Creating AI systems can feel daunting. Developers face immense pressure to create software that performs complex tasks with few bugs quickly. The stakes are high: advancements in AI have far-reaching implications across every sector of the economy, and these innovations can impact the lives of millions of people. Unsurprisingly, there's fierce competition among businesses to develop the best AI systems and bring their products to market first. In this blog, we'll explore the role of AI developers in building more brilliant, more efficient AI systems that drive innovation and real-world impact. You'll learn how AI developers can achieve their goals faster with the help of multi agent AI. At Lamatic, we created a generative AI tech stack to help AI developers and their teams work more efficiently and productively. Our tools help teams streamline the development process, so they can focus on what matters: building robust AI systems that make a difference.
How are AI Tools Changing the Way Developers Work?
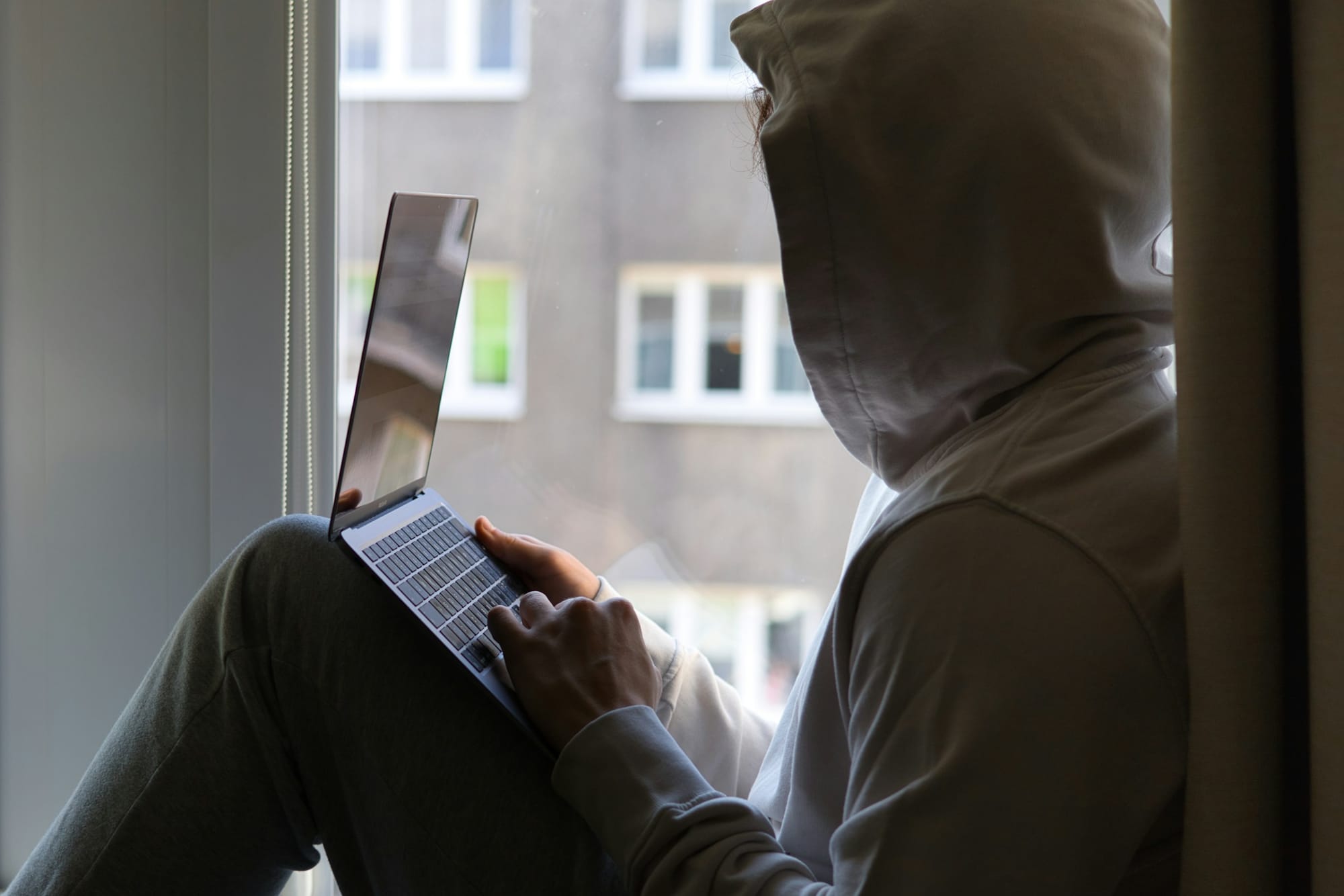
AI developers are at the forefront of modern software development, integrating artificial intelligence into applications while leveraging AI tools to enhance productivity and innovation. Their work goes beyond coding, they continuously optimize how AI models interact with other systems to ensure seamless performance and alignment with business goals.
For example, an AI developer working on an NLP chatbot for a financial services firm might analyze user logs to improve response accuracy. They refine prompt logic, adjust API calls, and integrate systems like retrieval-augmented generation (RAG) or sentiment analysis to enhance user satisfaction. Unlike machine learning engineers, who focus on model architecture and training, AI developers prioritize:
- Integration
- Deployment
- End-user performance
Cross-Functional Collaboration in AI Development
Collaboration is key: AI developers work closely with software engineers, data scientists, and product teams to test, monitor, and iterate on AI-driven features in production. They are essential to delivering intelligent, responsive, and efficient digital experiences.
AI Tools Are Automating Code Generation
AI is transforming software development by automating code generation and streamlining workflows. Developers have relied on libraries, repositories, and open-source projects to accelerate growth. Today, AI tools like GitHub Copilot take this further by analyzing prompts and existing code to generate snippets, functions, and complete modules with minimal human input.
This shift reduces development time and coding errors and allows engineers to focus on higher-level problem-solving. Copilot, powered by OpenAI’s Codex and trained on billions of lines of public code, can suggest:
- Real-time code completions
- Generate documentation
- Automate repetitive tasks such as refactoring
These capabilities significantly enhance productivity and support best coding practices across various programming languages.
AI Is Automating Repetitive Tasks
Software development involves a host of repetitive tasks. AI can help automate many tedious processes, freeing developers to focus on higher-level, creative tasks. For example, AI can automate code formatting, simplifying code neatness and organization. It can also:
- Create and run tests
- Generate documentation
- Assist with deployments
Parasoft and its suite of tools help automate the testing process to improve software quality and reduce time to market. By using AI to identify what needs to be tested and create test cases, the company helps eliminate manual processes and reduce the time spent on testing.
AI Tools Help Identify Bugs and Security Vulnerabilities
One of the most exciting applications of AI in software development is detecting bugs and security vulnerabilities. AI can analyze code and learn “normal” behavior, making finding anomalies that could indicate problematic code areas easier. For instance, if a piece of code is acting unusually and returning unexpected results, it may not function as intended and could be a candidate for further inspection.
If the code also operates under suspiciously low test coverage, it could represent a serious risk and even contain a security vulnerability. By identifying these issues early in the development process, AI tools can:
- Help reduce risks
- Improve software quality
- Accelerate time to market
AI Can Enhance Testing Processes
AI is revolutionizing software testing processes. Using machine learning, AI can:
- Automate test case creation and execution
- Analyze data from prior test runs to predict code failures
- Suggest improvements to existing tests
For example, startup Mabl has created a testing tool that uses AI to help developers and QA teams create and run automated tests. Mabl’s technology analyzes data from previous test runs to identify patterns and optimize testing processes, making it easier to catch bugs and improve software quality.
AI Improves Project Management
AI can also help improve software development teams’ project management. By analyzing historical project data, AI can predict upcoming tasks, assisting teams in understanding what to expect as they progress through a project. AI can also identify bottlenecks and make suggestions to improve team efficiency.
For example, AI can analyze code performance and suggest optimizations, enhancing software speed and efficiency. Informed decision-making. AI offers insights by analyzing data and helping developers make informed decisions about software design and architecture. AI tools for developers are empowering them to work:
- Smarter
- Faster
- More efficiently
Related Reading
- What is Agentic AI
- How to Integrate AI Into an App
- Generative AI Tech Stack
- Application Integration Framework
- Mobile App Development Frameworks
- How to Build an AI app
- How to Build an AI Agent
- Crewai vs Autogen
- Types of AI Agents
30+ Powerful Tools Helping AI Developers Solve Bigger Problems
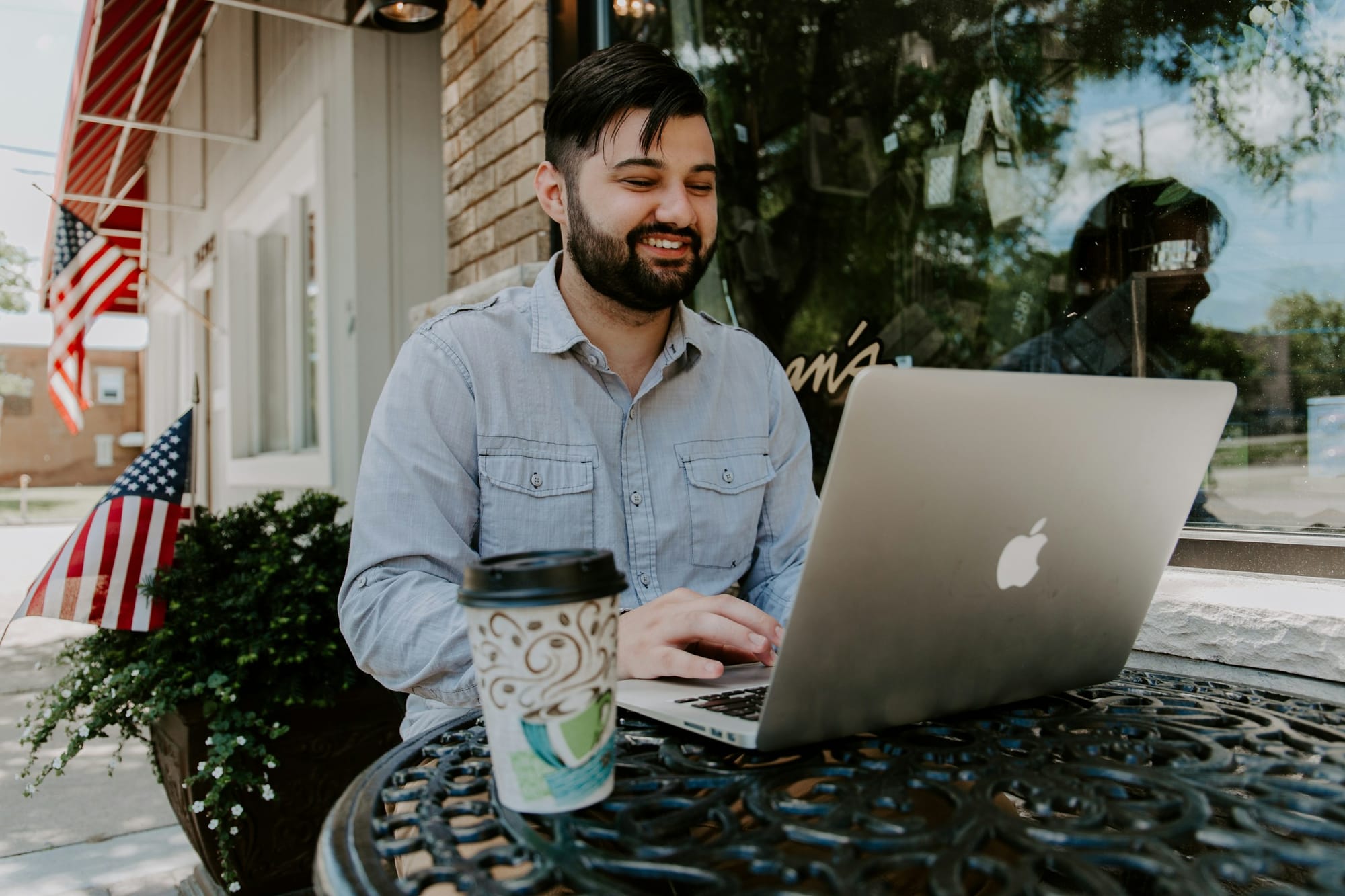
No-Code AI Tools for Developers
No-code AI tools help automate tedious tasks, allowing developers to focus on more complex problems. These tools also simplify AI integration for non-technical users.
Here’s a list of the best no-code AI tools for developers.
1. Lamatic
Lamatic offers a managed generative AI tech stack. Our solution provides:
- Managed GenAI Middleware
- Custom GenAI API (GraphQL)
- Low Code Agent Builder
- Automated GenAI Workflow (CI/CD)
- GenOps (DevOps for GenAI)
- Edge deployment via Cloudflare workers
- Integrated Vector Database (Weaviate)
Lamatic empowers teams to rapidly implement GenAI solutions without accruing tech debt. Our platform automates workflows and ensures production-grade deployment on the edge, enabling fast, efficient GenAI integration for products needing swift AI capabilities.
Start building GenAI apps for free today with our managed generative AI tech stack.
2. ConsoleX
When I need more control over prompts and outputs for complex tasks, ConsoleX offers precision that standard versions of ChatGPT don’t. It fine-tunes interactions and customizes outputs for various applications.
Here’s how I use ConsoleX:
- Customize coding and data analysis prompts: ConsoleX gives me more control to do complex coding tasks, debug issues, and run data analysis workflows more accurately.
- Multi-step workflow automation: I can create multi-step workflows where ConsoleX follows a sequence of commands to perform tasks like code generation, data extraction, and reporting.
- Custom output formatting: ConsoleX lets me tailor the format of its responses, which is useful when I need structured data or reports that fit into my existing processes.
- Advanced technical troubleshooting: I use ConsoleX to provide more nuanced and detailed responses for complex technical challenges, offering targeted solutions and actionable insights.
3. ChatArena.ai
ChatArena.ai lets me compare outputs from multiple large language models (LLMs) in one interface, such as:
- ChatGPT
- Claud
- Llama
This feature is valuable when evaluating how different models handle the same tasks or prompts.
Here’s how I use ChatArena.ai:
- Compare LLM outputs: I test multiple LLMs on the same prompt to assess their strengths and weaknesses, particularly for projects that require precise language understanding or creative problem-solving.
- Evaluate code generation: Different models often produce varying results when generating or debugging code. ChatArena.ai compares how each LLM handles the same coding query so I can choose the most accurate output.
- Refine prompt engineering: Since models interpret prompts differently, I refine my prompts using ChatArena.ai to ensure the best outcome.
4. Octoparse
Scraping data from websites can be time-consuming, especially with large datasets. Octoparse simplifies this process with a no-code platform that automates web scraping so that I can collect data quickly without coding skills.
Here’s how I use Octoparse in my workflow:
- Automated web scraping: I can extract data from multiple websites, such as competitor analysis, product listings, or keyword data, in a fraction of the time it would take manually.
- Data structuring and export: After scraping the data, I organize it into structured formats like Excel or CSV for further analysis.
- Use cases with external AI tools: I often export the scraped data and feed it into tools like ChatGPT for tasks like summarizing or generating content ideas.
- Lead generation and link building: Octoparse scrapes directories and forums for potential leads or link-building opportunities, making outreach more efficient.
AI APIs Use Cases
AI APIs enable developers to integrate existing AI functions into their projects and workflows. With AI APIs, developers can save time and resources while building applications with intelligent capabilities.
Here are some of the best AI APIs for developers.
5. Replicate
When I need to integrate AI models into my workflows quickly and without hassle, Replicate is my go-to. I can run any AI model as an API and integrate different models into my existing systems without technical overhead.
Here’s how I use Replicate:
- Run AI models as APIs: Deploy AI models instantly as APIs, eliminating time-consuming setup.
- Content generation: Integrate content-generation models into workflows for faster, scalable AI content creation.
- Model versioning: Test different model versions and revert to previous versions if needed.
- Collaborate with teams: Share and access models across teams, enabling easier collaboration on AI projects. Specialized applications: Implement AI models for image recognition, data analysis, and other specialized tasks with minimal effort.
6. OpenAI’s API for Speech-to-Text (Whisper)
Transcribing audio or video content can be challenging, especially when dealing with poor audio quality or multiple speakers. I use OpenAI’s Whisper to generate accurate transcripts from complex audio or video files.
Here’s how I use Whisper:
- Transcribe webinars and meetings: I convert spoken content from webinars and meetings into detailed transcripts for future analysis.
- Turn video content into text: Whisper makes it easy to transcribe and repurpose video content into blog posts, articles, or other formats.
- Handle complex audio: Whisper’s accuracy with poor-quality audio or multiple speakers ensures I never miss important details, making it a reliable tool for all my transcription needs.
7. Page Type Detection With GPT-4V
Manually categorizing web pages can be time-consuming when dealing with large volumes. I use GPT-4V (GPT-4 with vision) to analyze web pages visually, making it easier to detect and categorize different page types automatically.
Here’s how I use GPT-4V:
- Automated page type detection: Upload screenshots and have the AI classify each page as a product page, blog post, home page, etc.
- Content organization: GPT-4V organizes content by identifying page types based on visual input.
Build With AI
Developers can also use AI tools to accelerate the building process.
Here are some of the best AI tools for building applications.
8. Galileo: From Text/Image to User Interface (UI) Design
Galileo transforms text descriptions or images into user interface (UI) designs, making it possible to generate high-quality mobile and desktop mockups. Whether describing an app idea or uploading an image, Galileo quickly produces design mockups I can export to platforms like Figma for further refinement.
Here’s how I use Galileo:
- Rapid UI prototyping: I describe a design idea or upload an image, and Galileo generates a UI prototype for apps or websites.
- Export designs: I export mockups to Figma for further refinement, streamlining my design workflow.
- Customize designs: Galileo adjusts UI elements, offering flexibility to customize designs for usability and aesthetics.
9. Bubble: No-Code App Builder for AI Integration
Bubble is a no-code platform I use to build functional web apps quickly. I describe the type of app I want, and Bubble generates the core structure. The drag-and-drop interface lets me customize everything from design to AI integrations.
Here’s how I use Bubble:
- Build AI-powered apps: I create AI apps that automate content creation and SEO workflows.
- Drag-and-drop customization: I use the platform to design and build apps without technical skills.
- Integrate AI tools: Bubble supports integrating AI models, making it easy to add AI functionality to custom-built apps easy.
10. Streamlit: Turn AI Models Into Web Apps With Ease
When I need to turn an AI model into a functional web app, I rely on Streamlit to create fully operational applications without worrying about complex infrastructure or front-end development.
Here’s how I use Streamlit:
- Create interactive SEO tools: I can transform an AI model into an interactive SEO tool that clients or my teams can use.
- Share data insights: Streamlit makes it easy to share data insights in an interactive and accessible web app format.
- Simplify app development: I upload my code, and Streamlit handles the entire web app infrastructure, removing the need for complex development.
11. Chainlit: Build Conversational AI Apps
Creating conversational AI applications can be daunting, but Chainlit simplifies the process. Whether I need to build a chatbot or automate internal workflows, Chainlit connects large language models to user-friendly interfaces.
Here’s how I use Chainlit:
- Build chatbots: I develop chatbots that respond to client inquiries or provide real-time insights.
- Query data from Google Analytics: I use Chainlit to interact with AI models to extract and analyze data from platforms like Google Analytics.
Setting Up Large Language Models (LLMs) Locally
Developers can run AI models locally, so they don’t have to rely on the cloud for every interaction. This setup allows for more control and privacy, especially when handling sensitive data.
Here are some tools that help set up LLMs locally.
12. Llama 3.2: Run AI Models Locally
Relying on cloud services for AI tasks can be frustrating due to usage costs, internet dependency, and privacy concerns. I needed a solution that offered robust AI capabilities without these limitations.
With Ollama, I run large language models like Llama 3.2 on my machine to access advanced AI features without relying on cloud services or incurring ongoing costs.
Here’s how I use Llama 3.2:
- Generate code: I use Llama 3.2 to write and debug code without connecting to the cloud.
- Create content: Llama 3.2 helps me generate content across various formats while maintaining complete privacy and control.
- Produce embeddings: I generate word and sentence embeddings for SEO tasks like keyword mapping, all locally.
- Integrate with tools: With Ollama’s local API, I can integrate Llama 3.2 into custom tools and workflows without relying on third-party services.
13. Open WebUI: User-Friendly Interface for Local AI Models
Running AI models locally is challenging if you’re uncomfortable with the command line or code. I wanted a solution that made this process more accessible for those who prefer graphical interfaces over text-based commands.
Open WebUI is an open-source interface that builds upon Ollama. I use it to run open-source AI models like Llama 3 on my machine. This allows me to perform tasks I typically do in ChatGPT locally without complex setups.
Here’s how I use Open WebUI:
- Write App Script code: I prompted Open WebUI to generate code that produces embeddings from OpenAI and inserts them into Google Sheets.
- Copy-paste code into Google Sheets: I generated the code, pasted it into Google Sheets, and ran it as a function, allowing for seamless integration into my workflow.
- Accessible for non-coders: This tool makes advanced AI capabilities available to those who prefer using graphical interfaces instead of working with the command line.
14. LM Studio: A Seamless Interface for Local AI Models
With LM Studio, I can easily download and run models like LLaMA 3.2 and interact with them through an intuitive interface. There’s no need for complex setups or coding skills: just download the model, and you’re ready to go.
Here’s how I use LM Studio:
- Generate content: LM Studio generates AI content for blogs, social posts, and more without needing cloud-based services.
- Produce embeddings for clustering and classification: Use embeddings to group similar texts or classify content based on semantic similarity, improving content organization and analysis.
15. GPT4all: Accessible Local AI Models
With GPT4All, I can perform tasks similar to ChatGPT without relying on cloud services or worrying about data privacy.
The setup was smooth:
- Install the application
- Download my model
No advanced technical skills are required.
Here’s how I use GPT4all:
- Perform AI tasks offline: From generating content to running embeddings, GPT4All offers the same functionality I’d get with ChatGPT but with local control.
- Document analysis: Using the RAG (Retrieval-Augmented Generation) feature, I upload local documents and quickly query them for insights.
16. Msty: Combine Local and Cloud AI Models
While local AI models offer control and privacy, sometimes I need the advanced capabilities of cloud services like ChatGPT. Msty combines open-source models running on my machine with cloud-based models for more flexibility and performance.
With Msty, I pick the best model for each task: handling privacy-sensitive jobs locally and switching to cloud models for more complex tasks. This hybrid approach optimizes my workflow, blending cloud services’ power with local models’ control and efficiency.
Here’s how I use Msty:
- Run hybrid AI workflows: I use local models for privacy-sensitive tasks and cloud models for more computationally demanding tasks.
- Easily switch between environments: Msty lets me switch between environments, offering a dynamic AI approach without added technical complexity.
AI Coding Tools
AI coding tools can help developers streamline programming tasks.
Here are some of the best AI coding assistants that help automate routine tasks and improve productivity.
17. Colab: Streamline Coding With AI Models
When I need to run AI models or write code without setting up complex environments, I turn to Colab. This Google tool runs Python code in the cloud, making it perfect for tasks like Natural Language Processing (NLP) or data analysis. It’s easy to use and even integrates with AI models like Gemini. As Britney Muller demonstrated, Colab simplifies the entire process.
Here’s how I use Colab:
- Run Python code in the cloud: I can run natural language processing models and data analysis scripts in Colab without worrying about local setups.
- Debug code with AI assistance: When errors arise, I paste them into the tool, and the AI offers debugging support.
- Process large datasets: For keyword research and topic modeling, I use Colab to process CSV files of keywords with AI SEO tools like BERT topic modeling.
- Integrate with AI models: Colab supports integrations with AI models like Gemini, giving me the power to run advanced machine learning tasks seamlessly in the cloud.
18. Gemini + Colab Integration: AI Code Execution
One of the most efficient ways I’ve improved my workflows is by combining Gemini with Colab. I can tap into Gemini’s language model directly within the Colab environment, making coding and AI tasks faster and more intuitive.
Here’s how I use Gemini with Colab:
- Run Python code with advanced AI assistance: I use Gemini’s AI capabilities to execute complex data processing and natural language tasks in Colab.
- Keyword clustering: Gemini helps me organize large sets of SEO keywords into clusters based on intent or topics.
- Content generation: I use the integration to generate content ideas and automate parts of the content creation process using Python scripts in Colab.
19. Programming Helper: AI Coding Assistance
Programming Helper offers AI support for writing and debugging code, making it essential for:
- Website optimization
- Integrating APIs
- Creating automation
It covers multiple programming languages and provides solutions where ChatGPT may not suffice.
Here’s how I use Programming Helper:
- Code generation: I describe what I need in plain language, and Programming Helper generates code in various languages. This helps with data analysis scripts, SEO automation, or web scraping.
- Learning support: When I face unfamiliar programming challenges, the tool offers code examples and explanations, making new concepts easier to grasp.
- Debugging: Programming Helper identifies issues and suggests fixes, streamlining the debugging process and improving code functionality.
20. Llama Index: Build RAG Systems
Finding the most relevant information quickly becomes challenging when handling vast amounts of content. Traditional search methods often fail to deliver context-rich responses, especially for SEO tasks that require precise accuracy.
Llama Index creates RAG systems by indexing extensive document collections, allowing AI to find the most relevant text based on a query. While it doesn’t support creating an index from sitemaps, I developed custom code to add this feature, making it more effective for content retrieval.
Here’s how I use Llama Index:
- Generate accurate content: Llama Index retrieves precise information to automate complex content tasks.
- Optimize content strategy: I use Llama Index to dig into large datasets and uncover critical insights that improve my content strategies.
21. LangChain: Build AI Agents
LangChain is my go-to framework for building AI agents that can perform tasks based on real-time data. It enables me to integrate language models into workflows and automate processes beyond basic tasks.
Here’s how I use LangChain:
- Connect to real-time data sources: I connect LangChain to tools like Google Analytics and Search Console to retrieve and analyze real-time data.
- Automate technical SEO tasks: I can write code for LangChain to automate actions like keyword tracking, meta tag analysis, and crawling for SEO issues.
- Generate content based on live data: I create agents that generate content based on current trends or data pulled from my connected sources.
- Build custom AI workflows: I use LangChain to design workflows that integrate AI models with any application, enabling more versatile and complex automation.
22. LangFuse: Manage and Observe AI Prompts
When working with multiple AI models and complex workflows, tracking how prompts perform and understanding the inner workings of each interaction is crucial. Without visibility into prompt usage, I risk inefficiencies and missed opportunities for optimization.
LangFuse solves this by providing a comprehensive view of AI prompts, showing how they perform, when they’re used, and where adjustments are needed.
Here’s how I use LangFuse:
- Track prompt performance: I monitor how each prompt performs across different workflows to identify which generates the best outcomes.
- Optimize prompt management: I tweak prompts to increase efficiency and improve workflow performance by observing usage patterns.
- Manage complex workflows: LangFuse helps me oversee AI interactions in workflows involving multiple models, ensuring that everything runs smoothly and effectively.
- Improve AI output quality: With real-time insights into how prompts are used, I can make data-led adjustments that improve the quality of AI content.
23. Regexer: AI Regex Generation
Writing regular expressions (regex) can be challenging, especially if you’re unfamiliar with syntax, or, like me, you’d rather not write regex. Regexer solves this by generating regex patterns from natural language descriptions.
Here’s how I use Regexer:
- Generate regex patterns: Instead of coding complex regex manually, I describe the task, and Regexer creates the pattern, saving time when handling large datasets or complex URLs.
- Filter data: I use Regexer to filter data in tools like Screaming Frog or Google Analytics, helping me focus on specific content or areas of a website.
- Create custom redirects: Regexer generates patterns for setting up redirects on large sites, especially when cleaning up outdated URL structures.
- Extract data from logs: Regexer extracts key insights from server logs by generating patterns to match specific details, improving log analysis for site optimization.
24. Literal AI: LLM Monitoring and Evaluation for Product Teams
AI development often requires robust monitoring and evaluation to ensure reliable outputs, which can be challenging when deploying LLM applications at scale. Literal AI addresses this by offering an end-to-end platform for observability, evaluation, and prompt management tailored for developers and product teams.
Here’s how I use Literal AI:
- Prompt testing and debugging: Literal AI’s prompt playground lets me create, test, and refine prompts in real time. I can easily adjust prompts to improve accuracy and output quality with in-context debugging and session visualization.
- Performance monitoring: I track essential metrics like latency and token usage, and set up alerts to notify me if I exceed performance thresholds.
- Comprehensive evaluation: Literal AI supports offline and online assessment, A/B testing, and RAG workflows. This variety of options helps me assess model accuracy and efficiency under different conditions.
- LLM observability: With multimodal logging, Literal AI captures LLM behavior across text, image, and audio inputs, giving me insights that inform adjustments and improve my models for better performance.
IDE (Integrated Development Environment) Tools for AI
One of the most essential tools for AI development is an IDE. An integrated development environment is a software application that provides comprehensive features to help developers write and debug code. Some of the best IDEs for AI development include:
- Visual Studio Code
- PyCharm
- Eclipse
25. Visual Studio Code
This lightweight source code editor works on desktops via the Mac, Windows, or Linux platforms. It boasts a rich pool of libraries and extensions you can use for development. VS Code has built-in support for:
- Node.js
- TypeScript
- JavaScript
VS Code Tools for AI is a cross-platform extension for VS Code. It allows developers to use deep-learning frameworks like TensorFlow and CNTK.
26. PyCharm
PyCharm is an IDE dedicated to Python development. As such, it provides a convenient environment and essential tools for “snake charmers.” It’s a popular IDE in artificial intelligence because Python is used for data science and machine learning.
27. Eclipse
Another popular AI-friendly IDE is Eclipse. It offers an appealing development environment for Java coders. Java is also an excellent programming language that is powerful enough to build AI-based apps. Like VS Code and PyCharm, Eclipse hosts tools and extensions for AI development.
Machine Learning Tools and AI Frameworks
Another essential tool for AI software development is a deep-learning platform. These software applications help developers build and train machine learning models. Some of the best machine learning platforms include:
28. TensorFlow
Google’s TensorFlow is a free open-source library popular among AI developers. It has many features, but mainly focuses on training deep neural networks. While TensorFlow uses Python, it requires C++ to boost performance.
29. Keras
Like TensorFlow, Keras is a free open-source library used for AI development. At its core, it’s an API (application programming interface) that provides backend support for TensorFlow. World-famous websites use Keras, like:
- Yelp
- Netflix
- Uber
30. Scikit-learn
This is another free Python-based library for coding artificial intelligence software. Although the lack of uppercase letters makes it hard to write about scikit-learn, this library supplies algorithms that help software perform:
- Data classification
- Regression
- Clustering
31. PyTorch
PyTorch is yet another machine learning framework based on Python and the Torch library. Its main goal is to speed up the prototyping process in deep-learning research.
Learning Resources and Communities
It’s essential to have a complete understanding of data structures and algorithms. Structures organize data in ways that make it easy to retrieve and manipulate, while algorithms are sets of instructions that solve problems.
Some of the best resources for real intelligence on AI data structures and algorithms include:
- Coursera
- DataCampC
- Udacity
Each platform offers a variety of materials that can help you understand artificial intelligence concepts better. Software developers also flock to different communities for AI development assistance. These are some of the top software developer communities on the interwebs today:
- GitHub
- Stack Overflow
- Hackernews
- Hackernoon
- Women Who Code
Related Reading
- Llamaindex vs Langchain
- LLM Agents
- LangChain vs LangSmith
- Langsmith Alternatives
- LangChain vs RAG
- Crewai vs Langchain
- AutoGPT vs AutoGen
- GPT vs LLM
- AI Development Tools
- Rapid Application Development Tools
Start Building GenAI Apps for Free Today with Our Managed Generative AI Tech Stack
Lamatic provides a managed generative AI tech stack that helps developers build intelligent applications. Focusing on automation, Lamatic equips teams with the tools they need to implement GenAI solutions quickly while avoiding technical debt.
The platform's automated workflows and production-grade features ensure that generative AI applications integrate smoothly into existing systems and function reliably in the real world.
Related Reading
- Best No Code App Builders
- LLM vs Generative AI
- Langchain Alternatives
- Autogen vs Langchain
- Langflow vs Flowise
- SLM vs LLM
- Langgraph vs Langchain
- Haystack vs Langchain
- Semantic Kernel vs Langchain
- UiPath Competitors
- Agentic Definition
- Best AI Models
- Best AI Coding Assistant
- LangChain Agent
- Best AI Code Generator
- AI Developer Tools