the pre-training time and computational resources compared to previous models. ELECTRA has gained much attention in the natural language processing community with its unique training approach.
23. DeBERTa
DeBERTa is an open-source large language model (LLM) developed by Microsoft Research Asia. It is designed to enhance the performance and efficiency of transformer-based models. With its unique approach, DeBERTa introduces novel techniques to address challenges in natural language processing tasks.
By incorporating disentangled attention mechanisms and eliminating the need for task-specific architectures, DeBERTa achieves top results across various language understanding benchmarks. Its innovative design and advanced features make DeBERTa a valuable tool in the data science industry.
Selecting the Best LLM for Data Analysis: Key Factors to Consider
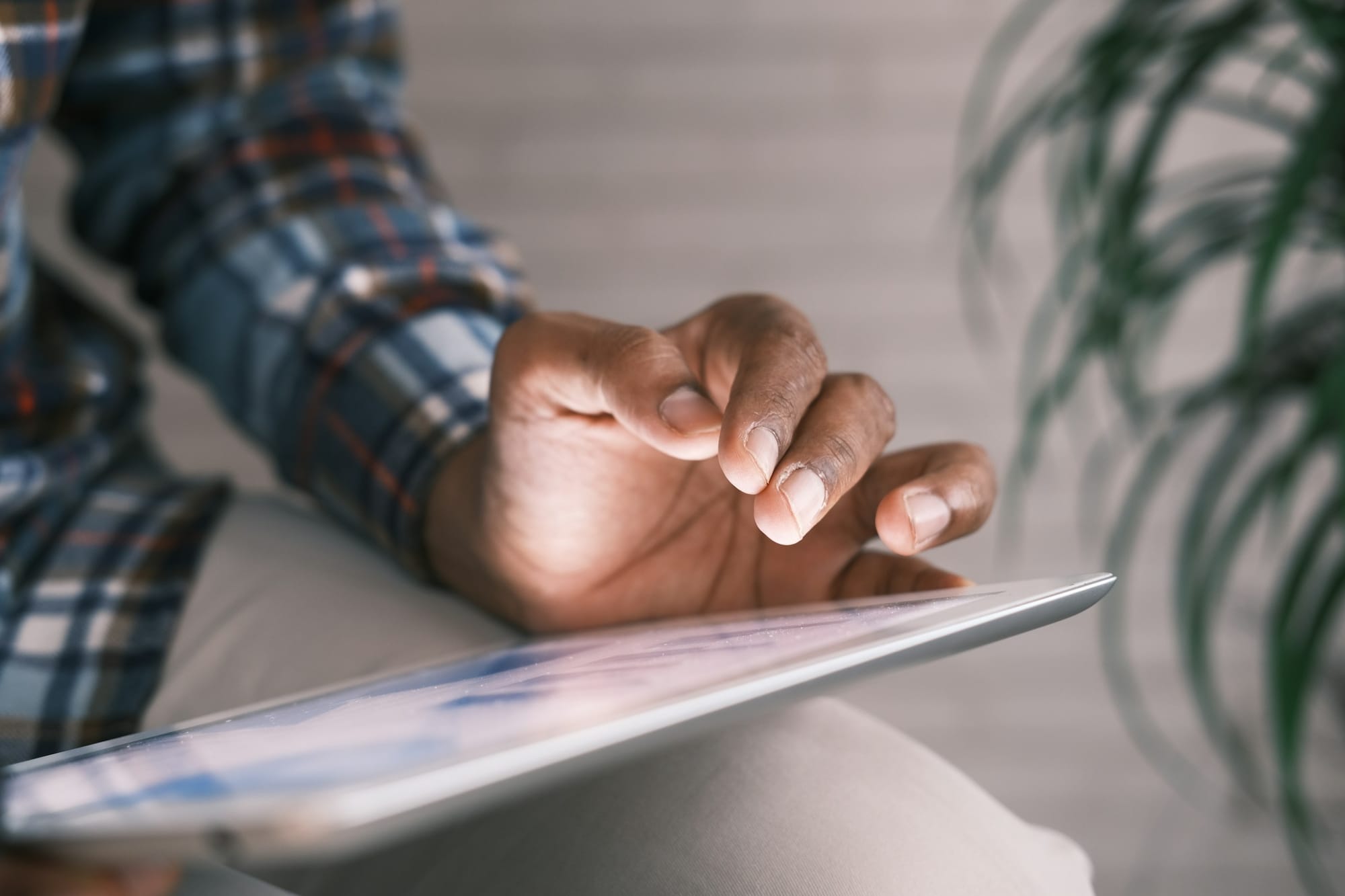
When selecting the best LLM for data analysis, it is crucial to consider the specific requirements of your project and the capabilities of different models. Here are some key factors to keep in mind:
Model Capabilities
Some LLMs can handle both text and images, which can be beneficial for data analysis that involves visual data.
Multi-modal Support
Some LLMs can handle both text and images, which can be beneficial for data analysis that involves visual data.
Tool Calling
Certain models support tool calling, enhancing their functionality in AI-agent scenarios.
Configuration Options
AnythingLLM provides flexibility in configuring LLMs:
- System LLM: This is the default model used unless a workspace-specific model is defined.
- Workspace LLM: You can set specific LLMs for different workspaces, allowing for tailored responses based on the analysis context.
- Agent LLM: For AI agents, ensure the selected LLM supports the necessary functionalities for effective data analysis.
Supported Providers
AnythingLLM supports a variety of LLM providers, making it easy to find the right fit:
- Built-in (default): The default model that requires minimal setup.
- Ollama: Known for its robust performance in data-related tasks.
- LM Studio: Offers advanced features for data manipulation and analysis.
- Local AI: Ideal for users who prefer local processing.
- KobaldCPP: A versatile option for various data analysis needs.
Top LLMs For Data Analysis
Here’s a roundup of what we believe to be the most useful tools for anyone wanting to apply generative AI to data analytics, whether enthusiastic amateurs or experts in the field.
Related Reading
- How to Fine Tune LLM
- How to Build Your Own LLM
- LLM Function Calling
- LLM Prompting
- What LLM Does Copilot Use
- LLM Evaluation Metrics
- LLM Use Cases
- LLM Sentiment Analysis
- LLM Evaluation Framework
- LLM Benchmarks
- Best LLM for Coding
How to Use LLM for Data Analysis
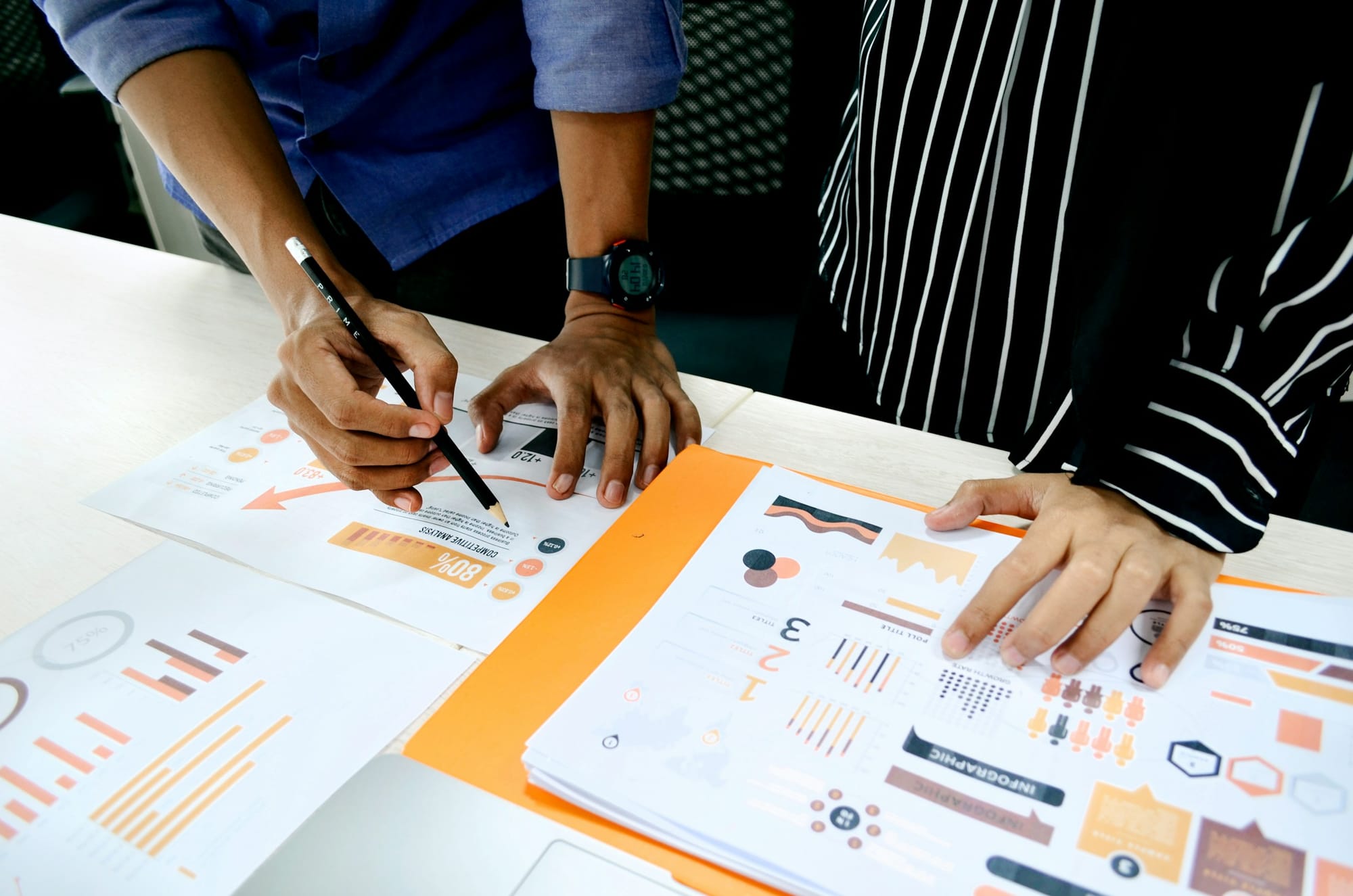
1. Data Collection: Where the Magic Begins
Data is the foundation of any LLM analysis. The process begins by gathering relevant data from:
- Databases
- Sensors
- User interactions
- Online repositories
Your data can come in various forms, including:
- Text
- Images
- Numerical data
Using LLMs for data analysis works best when incorporating diverse data types, so consider casting a wide net in your search.
2. Data Cleaning and Preparation: Getting the Data LLM-Ready
Once you collect your data, clean it by removing any irrelevant or erroneous information. This step involves filtering out noise, correcting errors, and organizing the data into a structured format suitable for analysis. High-quality, clean data are crucial for accurate LLM performance.
3. Training the LLM Model: Feeding the Beast
Input your cleaned data into the LLM to train it. This involves feeding the model large amounts of data to learn:
- Patterns
- Relationships
- Structures
Use libraries like:
- TensorFlow
- PyTorch
- Hugging Face's Transformers
To facilitate this process.
4. Fine-Tuning the Model: Tweaking for Peak Performance
Fine-tune the model to improve its performance on your specific dataset. Adjust the hyperparameters and perform additional training iterations to enhance accuracy and efficiency.
5. Evaluating the Model's Performance: Checking the Scorecard
Evaluate the trained model using various metrics such as:
- Accuracy
- Precision
- Recall
- F1 score
This step ensures that your model performs well on unseen data and provides reliable predictions.
6. Making Predictions: Putting Your LLM to Work
The trained and fine-tuned model will be applied to new data to generate predictions and insights. This step allows you to utilize the model for practical applications, such as:
- forecasting trends;
- identifying patterns;
- making data-driven decisions.
Common Mistakes to Avoid
While LLMs are a powerful tool, it's crucial to know common pitfalls to avoid inaccurate results. Some common mistakes include:
- Failing to check for multicollinearity among independent variables;
- Overfitting the model by including too many independent variables;
- Ignoring the assumptions and limitations of LLMs.
Proper model validation and interpretation are crucial in ensuring the reliability and validity of your linear regression analysis. By addressing these common mistakes and understanding the nuances of LLMs, you can make informed decisions based on your data analysis results.
Interpreting Results from LLM Analysis: Understanding the Output
Once you complete your LLM analysis, it's time to interpret the results and make sense of the findings. Let's explore how to understand and use LLM output to make data-driven decisions.
Understanding LLM Output
The output from LLM analysis provides valuable information about the relationship between the variables in your model. It includes:
- Coefficients
- P-values
- Odds ratios
- Goodness-of-fit measures
By analyzing this output, you can identify significant variables and understand their impact on the dependent variable. Examining the confidence intervals around the coefficients can offer additional insights into the precision of the estimates. A narrower confidence interval indicates more precise estimates, while a wider interval suggests greater uncertainty. This information is crucial for assessing the reliability of the results and determining the robustness of the relationships identified in the analysis.
Making Data-Driven Decisions with LLM
LLM analysis equips you with insights that can guide your decision-making process. By understanding the relationships between variables, you can:
- Identify key drivers
- Uncover hidden patterns
- Predict outcomes
This knowledge empowers you to make informed decisions and optimize your strategies.
Leveraging the results of LLM analysis in a practical setting involves more than just understanding the statistical outputs. It requires translating the findings into actionable strategies that can drive business decisions. By integrating the insights from LLMs into the decision-making process, organizations can enhance their competitive advantage, improve performance, and achieve their goals more effectively.
Optimizing Your LLM Analysis: Taking It Up a Notch
To optimize your LLM analysis, consider the following techniques:
- Feature Engineering: Create or transform new variables to improve predictive power. This technique allows you to extract more meaningful information from your data and enhance the performance of your LLM model.
- Regularization: Apply techniques like L1 or L2 regularization to handle multicollinearity and reduce overfitting. Regularization helps prevent your model from becoming too complex and generalizes new data well.
- Interaction Terms: Include interaction terms to capture complex relationships between variables. By considering the combined effects of two or more variables, you can uncover synergistic or antagonistic relationships that may significantly impact your analysis.
By incorporating these optimization techniques into your LLM analysis, you can elevate your data analysis to new heights and gain deeper insights into your data.
Future Trends in LLM Data Analysis: What Lies Ahead?
LLM data analysis, like any other field, continues to evolve. As technology advances and new methods emerge, it's essential to stay updated. Let's take a glimpse into the future of LLM data analysis and explore some emerging trends:
- Bayesian LLMs: Combining the power of Bayesian statistics with LLMs for more accurate predictions. Bayesian LLMs allow you to incorporate prior knowledge and update your beliefs as new data becomes available, resulting in more robust and reliable analysis.
- Big data LLMs: Applying LLMs to massive datasets to uncover hidden insights and trends. With the explosion of data in today's digital age, traditional analysis techniques may need to be revised. Big data LLMs enable you to handle large volumes of data and extract valuable information that can drive strategic decision-making.
- Machine learning integration: Incorporating machine learning algorithms with LLMs to enhance predictive modeling. Machine learning techniques like random forests or gradient boosting can complement LLMs by capturing non-linear relationships and handling complex data structures.
As these future trends continue to shape the field of LLM data analysis, staying abreast of the latest developments will be crucial for data professionals seeking to stay ahead of the curve.
Leverage LLMs for Your Data Analysis Tasks
Leveraging large language models (LLMs) for data analysis is a game-changer. It offers the ability to process and interpret vast datasets with remarkable precision and efficiency. Following a structured approach can unlock powerful insights that drive informed decision-making.
Start Building GenAI Apps for Free Today with Our Managed Generative AI Tech Stack
Lamatic offers a managed Generative AI tech stack that includes:
- Managed GenAI Middleware
- Custom GenAI API (GraphQL)
- Low-Code Agent Builder
- Automated GenAI Workflow (CI/CD)
- GenOps (DevOps for GenAI)
- Edge Deployment via Cloudflare Workers
- Integrated Vector Database (Weaviate)
Lamatic empowers teams to rapidly implement GenAI solutions without accruing tech debt. Our platform automates workflows and ensures production-grade deployment on edge, enabling fast, efficient GenAI integration for products needing swift AI capabilities.
Start building GenAI apps for free today with our managed generative AI tech stack.