Retail has long been about creating the perfect shopping experience to delight customers and keep them returning for more. But today, retail is in the midst of a major transformation as artificial intelligence technology better understands human behavior and preferences. Now, retailers can use AI in retail to improve the customer experience, optimize internal operations, and boost overall business performance. This blog will examine how generative AI works, explore its many valuable applications, and reveal best practices for successfully scaling multimodal LLM in your retail business for optimal results.
Lamatic's generative AI tech stack can help you easily reach your goals by providing the tools and strategies to enhance functionality, streamline operations, and drive growth with minimal complexity.
What is the Role of AI in Retail?
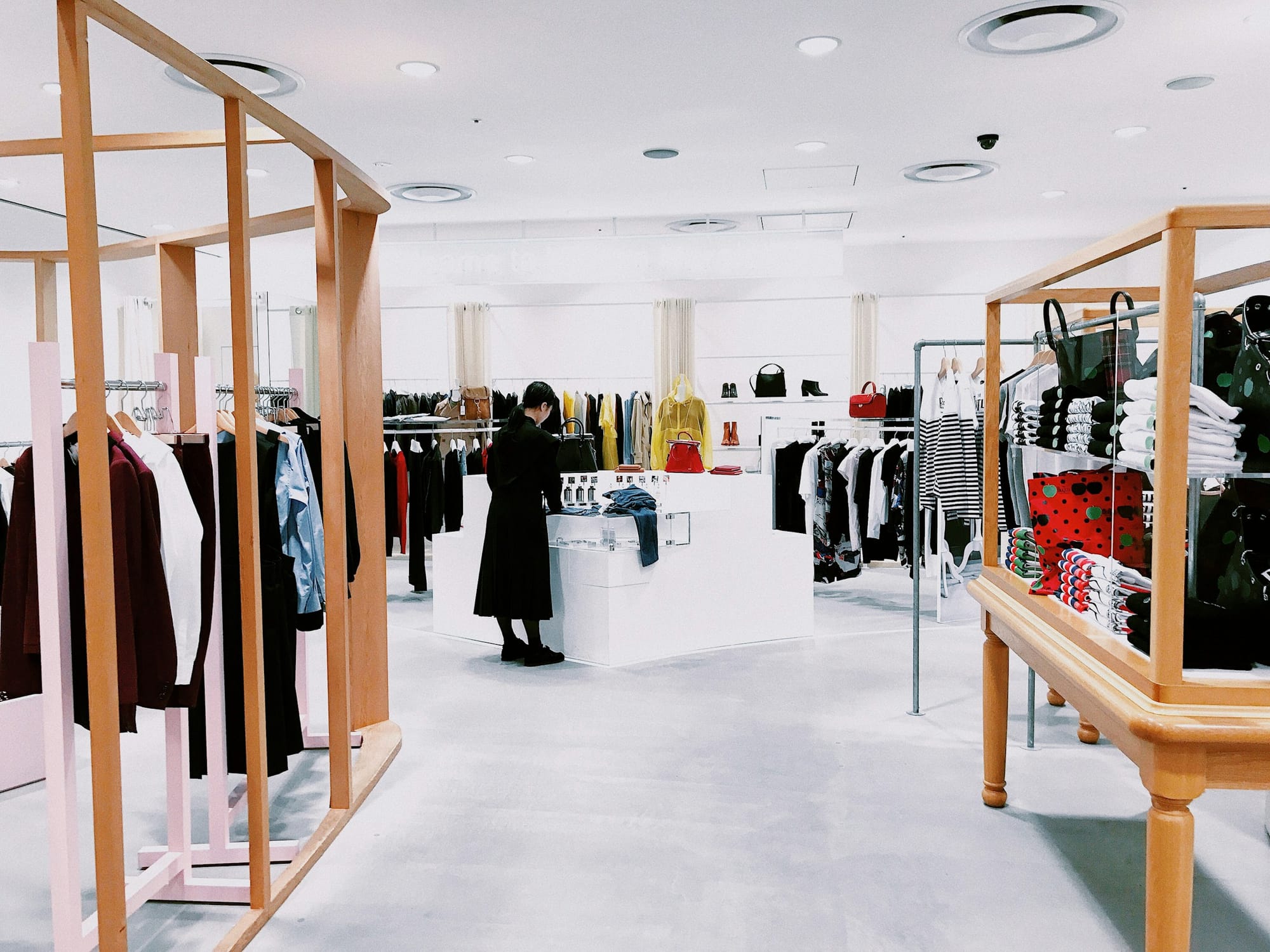
Gone are the days of one-size-fits-all recommendations and bland product descriptions. Today's retail industry is undergoing a revolution fueled by artificial intelligence. Generative AI is revolutionizing shopping and product interactions by enabling:
- Personalized experiences tailored to individual preferences.
- Engaging interactions that captivate and retain customers.
- Efficient processes for businesses and consumers
With the global AI in retail market size projected to hit a staggering $45.74 billion by 2032, it's clear that AI is here to stay. But what AI solutions drive this growth, and how can retailers leverage them to boost sales, delight customers, and stay ahead of the curve? Let's explore the exciting possibilities.
The Role of AI in Retail
Even though AI adoption is still in its early stages, 87% of retailers have already deployed the technology in at least one area of their business. 60% of retail companies plan to boost their AI investments shortly; by 2025, 80% of retail executives expect their organizations to adopt AI automation.
AI: A Retail Game-Changer
As AI’s presence grows, retailers face a crucial choice: embrace it to unlock strategic applications and boost performance or risk falling behind. Ignoring AI is no longer an option, mainly if customers are willing and enthusiastic about using GenAI tools to enhance their online shopping experiences.
Consumers Embrace AI-Powered Shopping
For example, 87% of shoppers who have tried a GenAI tool are excited about AI's positive impact on their shopping experiences. Seventy-three percent of consumers are open to AI-powered chatbots for customer service, and 60% have already used virtual assistants to purchase through voice commands.
The Financial Benefits of AI in Retail
In essence, AI represents a pivotal turning point for the retail industry. Those who embrace its potential will thrive in the new era of retail, while those who resist risk becoming relics of the past. It’s been found that 69% of retailers have reported an increase in their annual revenue due to adopting AI, and 72% of those already using AI say they experienced a decrease in operating costs.
McKinsey forecasts that artificial intelligence could increase retail sales by $310 billion by improving digital customer interactions. That sounds impressive, doesn’t it?
Main Reasons Behind the Increasing Use of AI in the Retail Industry
The main reasons behind the increasing use of AI in the retail industry include:
- Supply chain and logistics
- Improving products
- Guiding customers in stores
- Analyzing payments and pricing
- Managing inventory
- Customer Relationship Management (CRM)
AI Use Cases in Retail
AI brings many opportunities to the retail industry beyond improving customer experience and generating unique content. It streamlines business operations and enables you to maintain a competitive edge. Take a look at some noteworthy AI applications in retail below.
Personalized Product Recommendations
As more consumers expect brands to understand their preferences, AI is becoming a major game-changer. 75% of retail customers are likelier to buy again from brands that personalize their experience. That being said, it shouldn’t be a surprise why companies decide to deploy AI solutions on a larger scale than ever before.
AI-Powered Personalization: A Revenue Booster
AI allows you to analyze large amounts of customer data, such as:
- Browsing and purchase history
- Items added to a cart
- Demographics
Personalized Recommendations
This level of analysis means that you can offer product recommendations tailored to your customer’s tastes and preferences. This simplifies shopping and makes it more enjoyable, guiding customers toward better purchase decisions. Brands leveraging advanced digital personalization tools see revenue jump by 6% to 10% faster.
AI Retail Use Case: Amazon
Amazon stands as the best example of using GenAI to create personalized recommendations. As an omnichannel retailer, it customizes its homepage for each customer using AI-powered analytics and data collected on their purchasing behavior, preferences, wish lists, and items in their cart.
An Amazon Case Study
Amazon analyzes past and real-time data by gaining valuable insights into its customers’ preferences. This allows the company to create highly personalized marketing campaigns that enhance customer experience and satisfaction. According to McKinsey, recommendations drive 35% of purchases on Amazon.
Virtual Try-Ons
One typical challenge customers often face when shopping for clothes and other wearables online is ensuring the perfect fit. Fortunately, the days of relying solely on static images to imagine how a product might look or fit are gone.
AI-Powered Virtual Try-Ons
Virtual try-ons powered by Artificial Intelligence (AI) and augmented reality (AR) technologies enhance the shopping experience by allowing customers to:
- Visualize how clothes and accessories will look on them.
- Test makeup products for suitability and appearance.
- Preview furniture items to see how they fit and complement their spaces.
The outcome is reduced product return rates and increased customer satisfaction.
AI Retail Use Case: Warby Parker
Warby Parker offers a virtual try-on experience for glasses and sunglasses on its website and through its mobile app. Its system combines AI and AR technologies to analyze customers’ facial features and virtually overlay eyewear frames. Using the customer’s device camera, the tool creates a 3D map of their face to ensure accurate placement and size comparison.
This allows customers to try on various styles and colors of frames in real-time, with the system providing instant rendering. As customers tilt, turn, and move their heads, the virtual frames adjust dynamically, mimicking natural movement and light reflection.
Intelligent Product Search
Why do consumers leave an online store without buying anything? The top reason is that they can’t find what they want. According to Nielsen Norman, 17% and 20% of users give up after just one unsuccessful search attempt.
These abandoned shopping trips have a big impact, costing U.S. retailers an estimated $330 billion in 2021 due to search-related problems. With AI, product search becomes different. How? AI-powered site search can grasp context and intent, enabling shoppers to input any keywords and get precise, relevant search results. As it processes extensive data and learns about each shopper’s preferences, the results become personalized to each individual over time.
AI Retail Use Case: Zalando
Zalando uses complex AI algorithms to analyze vast customer data: past purchases, browsing behavior, and saved items. This allows the platform to personalize search results for each customer and display products most likely to interest them based on their unique preferences.
Personalized Search Experiences with Dynamic Filters
Zalando uses dynamic filters which evolve based on a user’s behavior. For example, if a customer consistently applies size or color filters, these options may be pre-selected or highlighted in future searches.
A New Era of Product Discovery
There’s also a search bar that understands natural language (NL) queries beyond just keywords. Customers can describe desired styles, materials, or occasions (e.g., “black ankle boots for winter”), and the AI interprets the intent, delivering relevant product suggestions.
Enhanced Experience with Visual Product Search
Studies show that 74% of US and UK online shoppers need help finding the products they want. This highlights the need for better search capabilities, with visual search emerging as a promising solution. It’s estimated that the global visual search market will rise to as much as $33 billion by 2028.
Visual search technology employs image recognition and AI algorithms to enable users to search for products using images instead of text. For users who might need to become more familiar with specific search terms or type the wrong search terms into the search bar, this makes it easier and faster to find relevant products.
The Rise of Visual Search
Providing a more intuitive, engaging, and efficient way to discover and buy products becomes important, especially in a world where 90% of information transmitted to the human brain is visual. 62% of millennials prefer visual search over any other technology.
AI Retail Use Case: ASOS
Asos offers a Style Match feature on its app, which employs visual search technology. With Style Match, customers can snap a photo of an item or upload an image from their library to initiate the search process. ASOS’ machine learning algorithms analyze visual information like color and patterns to find a match and provide personalized recommendations to customers.
Hands-Free Shopping Through Voice Search
AI brings another opportunity to retail—voice search, which enables consumers to browse products and make purchases without lifting a finger. They can use voice commands via smart speakers like:
- Google Assistant
- Apple’s Siri
- Amazon’s Alexa
- Other voice-activated platforms
Many retailers also develop voice-enabled shopping experiences directly integrated with their apps or websites.
A New Frontier in Retail
Recent statistics show that 55% of consumers use voice search to find products, and 44% have already used it to add items to their shopping lists. In the USA, 33.2 million consumers use voice search to make purchases. Why? Simply because it proves useful, accessible to individuals with disabilities, and often much faster.
AI Retail Use Case: Walmart
Walmart has also tapped into the potential of voice commerce, thereby enhancing the shopping journey for its customers. Through Google Assistant or Siri, customers can effortlessly:
- Add items to their Walmart online shopping carts
- Create shopping lists
- Initiate checkout using voice commands
What sets Walmart apart is its focus on user convenience. Shoppers can easily access information about their previous purchases and preferences, which enables quick reorders and reduces the need for repetitive tasks. What’s more, Walmart’s voice commerce platform integrates with its physical stores, offering customers the option of in-store pickup or delivery.
Advanced Description Generation
The greater the number of products you sell, the longer it might take to write unique descriptions for each one. How about using AI‑powered tools for this task? While not being professional copywriters, AI might quickly generate unique, compelling, and SEO-optimized descriptions, capturing details important to customers.
The Power of Accurate Product Descriptions
Accurate e-commerce descriptions are crucial for setting expectations, building trust, and ensuring customer satisfaction. They provide comprehensive information about product features, specifications, and usage, helping customers make informed decisions and reducing returns and complaints.
AI Retail Use Case: H&M
H&M, a well-known fashion retailer, has implemented an AI " Cherry " system to write product descriptions for its online store. This system analyzes images of clothing items and uses natural language processing to generate descriptions.
Human writers then review and edit these descriptions. This approach has helped H&M streamline its content creation process and provide consistent and accurate product descriptions for its customers.
Dynamic Pricing and Promotions
Regarding retail, price is a key determinant of purchase decisions. Surveys reveal that 90% of shoppers plan to switch brands, look for lower prices, and reduce spending because of higher prices. More than half are already doing so. Dynamic pricing and promotions are crucial for attracting customers, maximizing profits, and staying competitive. AI pricing engines continuously optimize prices using:
- Data
- Algorithms
- Feedback loops
As a result, retailers can adjust prices based on their:
- Promotional activities
- Pricing history
- Product range
- Other data
This is not just by chain, region, or store but by individual.
AI Retail Use Case: Amazon
Amazon employs a dynamic pricing tool called Amazon Price Optimizer to adjust its product prices several times daily. The process considers factors like:
- Demand
- Competitor pricing
- Sales volume
- Product availability
By doing so, Amazon remains competitive while maximizing profits. According to reports, this solution has led to a 5% increase in sales and a 2% profit improvement for Amazon.
Personalized Customer Experience with AI-Powered Loyalty Programs
Consumers crave connections with brands, and 80% are likelier to stick with a brand that offers a loyalty program. Thus, offering relevant products, recommendations, and rewards tailored to their needs builds trust and loyalty. And those retailers who deliver and win their customers’ hearts (and wallets). This translates to higher sales, increased customer satisfaction, repeat purchases, and higher lifetime value. Imagine a world where your favorite store:
- Remembers your preferences
- Suggests items you’ll love
- Rewards you for your loyalty
That’s the power of personalization and loyalty programs, a key ingredient for retail success.
AI Retail Use Case: Starbucks
Starbucks might be an excellent example of how a customer loyalty program can make a tremendous difference for the business. Starbucks uses AI to study customers:
- Past purchases
- Preferences
- Time of day they visit
This information helps the Starbucks Rewards program offer personalized rewards like discounts on favorite drinks or exclusive deals. This personal touch makes customers more loyal and encourages them to spend more and visit more often. Starbucks rewards members are five times more likely to see a Starbucks daily.
The company's loyalty program increased active membership in the US by 15% year over year in 2023, reaching nearly 31 million members. These loyalty members account for 41% of Starbucks’ sales in the USA.
Streamlined Customer Service with AI Chatbots
AI-powered chatbots and virtual assistants are changing how customer service works in retail. These intelligent conversational agents can answer customer questions, give product information, and deliver assistance round the clock. 64% of consumers prefer interacting with a chatbot rather than waiting for a human agent.
By using chatbots, stores can reply to customer messages quickly, improving customer satisfaction. Since 75% of customers engage through multiple channels throughout their journey, AI ensures consistent, high-quality service across all platforms.
AI Retail Use Case: eBay
One excellent example of chatbots in online shopping is eBay ShopBot. It’s a virtual shopping assistant available on Messenger. By quickly answering all shopper questions and providing instant replies, this chatbot helps save time for everyone. No more tedious scrolling through eBay or ticking boxes; ShopBot offers friendly conversations and direct links to products you’re interested in.
Increased Security with Fraud Detection
As customers slowly shift from in-store to online purchasing, fraudulent activities in transactions, orders, and deliveries are on the rise. About 34% of American consumers report being potential fraud victims, and e-commerce businesses lose an average of $48 billion to fraud annually.
AI as a Fraud Fighter: Protecting Retailers and Consumers
Retailers use AI-based tools for fraud detection and prevention to tackle this issue. These tools analyze data to identify suspicious transactions by spotting unusual behaviors and inconsistencies in product descriptions. By quickly flagging and blocking such transactions, AI helps prevent fraud and ensures a safer shopping experience for everyone involved.
AI Retail Use Case: PayPal
PayPal relies on an AI-powered system called Deep Learning Fraud Detection to detect and prevent fraud in financial transactions. These tools analyze user behavior, transaction patterns, and various parameters related to user identification and credit card information, including address verification.
AI’s Role in Detecting Sophisticated Fraud Tactics
They also examine patterns to identify users with multiple accounts or those trying to exploit proxy servers for various purchases. These tools continuously adapt and improve their fraud detection capabilities through machine learning. PayPal reports that the AI-powered system has helped them reduce losses from fraud by 25%.
Enhanced Inventory Management and Demand Forecasting
Keeping the right balance of stock to meet customer demand, both in-store and online. What retailer would like to overstock or run out of products? Probably none. They should have enough items to fulfill orders quickly but not so many that their storage space gets crowded with products that aren’t selling. Nevertheless, retailers can’t have everything under control at all times. That’s when AI technology comes in.
AI-Driven Inventory Management
By analyzing historical data, customer preferences, and competitor information, AI helps retailers make smarter decisions when ordering inventory. Meanwhile, AI-powered tools such as cameras and sensors enable the real-time monitoring of inventory levels. This optimizes supply chain and delivery processes and helps retailers avoid the dreaded “Out of Stock” status, which may drive customers away.
AI Retail Use Case: Lowe’s
Lowe’s, the American home improvement retailer, leverages AI to revolutionize inventory management and enhance customers' shopping experience. It uses small cameras strategically positioned on shelves in key areas of the store, like the light bulb section.
Ensuring Product Availability
These cameras keep an eye on stock levels in real time. When they notice a gap on the shelf, they send a quick alert to the store’s devices. This helps staff know when to restock from the stockroom promptly. By using AI this way, Lowes ensures that customers always find what they need and make shopping more efficient and enjoyable.
The Benefits of AI in Retail
AI can help retailers reduce labor, shrinkage, and waste costs and generate incremental revenue by suggesting better assortment mixes in stores, producing more precise recommendations for online shoppers, and helping ensure retailers have enough stock of popular items. Read on for more information on those and other benefits.
More Profitable Product Mixes
AI can help retailers make better decisions about what inventory to hold and in what quantities by analyzing a store's historical sales trends and third-party data, such as weather forecasts and calendars of upcoming local events.
Forecasting Demand
Sporting goods retail planners don’t need to be rocket scientists to know they should stock up on Lionel Messi jerseys when Inter Miami comes to town, but AI might suggest they also stock up on jerseys for Messi’s Spanish teammate Jordi Alba because there’s a large Spanish population in that city.
AI can also suggest opportunities for retailers to substitute private label or generic alternatives to manufacturers’ brands and help determine when those alternatives would be accretive to earnings rather than cannibalize existing sales.
Automated Item Attribution
Retailers can use generative AI to interpret and summarize product attributes from long, detailed descriptions provided by manufacturers, extract the most salient features, and write pithy descriptions that appeal to factors that drive customer buying decisions. This can apply as easily to furniture descriptions in online catalogs as it can to esoteric new grocery items.
Better E-Commerce Recommendations
AI can quickly consider a customer’s ordering history to offer more personalized cross-sells. For example, it can suggest that a female customer shopping for sandals also buys youth-sized crew socks—not to go along with the sandals, of course, but because it detected that she previously bought children’s sneakers.
Supplemented Labor
Retailers can deploy robots with embedded AI capabilities to help with mundane tasks, such as
counting items on shelves to ensure they’re correctly stocked or monitoring floors for spills and cleaning them up as necessary.
With AI running on a handheld tablet, the people doing those tasks can be redeployed to help customers find what they’re seeking or even suggest additional items they can buy.
Reduced Shrinkage
According to the National Retail Federation, US retailers lose more than $110 billion a year to inventory shrinkage, which occurs when inventories decrease due to:
- Shoplifting
- Vendor fraud
- Employee theft
- Other non-sales reasons
A Vigilant Eye on Retail Fraud and Inventory
Retailers can use AI, often in conjunction with sensors and other technologies at the point of sale, to detect when customers take a more expensive item than the one they scan or if a cashier deliberately undercharges a customer, often called sweethearting.
Retailers can also use AI to help ensure that products don’t wither on the vine because they’ve suddenly become unpopular and thus unsellable.
Better Location Decisions
Location, location, location! Retailers can use AI to run many simulations using an almost incalculable number of variables—the area of competing retailers, population density, rent, and other costs, climate and demographic information, and many others—to make better decisions about where to place new stores locations.
Related Reading
- LLM Security Risks
- What is an LLM Agent
- LLM Deployment
- How to Run LLM Locally
- How to Use LLM
- LLM Model Comparison
- AI-Powered Personalization
- How to Train Your Own LLM
How to Scale Gen AI in Retail
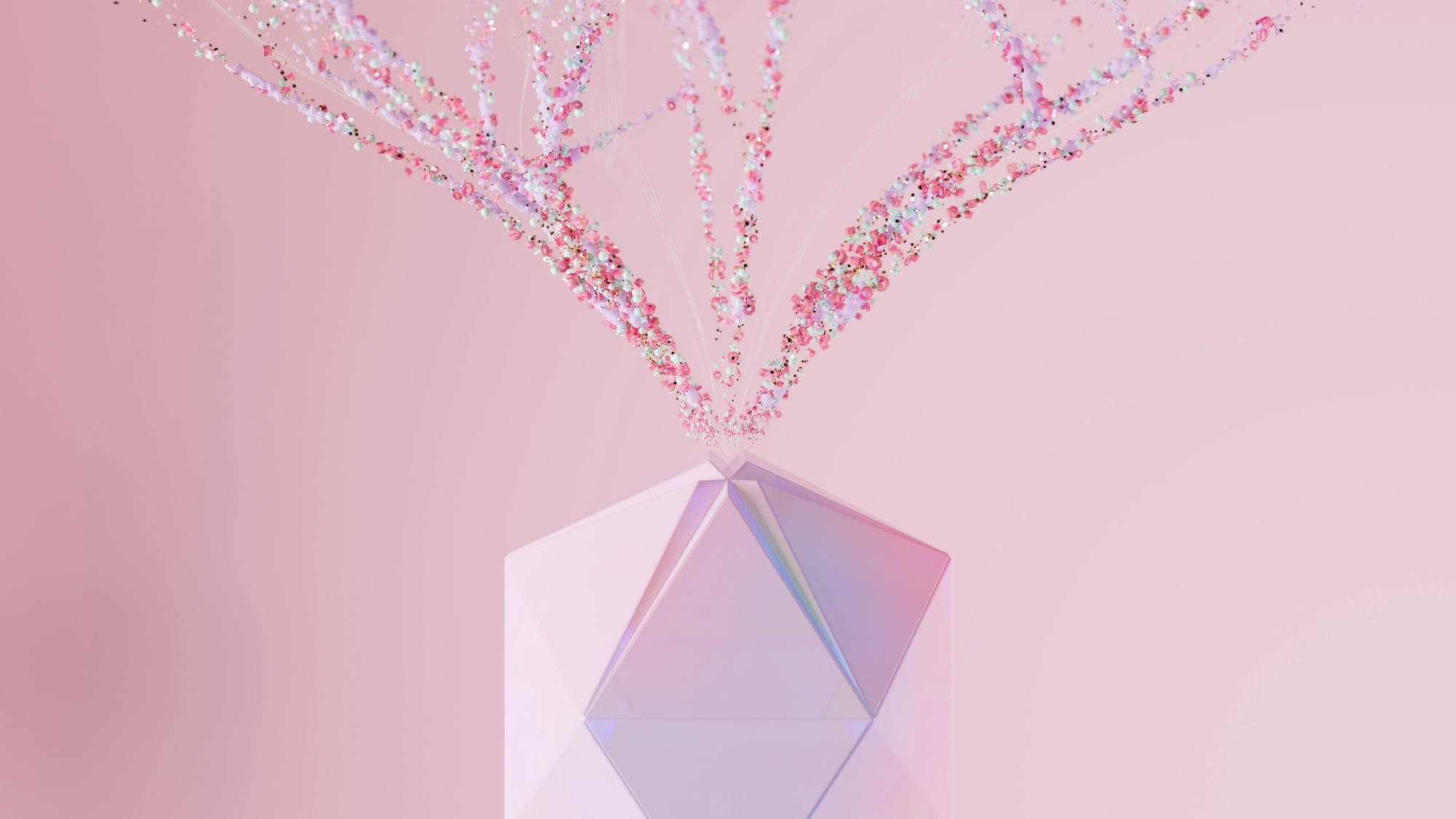
Generative AI is no longer a novelty. As companies figure out how to implement the technology to create real value, best-in-class retailers must move from testing to scaling or risk falling behind their competitors—or, worse, losing customers. We examine the steps and common pitfalls of navigating the challenges that new developments will pose to businesses and the keys to implementing a scalable AI framework.
Scalability AI: Initial Barriers
Despite its short life, generative AI has already allowed data and artificial intelligence experts to work on many cases, revealing the priceless value of this technology and the emergence of challenges to scale it.
A Major Hurdle in AI Adoption
Proper data management remains one of the main barriers to creating value in GenAI projects. In fact, according to a McKinsey survey, 70% of respondents have needed help integrating data into AI models, ranging from issues with data quality to defining processes for data governance to the availability of sufficient training data.
Many companies still need to fully understand how to develop the data capabilities to support generative AI cases at scale and how to use them to improve data practices.
3 Pillars to Drive Large-Scale AI
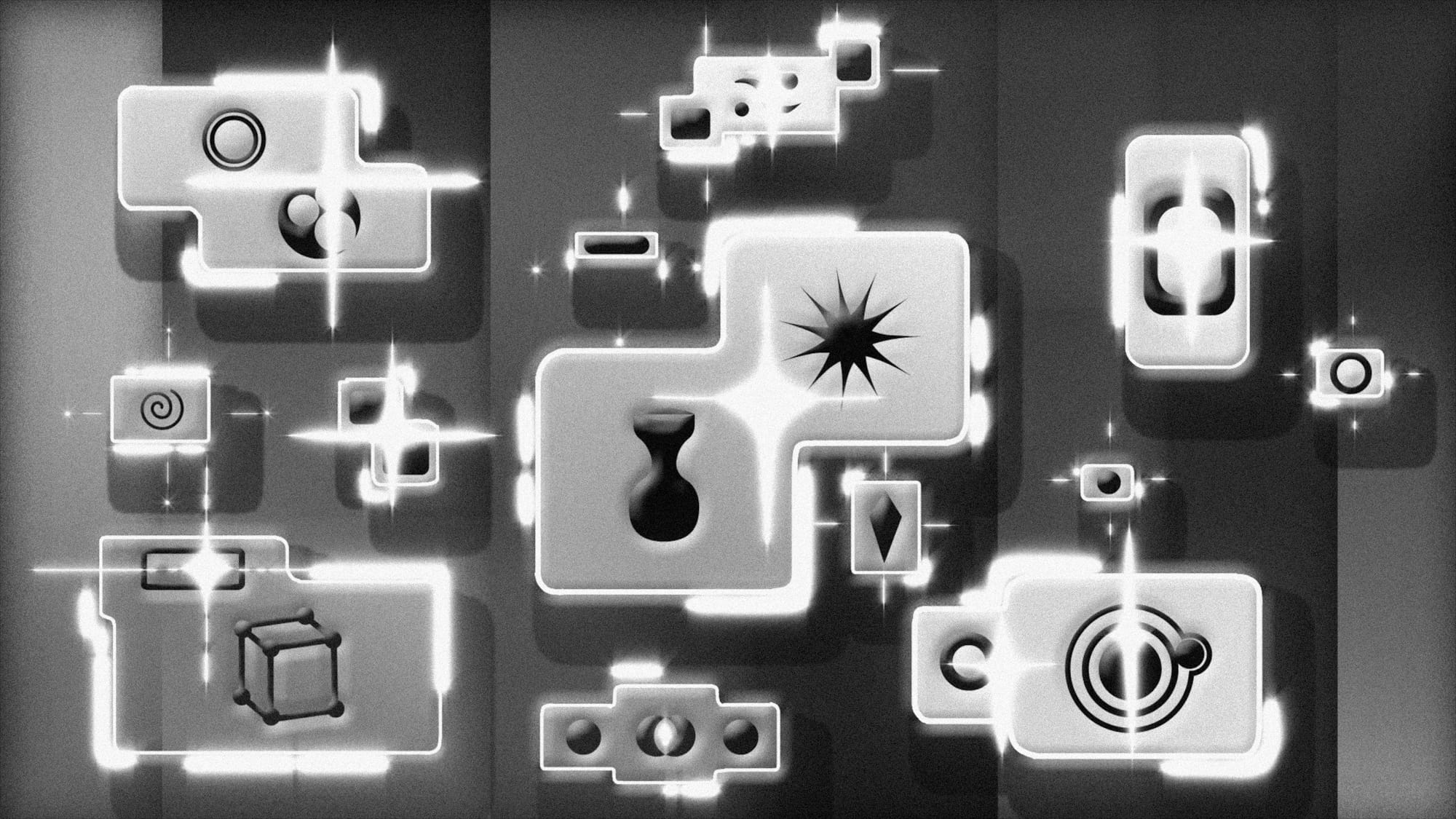
1. Community
The development of an AI-centric business within an organization is critical. Fostering a culture of collaboration and knowledge sharing is important. This fosters innovation and accelerates the organization's learning curve for AI applications.
2. Common Ground
Emphasis should be placed on building comprehensive and integrated AI platforms. This approach can provide a broader view of potential use cases and improve the combined utility of AI tools across different business functions.
3. Coordination
It is essential to identify and prioritize high-impact AI initiatives. Strategic alignment ensures that each company’s business objectives are clear and tangible results can be achieved.
How to Achieve Scalable AI
As mentioned above, the first and most crucial barrier companies face when implementing an AI project is getting good and sufficient data.Introducing poor data into GenAI models poses great risks, including:
- Poor results
- Costly solutions
- Cyber breaches
- Loss of user confidence
Therefore, inaccuracy of results is the biggest risk a company can encounter in using this technology.Traditional methods of ensuring data quality are insufficient, and new ways of improving and extending source data must be considered.
Getting Data from Better and More Reliable Sources
It is common to need help managing growing unstructured data sets. Combining it with structured data increases the possibility of errors, as it is more complicated to code it so that data processes can be easily replicated.
The Complexity of Data Integration
The good news is that tools have evolved to manage the relationship between different types and data sources. But even though data engineers understand the relationship between data sets, they must assign different methods to interpret that data based on various attributes, such as data format. This is a significant challenge as companies integrate formats into increasingly complex systems.
The Rise of Multimodal Models
Fortunately for businesses, multimodal models are now sophisticated enough to analyze more complex document types with disparate data formats, such as extracting tabular data from unstructured documents.
Ensuring Model Quality and Consistency
Accuracy issues require constant review, which is time-consuming if done manually. Therefore, automated evaluation methods, mechanisms to manage version control, and model consistency must be implemented.
Creating Necessary Data on Gen AI Use Cases
Some more advanced AI use cases are difficult to implement because the necessary data is difficult to obtain and process, which is often a problem in industries with very strict data security standards.To overcome this challenge, data engineers can manually generate a file to test the efficiency of a use case. Nevertheless, the process can be time-consuming and inefficient.
The Power of Synthetic Data
This is why data and AI leaders invest in AI tools to generate synthetic data as test data or to produce new values based on column descriptions and table context, allowing them to create new data or revise existing data.
Focusing on Data Governance and Data Security
The starting point for generative AI is to lay the foundations for trust in its design, its function, and how the results are used. Therefore, to achieve trusted AI, one must start with data governance and specific considerations around security.
GenAI-specific governance and usage policies help manage the potential risks of the technology’s capabilities embedded in enterprise resource planning, customer relationship management, and other business applications.To add greater security, look beyond the systems themselves. The new risks require evaluating the network architecture, security policies, data governance, and compliance framework.
In addition, a good option is to consider third-party risk management and continuous monitoring of how governance and risk practices interact with those of the enterprise. This will help to close gaps and achieve a much more secure environment.
Designing a Strategy to Speed Up Return on Investment
The best AI solution is the one that can scale. Therefore, creating patterns that can be applied across business processes is important. Unlike traditional AI, generative AI does not require a new model for each task, making it easy to quickly deploy the same GenAI model in many areas.Focusing on each business's core processes is the best way to achieve the greatest return on investment.
Implementing Use Cases that Create Value
The first PoCs should be aligned with each company’s strategy, with a focus on core business processes, organizational readiness, and repeatability.
Iterative Improvement of GenAI Models
When these early use cases are in place, data and AI professionals can study those with positive and notable results and thus create a working model in which the results of GenAI models can be refined and a mechanism to monitor and customize the internal workings of the model.
Outcompeting with Gen AI in Retail
To scale their gen AI tools, retail executives can consider five imperatives for outcompeting in digital and AI:
1. Identify Domain-Level Transformation Candidates
Retail executives should identify the domains where a transformation is needed, such as in customer experience, marketing, or store employee productivity, before identifying which gen AI use cases to pursue. By identifying transformations at the domain level first, retailers can determine which tools will bolster gen AI’s impact, such as:
- Robotic process automation (RPA)
- Advanced analytics
2. Upskill Talent to Develop Gen AI Skills
Retail people leaders should offer technical and nontechnical talent the opportunity to engage in learning programs, such as those focused on gen AI software development and prompt engineering.
3. Form a Centralized, Cross-Functional Team to Enable Scaling
While scaling gen AI will hinge on a retailer’s tech capabilities, retailers can gather leaders from across the organization to identify how gen AI can help improve the business. These cross-functional teams convened to help accelerate scaling in the short term should have shared goals that extend across the business and reinforce the retailer’s overall strategy.
4. Set Up Technology Architecture to Scale
Before committing to a specific gen AI vendor, retailers should experiment with different ones to assess which best suits their needs. The ideal gen AI architecture for retailers will be agile enough to make switching between LLMs easier, thus making scaling the technology across the organization easier. (This means using modular components that can be easily swapped out.)
5. Ensure Data Quality to Fuel Models
Unstructured data will be critical to powering retailers’ gen AI tools and providing key customer insights. Retailers should identify the unstructured data sources that differentiate them from other retailers (grocers, for example, could develop new recipe databases or leverage existing ones) and establish metadata tagging standards so tech teams can more efficiently power a retailer’s gen AI models.
Decisions on data should be backed by a clear understanding of the data’s business application.
The Unique Challenges and Rewards of Scaling Gen AI in Retail
Some of the guidance outlined above may be sector-agnostic, but scaling gen AI in retail is unique because several of the technology’s use cases involve direct consumer interactions. In retail, even a 1 percent margin of error could result in millions of customer-facing mistakes.
This emphasizes the importance of solid-gen AI risk guidelines and safety testing. The stakes may be higher, but the rewards are, too.
Related Reading
- How to Fine Tune LLM
- How to Build Your Own LLM
- LLM Function Calling
- LLM Prompting
- What LLM Does Copilot Use
- LLM Evaluation Metrics
- LLM Use Cases
- LLM Sentiment Analysis
- LLM Evaluation Framework
- LLM Benchmarks
- Best LLM for Coding
The 11 Best Generative AI Solutions for Retail
1. Lamatic – Your Managed Generative AI Tech Stack
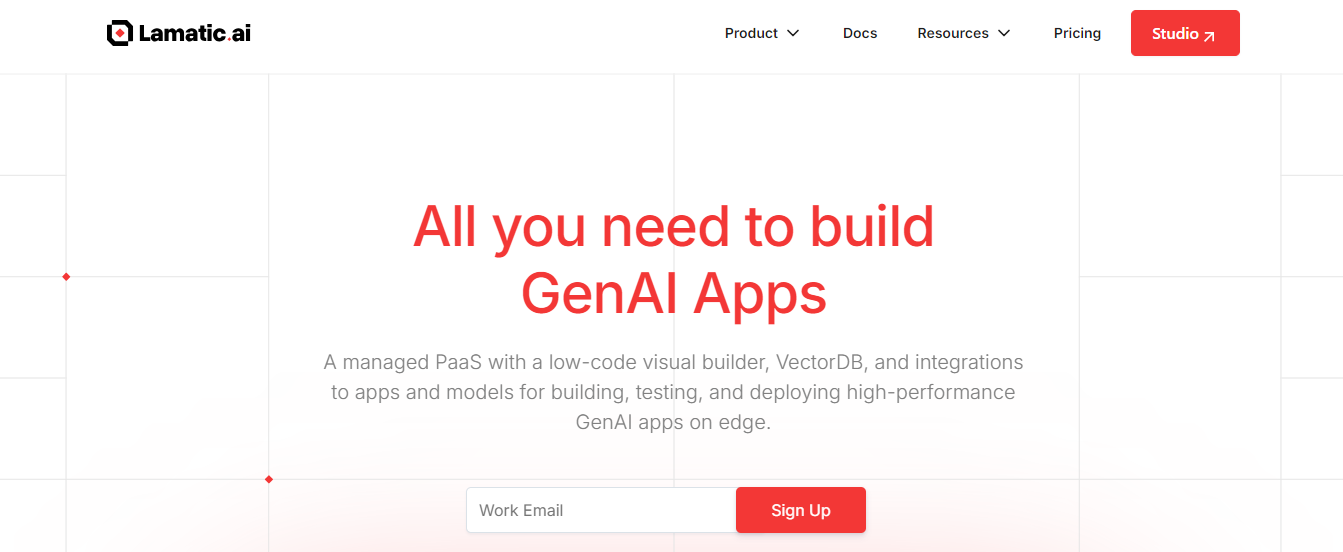
Lamatic offers an agile-managed generative AI tech stack designed to help teams:
- Rapidly and reliably implement AI solutions.
- Automate workflows for building, deploying, and managing AI apps.
Key benefits of the platform include:
- Avoid the accumulation of technical debt.
- Enabling production-grade deployment on edge via Cloudflare Workers.
Start building custom generative AI tech stack for free today!
2. ChatGPT (GPT 3.5) – The Free AI Tool for Writing and Research
OpenAI’s ChatGPT (based on the GPT 3.5 large language model) is an incredibly versatile AI tool. Released in November 2022, it shines at generating human-like text and can assist with a variety of tasks, including:
- Content generation
- Customer support
- Author brainstorming
- Much more
The model’s impressive capabilities in natural language processing, understanding, and generation make it especially suitable for a myriad of uses in retail.
3. GitHub Copilot – The AI Assistant for Coding
GitHub Copilot is arguably one of the best generative AI tools for coding. The model offers various collaborative features and integrations with popular code editors. As a code-focused LLM-driven solution, GitHub Copilot utilizes natural language processing technology to generate:
- Code snippets
- Context-based guidance
- Explanations
As such, the model can significantly enhance developer productivity and learning. This impressive versatility also makes it suitable for various beneficial use cases, including:
- Accelerating the coding process
- Facilitating learning
- Elevating code quality through effective defect detection
- Facilitating learning by helping programmers learn new programming concepts
On the downside, you may need to be vigilant when utilizing the model, as some generated code may need further polishing. It also heavily relies on external APIs for suggestions, which might complicate setup and utilization for inexperienced users.
4. Scribe – Your AI Writing Assistant
Scribe is an AI writing assistant specially designed to streamline content generation. It shows remarkable prowess in:
- Crafting reports
- Summarizing articles
- Aiding in academic writing
This has made it especially popular among students, journalists, and other professionals who use it for research and content generation.
5. ChatGPT Plus (GPT-4) – The Latest AI Model for Writing
GPT-4 is the latest addition to OpenAI’s GPT family. Unlike ChatGPT in its free version, GPT-4 has multimodal capabilities, which means it can process text and image inputs. Like its predecessors, GPT-4 has remarkable capabilities in content generation, making it an invaluable tool for:
- Marketers
- Writers
- Editors
It is important to note that GPT-4 was trained on a larger corpus of data than its predecessors, which means it can generate higher-quality content across various domains and handle more nuanced queries.
6. Cohere Generate – The AI Tool for Dynamic Conversations
Cohere Generate has proven effective in crafting dynamic dialogue systems that enhance user engagement. The model leverages NLP technology to generate personalized content, facilitating various use cases, from virtual agents to custom email generation.
7. AlphaCode – The AI Model for Coding
AlphaCode is a dynamic coding assistant that leverages gen AI to perform many coding tasks. The model excels in various use cases, including:
- Bug resolution
- Code generation
- Task automation
- Suggesting optimal programming solutions
Human Oversight Remains Crucial
Despite its efficiency in mitigating coding errors and fostering coding proficiency, you may need to perform further polishing in intricate tasks since the model relies on established programming patterns.
8. Gemini (previously Bard) – Google’s ChatGPT Alternative
Google Gemini (p. Bard) is a revolutionary chatbot and content-generation tool. Developed as Google’s answer to ChatGPT, Bard leverages LaMDA, a transformer-based model to generate natural, human-like content. Bard can draft several forms of written content, including:
- Blog posts
- Articles
- Creative writing pieces
It can also aid in code generation and provide helpful suggestions for programming-related queries.
9. Claude – The Conversational AI Assistant
Claude is a cutting-edge AI assistant developed by Anthropic, capable of:
- Handling extensive data processing tasks
- Automating complex workflows
- Engaging in natural, fluent conversations
10. DALL-E2 – The AI Image Generator
Dall-E2 is a state-of-the-art image generation tool. It can translate text into captivating visuals, enabling designers and artists to explore new realms of reality. The model can also accommodate various image styles and genres, allowing it to produce unique artwork and custom images.
11. Duet AI – The Writing Assistant for Google Apps
Duet AI is a cutting-edge writing assistant poised to revolutionize Google Workspace. The model leverages LLM capabilities to generate and summarize content and integrates seamlessly with popular Google applications.
AI-Powered Productivity
The model is still in beta but shows significant potential to streamline workflows within Google applications. According to the company, you’ll soon be able to:
- Generate content
- Summarize text
- Rewrite content within familiar tools like:
- Google Docs
- Gmail
- Google Meet
Start Building GenAI Apps for Free Today with Our Managed Generative AI Tech Stack
Lamatic offers a managed generative AI tech stack that helps teams build AI-powered applications and solutions without the hassle. Their platform automates workflows and provides production-grade deployment for generative AI applications so businesses can quickly implement AI capabilities to deploy applications in real-world environments.
Lamatics: Empowering Retail with AI Tools
Their robust middleware solution provides everything teams need to build, deploy, and manage AI applications, including:
- Integrated vector database
- Custom API for easy integrations
- Low-code agent and workflow builders
With Lamatic, the future of retail is bright, and teams can prepare for whatever comes next.